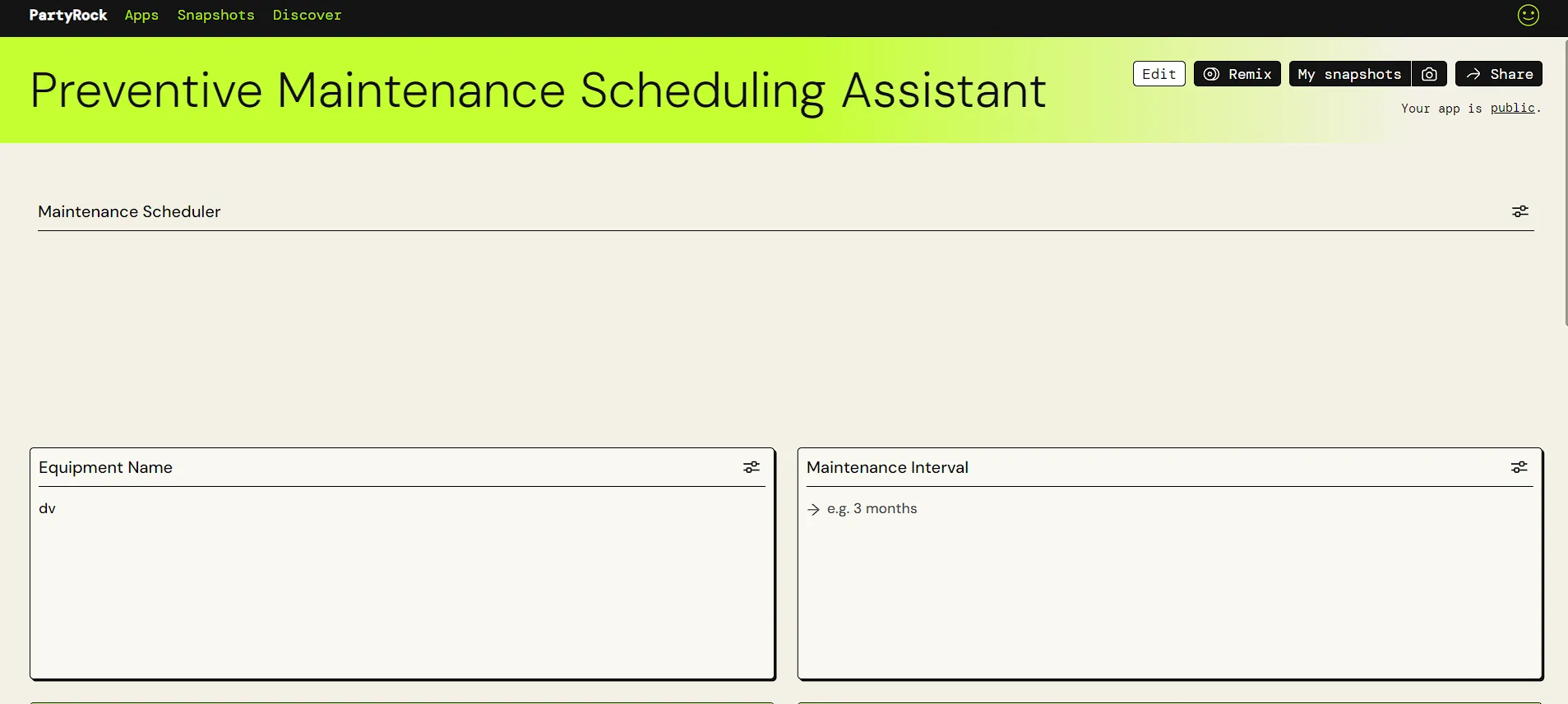
PREVENTIVE MAINTENCE SCHEDULING USING AI AND IOT
This project provides an overview of our project, focusing on optimizing preventive maintenance scheduling using Artificial Intelligence (AI) and the Internet of Things (IoT). Our goal is to improve operational efficiency by minimizing downtime and maximizing productivity through optimized maintenance schedules
Published Mar 3, 2024
We face the challenge of efficiently scheduling maintenance tasks to ensure minimal disruption to operations while considering factors such as machine usage patterns, wear and tear, resource availability, and budget constraints.
Our solution involves leveraging AI algorithms, specifically a Decision Tree Machine Learning model, to predict maintenance needs. Additionally, we employ IoT devices equipped with sensors for real-time monitoring, allowing for proactive maintenance interventions.
Our project stands out for its innovative integration of IoT and machine learning technologies to enable real-time predictive maintenance. This approach has broad applications across industries, offering automated alerts and improving operational sustainability
Implementation involves collecting and analyzing data from machine databases, installing IoT devices for real-time monitoring, and deploying a predictive maintenance system based on the trained AI model.
We validate the accuracy of our predictive maintenance system through data collection, model training, and scenario simulations. Continuous performance evaluation and user feedback drive iterative improvements.
Our cost estimation covers expenses such as IoT device procurement, software development, deployment, infrastructure setup, and miscellaneous costs. A detailed breakdown is provided based on the number of machines and specific maintenance requirements.
https://partyrock.aws/u/anandram006/HyJg28TsC/Preventive-Maintenance-Scheduling-Assistant/snapshot/JRtPdE4sW