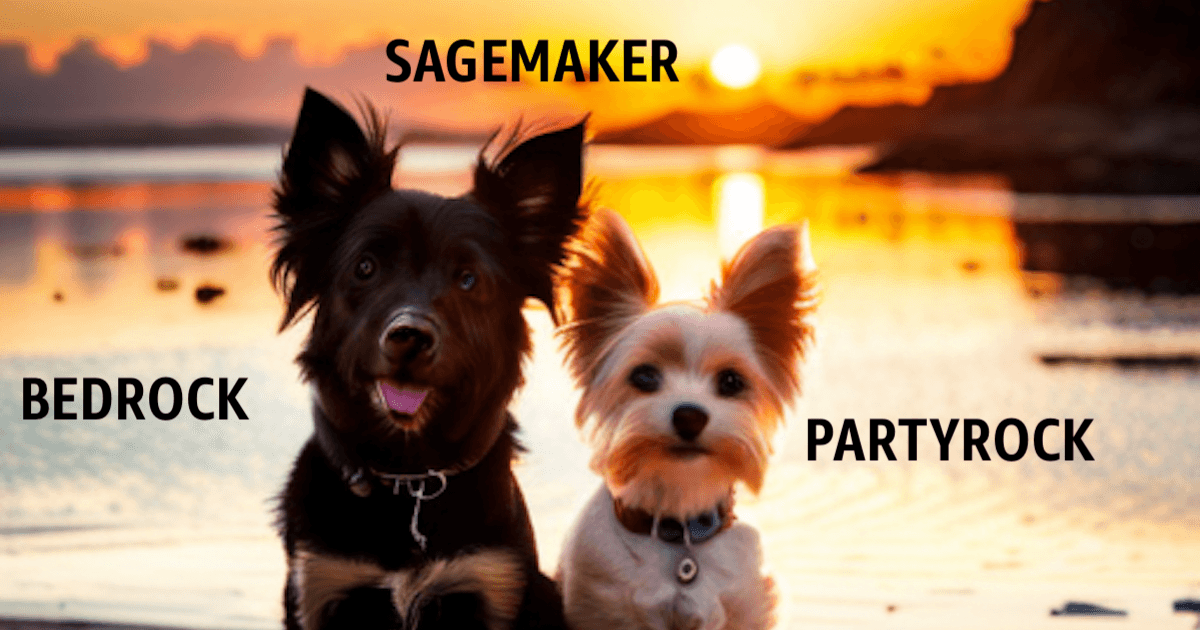
Bedrock, PartyRock, SageMaker: Choosing the right service for your Generative AI applications
Understand the capabilities and differences of Amazon Bedrock, PartyRock and Amazon SageMaker to decide what to use for your generative AI use-cases
Karan Desai
Amazon Employee
Published Dec 9, 2023
Last Modified Feb 1, 2024
Generative AI is the hottest topic in tech currently in 2023, and there are many different services available to build Generative AI projects and apps. At AWS, you might have come across three different services related to Generative AI namely- Amazon Bedrock, PartyRock, and Amazon SageMaker. If you are wondering what is the difference between them and when should you use which one, in this blog post I will help you understand each of them so that you can pick the right service for your use-cases.
If you are just getting started on your generative AI journey, this can be your first stop. PartyRock is a fun and intuitive hands-on, generative AI app-building playground. You don’t need to write any code, nor need any prior knowledge of machine learning to start creating your own apps with PartyRock. As you build your app using PartyRock, you will learn about the concepts and capabilities of generative AI such as various foundation models, interacting with the models by providing the right prompts and improving them iteratively - this is known as prompt engineering and is a skill set that will be very in-demand as we go into building more and more with generative AI.
Let’s build with PartyRock! Let's say I want generative AI to create unique children’s bedtime stories every day about the adventures of two dogs. We will use this example throughout this blog post.
Start by going to https://partyrock.aws/ and describe in normal English what do you want your app to do. For example, this is my requirement-
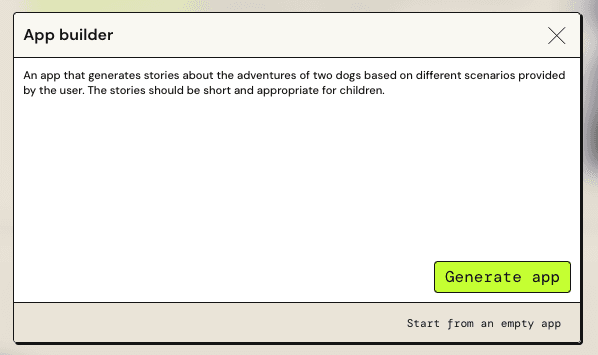
Once you click Generate app, PartyRock will do all the heavy lifting in the backend and create a web-based app where you can input your prompts and it will generate the desired content as the output. Here is what it created for me-
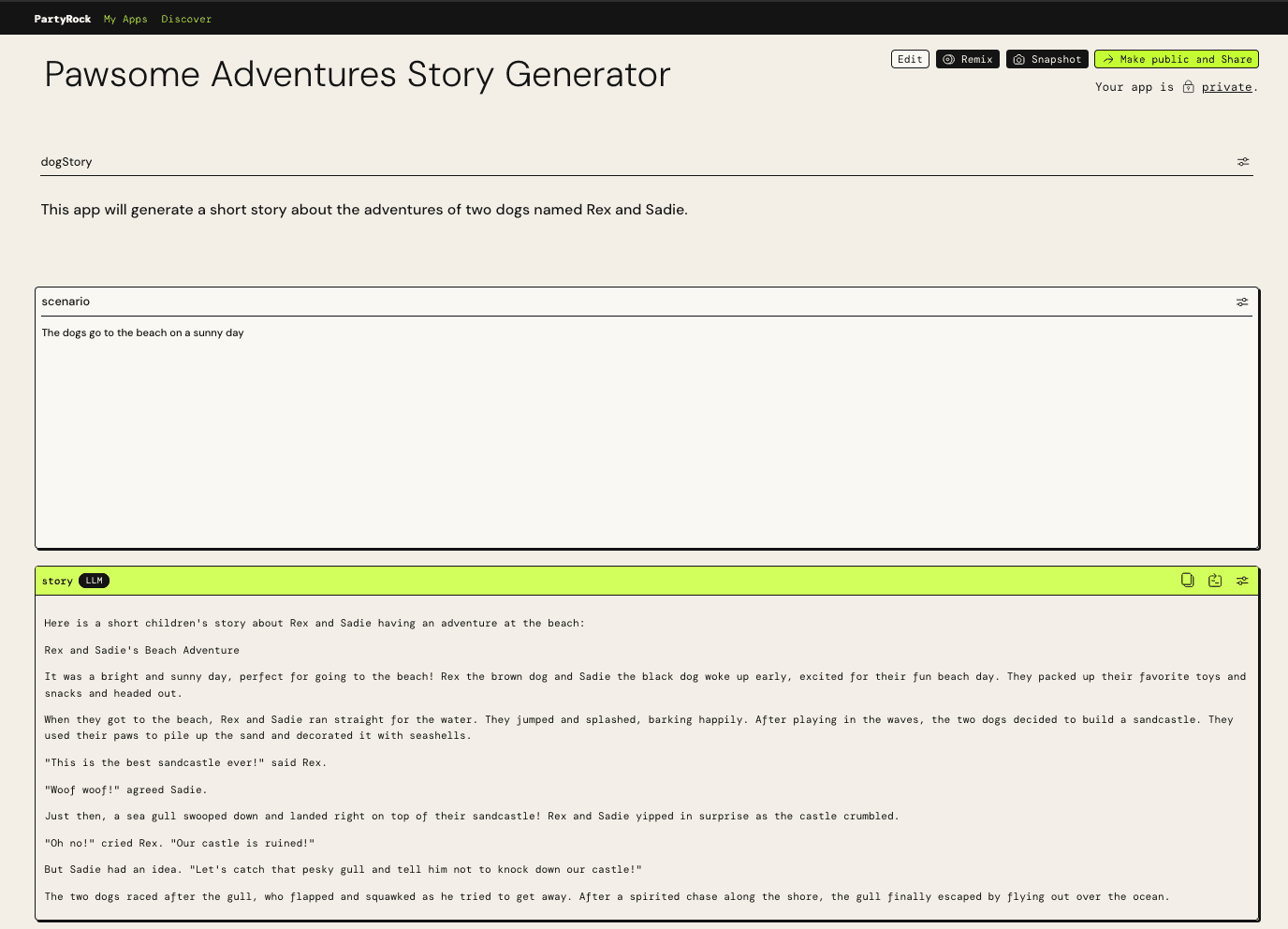
You can click on the little button on the right side of the story panel to get into the settings where you can further fine-tune your app if you wish to. Here you can see what large language model (LLM) has been used by PartyRock for your app, and what is the input prompt being fed to the model. You can change these to experiment more. In Advanced Settings you can also play around with changing values of two parameters called Temperature and Top P which determines the randomness of the LLM response and can result in more creative and imaginative text.
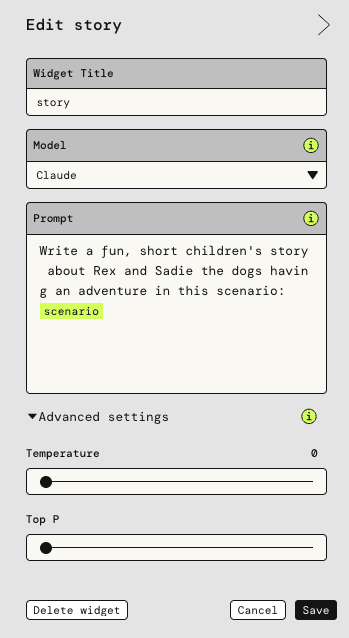
Your app is private by default, but if you are happy with what you have created and want to share it with the world, you can make it public. You can check out my Pawsome Adventures Story Generator.
If you want to learn more, you can also refer to Jeff Barr’s blog post on Building AI apps with PartyRock
When you feel you have learned the basics of generative AI with PartyRock and want to dive deeper into building generative AI projects, you can consider Amazon Bedrock.
Amazon Bedrock is a fully managed service that offers a choice of high-performing foundation models (FMs) from leading AI companies like AI21 Labs, Anthropic, Cohere, Meta, Stability AI, and Amazon via APIs. (Fun fact: PartyRock also uses Amazon Bedrock in the background, in case you were wondering)
With Amazon Bedrock, you can privately fine-tune FMs using your own labeled datasets. With fine-tuning and continued pre-training, Amazon Bedrock makes a separate copy of the base FM that is accessible only by you, and your data is not used to train the original base models.
If you want to build generative AI applications that use your organization’s proprietary information which the base foundation models are not aware of, Amazon Bedrock offers Knowledge Bases- a fully managed Retrieval Augmented Generation (RAG) capability to customize FM responses with your relevant private data.
Let’s repeat our earlier example, this time with Bedrock- once again we will create unique stories about our two doggie friends. To get started, go to Amazon Bedrock on the AWS console (you will need an AWS account for this. You can use your existing account, or create a new one).
In the Bedrock console, from the left panel you can go to Playground and select Text. This will give you an option to choose a model from several different providers. For my example, I choose Anthropic Claude 2.
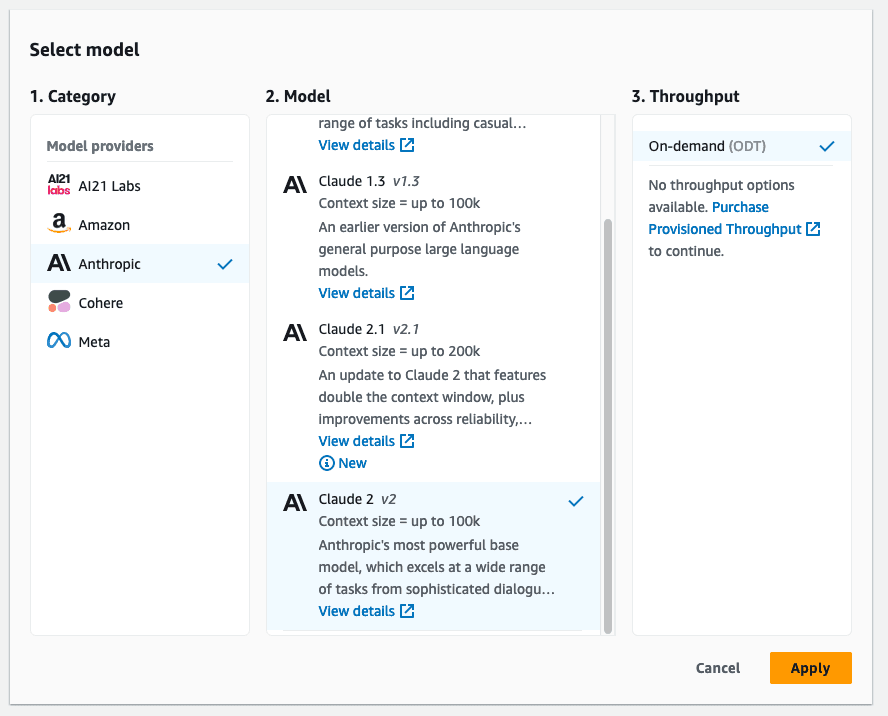
Once in the text playground, you can provide your prompt and run it to see what response the model generates. I give the same prompt that I gave earlier to PartyRock, and this is the story generated by the Claude 2 model running on Amazon Bedrock-
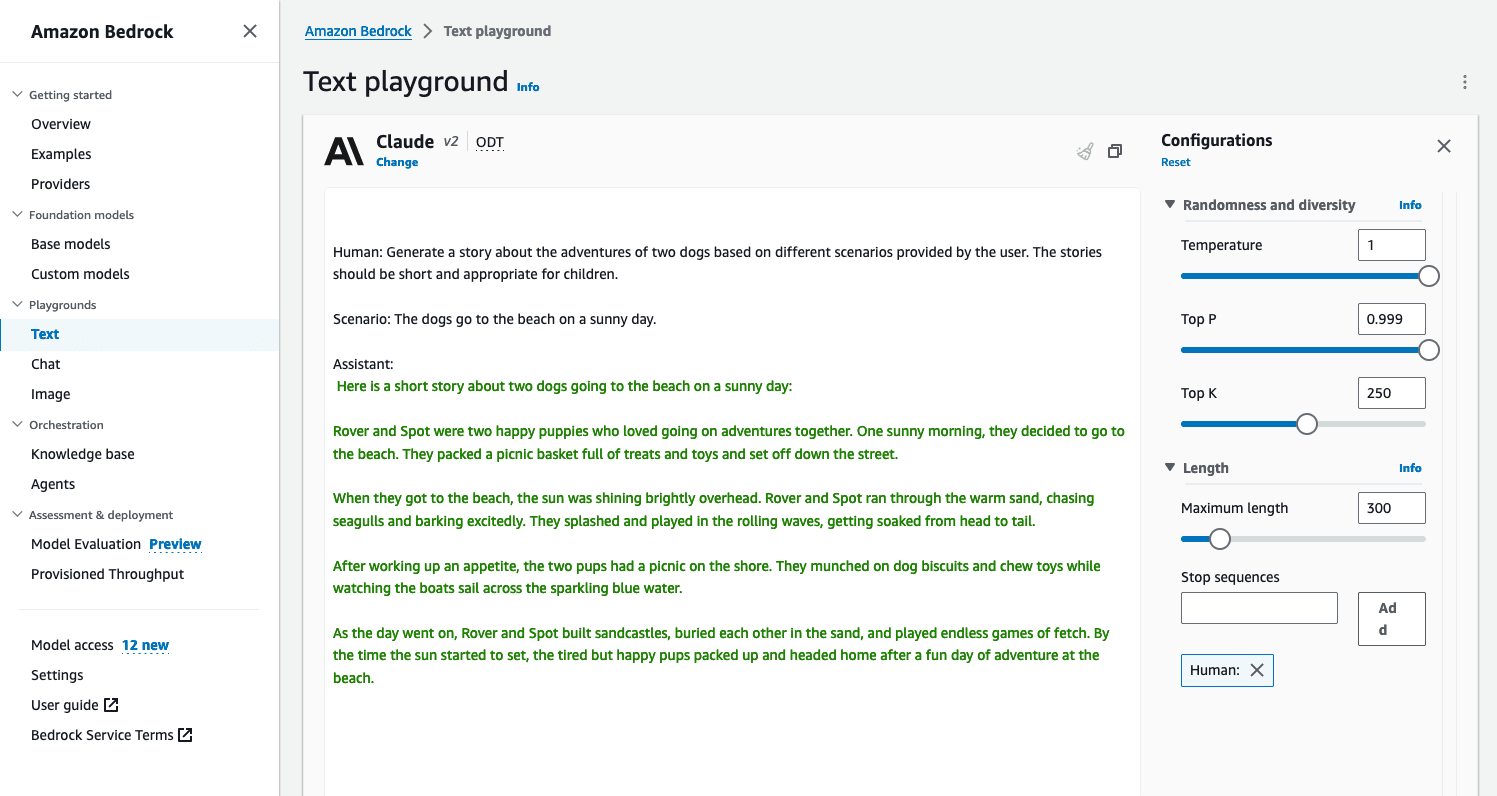
At this point, if you are wondering this is enough of playing around on the web, what about writing some code and start building, I got you covered! You can go to the Examples section in the left panel on the Bedrock console where you will find sample API requests for the various models available on Amazon Bedrock. For the above example, the API request would look like this-
You can make API calls to Amazon Bedrock through AWS SDKs for C++, Go, Java, JavaScript, .NET, Python (Boto3), and Ruby. Check out the User Guide to setup Amazon Bedrock API
If you want to get more hands-on experience, I recommend trying out the Building with Amazon Bedrock and LangChain workshop.
Amazon Bedrock is a great way to build generative AI apps in a fully-managed serverless environment where you can focus on using the foundation models via API calls without worrying about deploying the underlying infrastructure to run these models.
If you want to take it a step further in customization by either wanting to use models that are not available on Amazon Bedrock, or want to deploy the models yourself, and host them on compute instances with specific CPU and GPU capacities, we have that covered too- with Amazon Sagemaker.
Amazon SageMaker JumpStart is a machine learning hub where you can evaluate, compare, and select FMs to perform tasks like article summarization and image generation. Pre-trained models are fully customizable for your use case with your data, and you can deploy them into production with AWS SDK.
Using Amazon SageMaker for your generative AI project requires a few more steps than what we saw with PartyRock and Bedrock. Follow along if you want to set it up now.
Start by going to SageMaker on the AWS console. If this is your first time using SageMaker, you will have to first create a SageMaker domain and create a User Profile to use with the domain. Once ready, select JumpStart from the left panel and go to Foundation models. From the available models, you can view the model you want to use and select Open notebook in Studio which will take you to SageMaker Studio which contains SageMaker Jumpstart models.
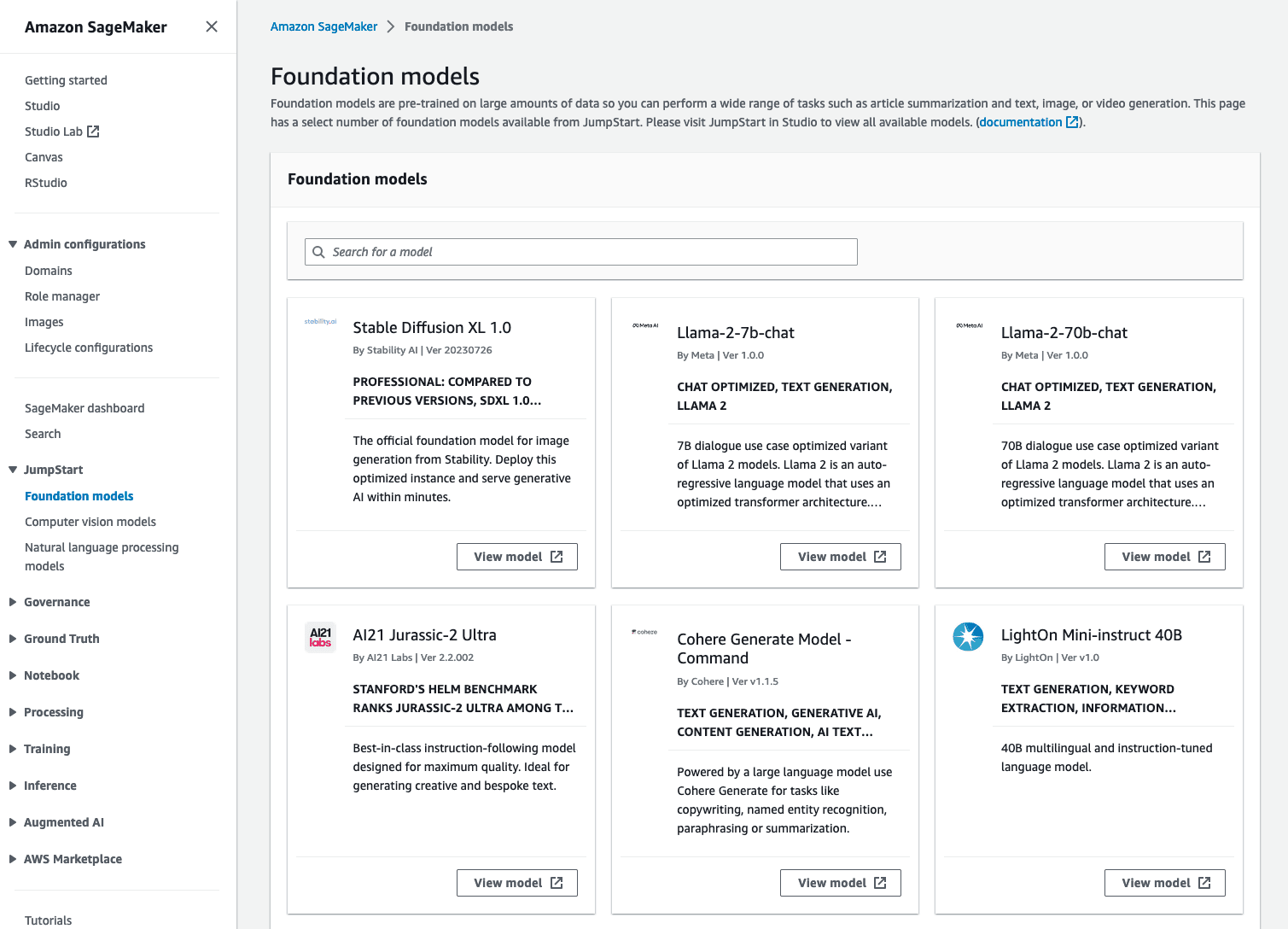
Just like the previous two times, I will once again use the example of creating a story of our two canine friends. This time I will use the HuggingFace Falcon 7B model from SageMaker JumpStart. You can customize the type of compute instance you want to use to host your model endpoint, the IAM role you want to use, whether the model should connect to a VPC, and what encryption keys you want to use to encrypt your data. I am keeping all options at default values and deploying the model to an endpoint.
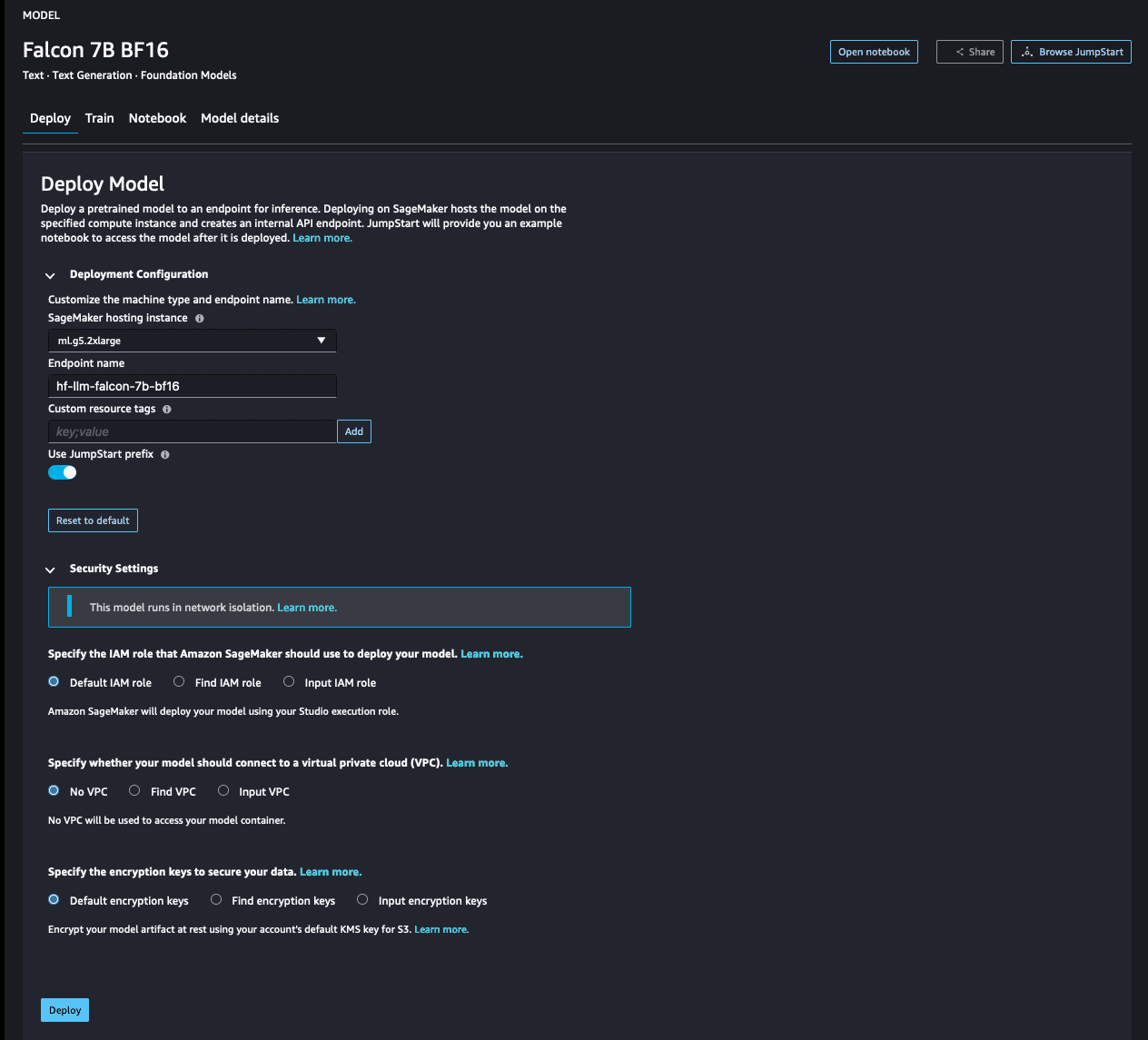
The model can be run in a Jupyter notebook with SageMaker API. You can run this notebook to test the model, or integrate it into your applications. Please note that running these models requires spinning up high-capacity EC2 instances which can incur a significant cost if you leave them running, so remember to terminate all resources immediately after use.
In the following example, I am providing the model context about the content I want to generate, and a query which can be used to change the scenario for which we want to generate the story. Together, they form the input prompt for the Falcon model.
client.invoke_endpoint
command calls the model and gets the response in JSON format which can be converted to plaintext and displayed to the end user, as seen in this screenshot-
You would have realized by now that SageMaker JumpStart gives you the most customization options and control over how and where you want to deploy your generative AI models for training and inference, but it also requires more effort to configure and operate. This option is generally preferred by data scientists and advanced machine learning users who prefer to have more control over the models and might also have existing AI and ML workflows where they want to integrate generative AI.
You can clone the SageMaker GenerativeAI Github repo to try out several different generative AI use-cases using a variety of different models on SageMaker. If you build something cool, share it here on AWS Community!
- If you are new to generative AI and want to learn the capabilities of what it can do, experiment with writing prompts and how it impacts the outputs generated by foundation models, all in a web UI without having to write any code - use PartyRock
- If you want to build generative AI applications using various foundation models accessed via APIs, want the ability to enhance the results with your proprietary datasets, without doing the heavy-lifting of managing the underlying infrastructure - use Amazon Bedrock
- If you prefer to use your own foundation models, or models not offered on Amazon Bedrock, or want to control and customize the infrastructure such as type of compute and GPU for training and inference jobs of your generative AI applications - use Amazon SageMaker
PS: The cover image for this blog post was generated using Stability AI SDXL generative AI model on Amazon Bedrock!
Any opinions in this post are those of the individual author and may not reflect the opinions of AWS.