Chat with Bedrock in the Amazon Connect contact control panel 🎧 🤖
Enable your contact center agents to have conversations with AI assistants in the same place that they receive customer calls
Ignacio Sanchez Alvarado
Amazon Employee
Published Apr 24, 2024
Last Modified May 16, 2024
Let's see how we can link an Amazon Bedrock assistant with our Amazon Connect contact center. Although there are already built-in generativeAI capabilities in Amazon Connect the Connect agents can also chat directly with a genAI model using the contact control panel with this integration.
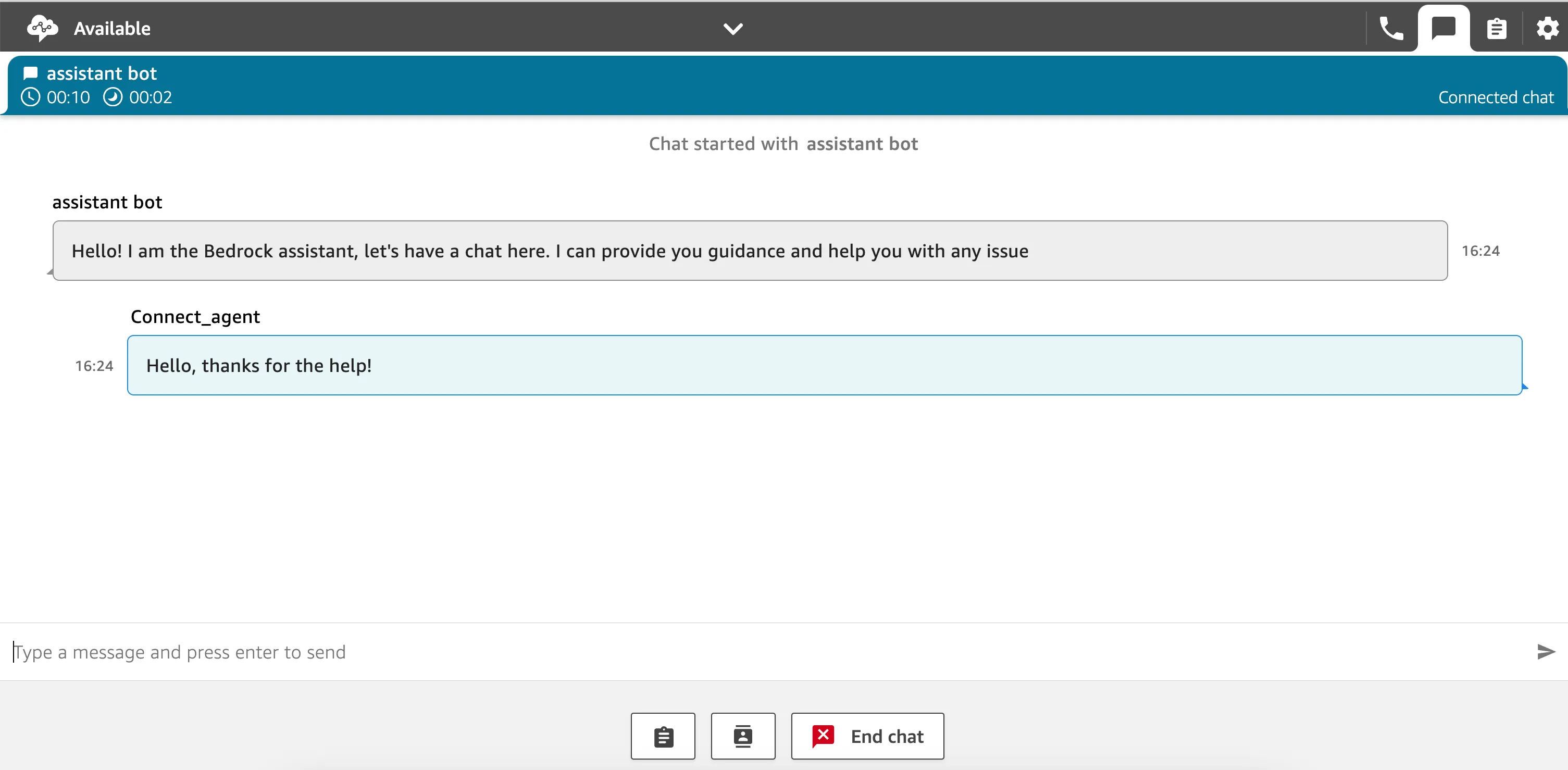
For this solution I used the Amazon Connect real-time chat message streaming feature that allows to integrate Amazon Connect chats in external chat services.
I also used AWS lambda to act as the link between Bedrock and the Amazon Connect APIs.
Finally I used DynamoDB for matching the conversation IDs between Bedrock and the Connect chat.
Note: regarding Bedrock usage I decided to leverage the Agents for Amazon Bedrock capability, as this comes with a managed chat feature that handles automatically conversation history and makes things simpler. Would also be possible to use directly the bedrock invokeModel API and implement a conversation history by ourselves.
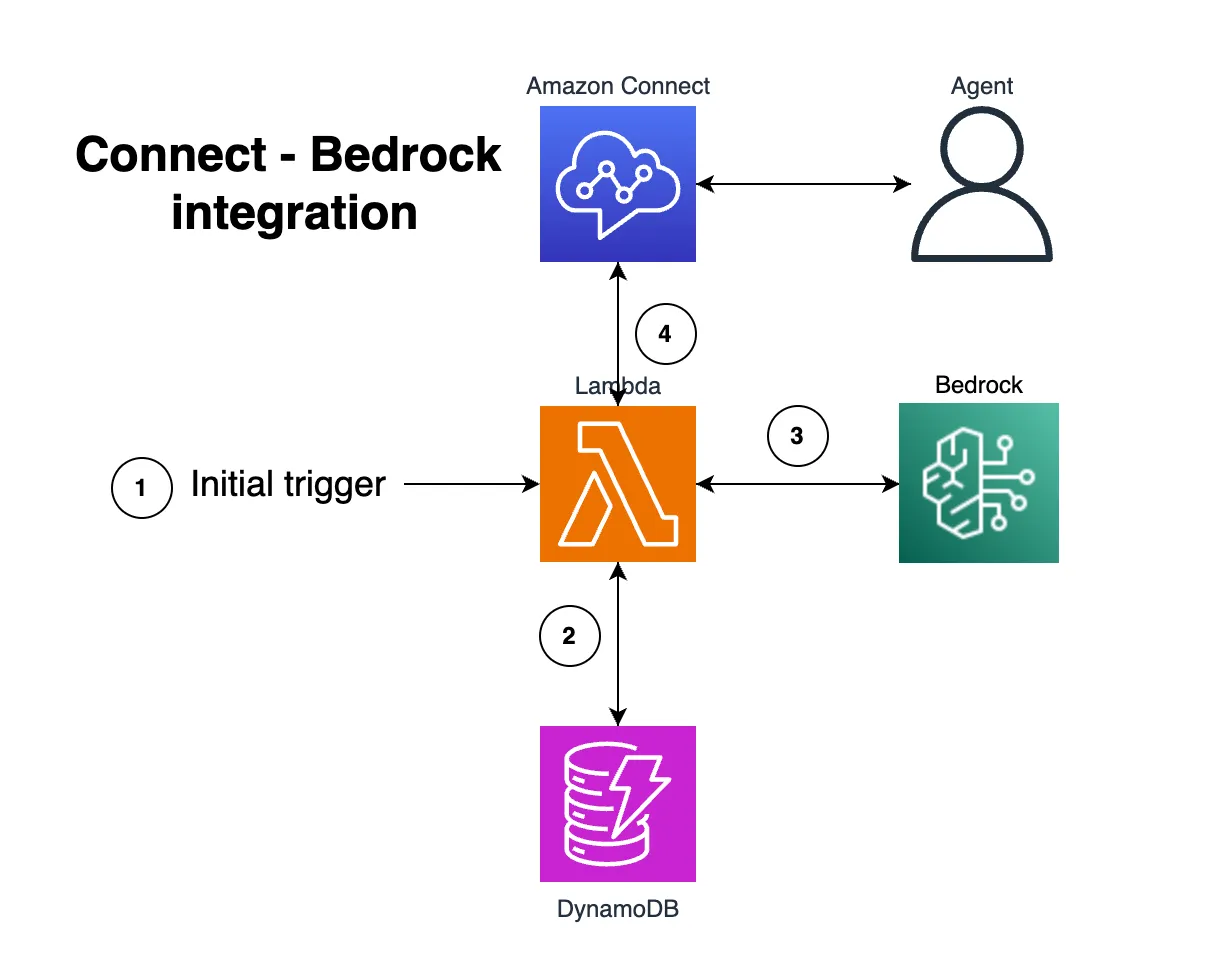
First some initial requirements:
- Create a dynamoDB table with the next partition key: "contact_id" (String) and the rest default settings (see Create a table example).
- Check the Amazon Connect instance ID and contact flow ID (see Find the flow ID). This will be the Connect contact flow that will receive the Bedrock conversation. Check also that your Connect agents have access to this contact flow and its queue (see How routing works).
- If you don't have yet an Amazon Connect instance check the Get started with Amazon Connect guide. In just some minutes you can start your own contact center instance in the cloud!.
- Create a standard SNS topic as explained in the real-time chat message streaming guide step1. (FIFO topics don't work with this!!).
- Create an Amazon Bedrock agent (see Create an agent in Amazon Bedrock guide).
- Create a SQS queue as the destination of the SNS topic. (see Fanout to Amazon SQS queues):
- Set the Visibility timeout to 60 seconds, you can leave the rest default parameters.
- In the SNS console select the SQS subscription and add the next message attribute policy:
Now let's create the main lambda function of the solution. This function will use the StartChatContact Api to start a new Connect conversation, then it uses the Bedrock InvokeAgent Api to send the message to the Bedrock model and finally it uses the send_message Api to send Bedrock response back to the Connect agent.
Go to the lambda console and create a new lambda function with Python as runtime and the rest of default configuration parameters. Once the function is created is necessary to configure several things:
- Increase timeout value to over 1min. Most of generative AI models will take several seconds to elaborate the answer (see Configure Lambda function timeout).
- Add lambda role next permissions policies: Bedrock access, Amazon connect access, Dynamodb access and Amazon SQS access (see Lambda execution role and AWS managed policies).
- Add the previously created SQS queue as the lambda trigger with the next configuration: batch size = 1.
- Create the next environment variables with your own parameters. You can get the agent_id and alias_id from bedrock agent console, the contact_flow_id and instance_id from Amazon Connect console, the dynamo_table name from DynamoDB and the sns_endpoint_arn from the SNS topic console:
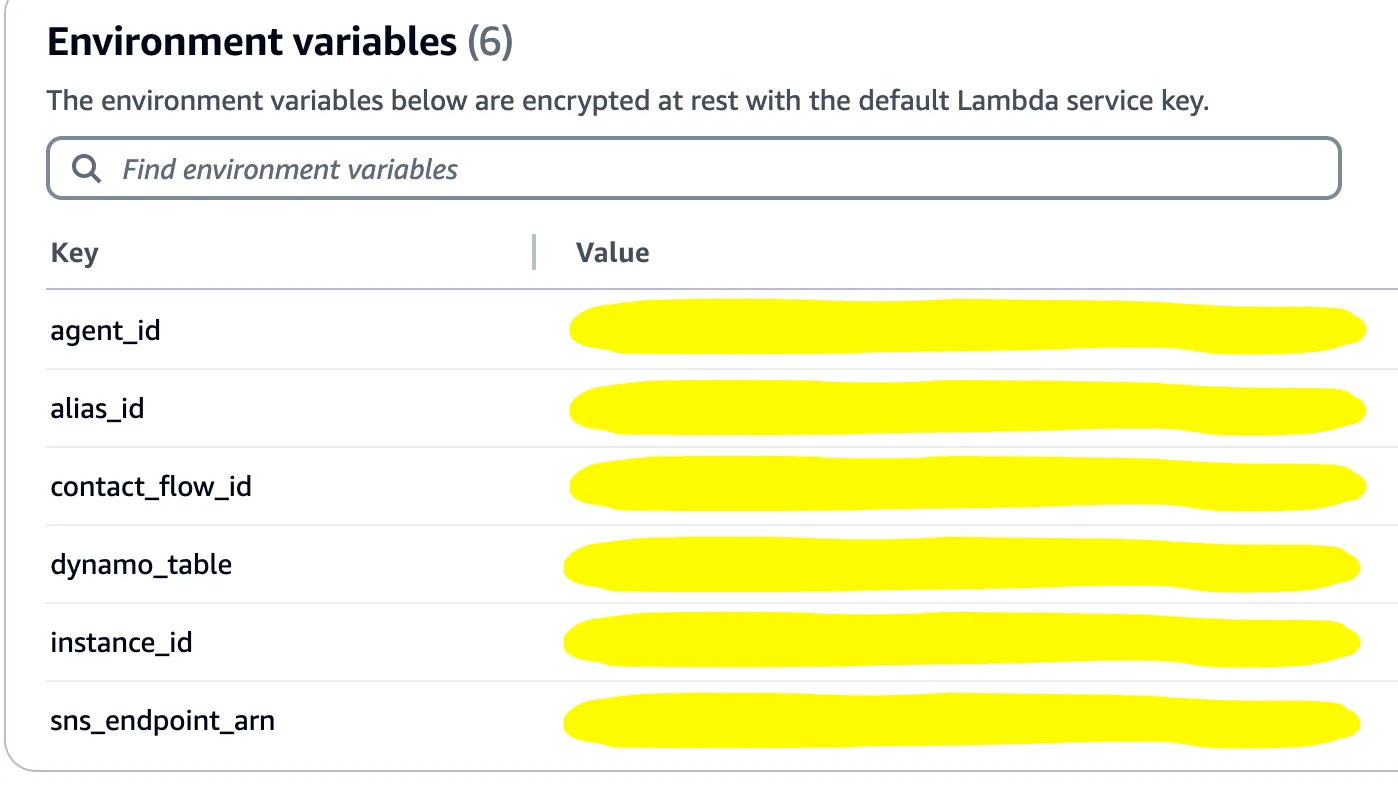
With this now you can copy paste the next code into the lambda code editor:
 You can explore the comments in the code to understand better what the function is doing. The most important points are the next ones:
- Start a new Connect conversation. This step is only executed if the message doesn't comes from the SQS trigger as this means that is a new conversation (See if-else logic in the lambda handler). In the code this is done with the function: start_agent_conversation.
- Saving and reading the connection_token (for the Amazon Connect conversation) and the session_id (For the Bedrock conversation). We have to match this two parameters to be able to have the same conversation in the Connect panel and in the Bedrock session. We use a DynamoDB table to store these values ​​persistently. In the code this is done with the functions: save_conversation_id and get_session_and_token.
- Send the Connect response to the Bedrock model. In the code this is done with the function: start_agent_conversation.
- Send the Bedrock response to Connect. In the code this is done with the send_message Api call.
We are ready to start receiving Bedrock calls in our Connect Control Panel. We just need to trigger the lambda and the agent will receive a new conversation message from Bedrock.
You can use the test function of lambda to do the trigger. Test event example, this is the first message that the Bedrock model will receive so you can tailor it to your specific use case:
For a production environment one common approach would be to use an Amazon API Gateway to trigger the lambda. Then this API can be integrated in a web app with a trigger button, an application with automated triggers or even into a Connect contact flow and let the same Connect agents to trigger the bedrock conversation. The flexibility is huge!
Once the lambda has been triggered we will receive a call in our Amazon Connect queue.
Let's see how this finally looks like:
For this specific conversation I created a Bedrock assistant in a retail business that has access to different company systems like current customer opened issues. I configured to trigger this assistant every X hours to do calls to the Connect agents and inform them about the current status of the issues.
As this is a generative AI assistant the connect agents can then engage in a conversation for obtaining guidance in resolving those issues. See the example below:
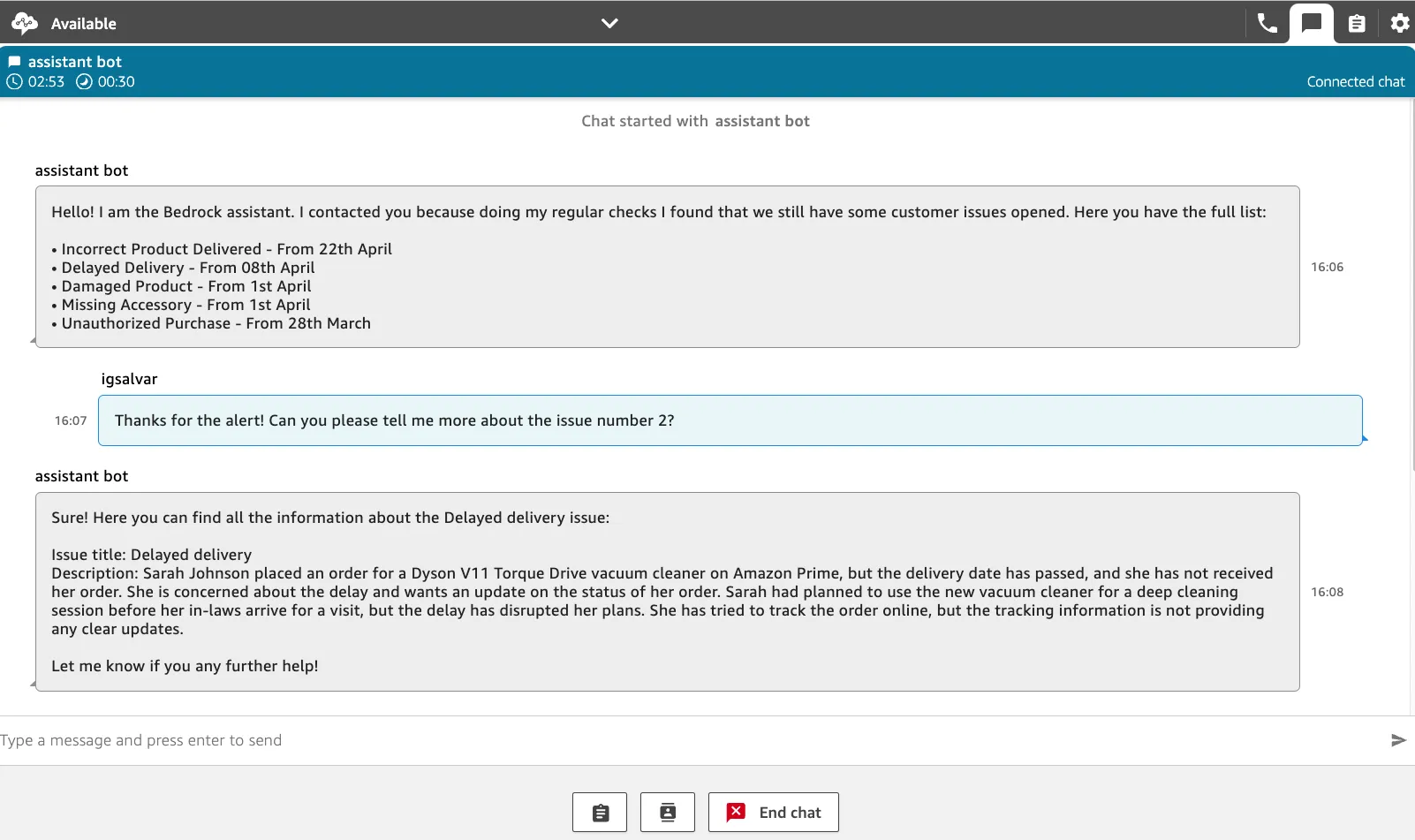
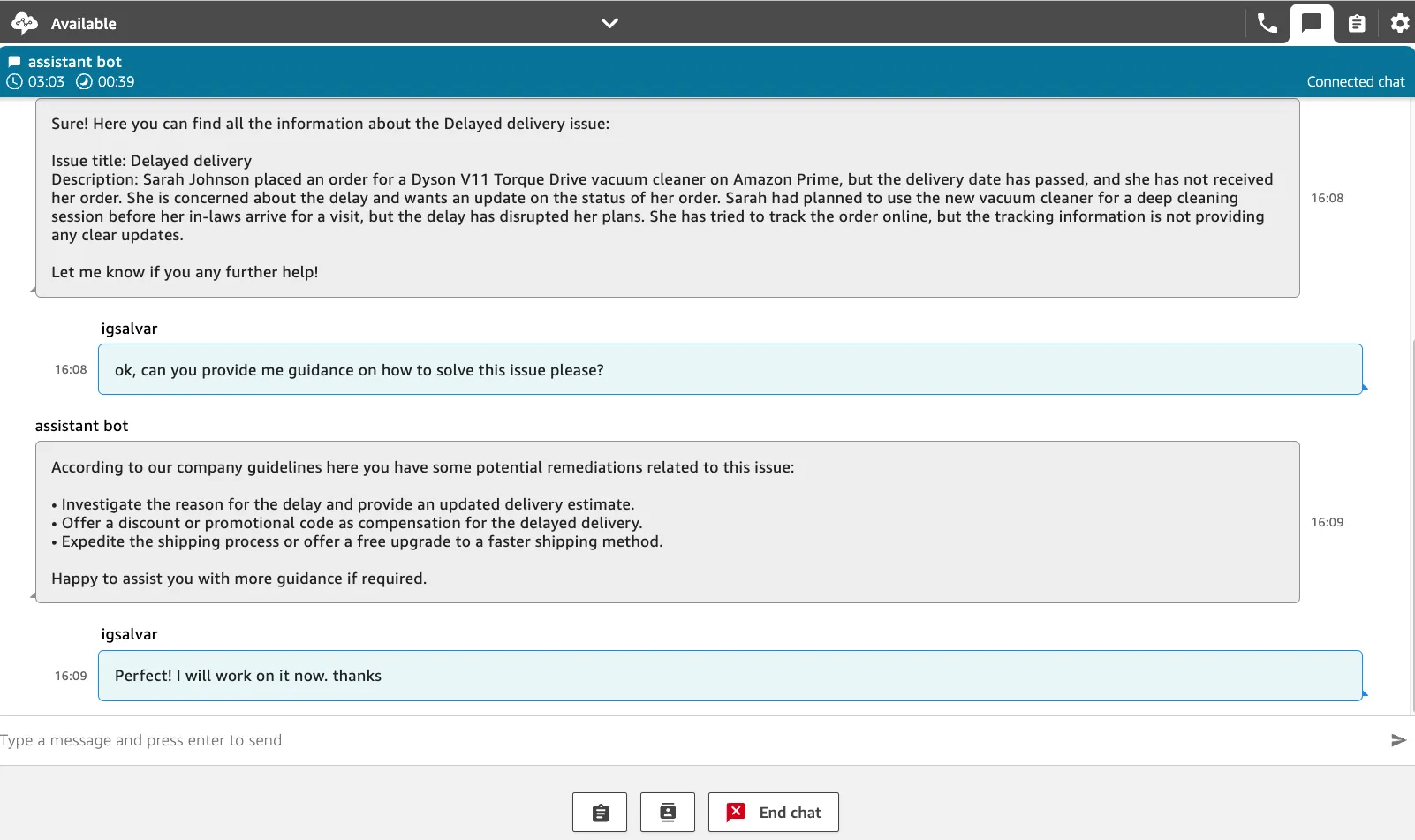
There are a lot of different possibilities and use cases to implement thanks to Amazon Bedrock models and the agent feature.
From assistants that review open issues in your contact center, to productivity helpers and / or models that access company systems and perform actions on your behalf.
The API usage of Amazon Bedrock gives the service a lot of flexibility, this was an example of an external integration with Amazon Connect, but similarly to this agents can be embedded in other communication applications like Slack, Whatsapp, Teams etc...
Keep and eye on new post where I will explore more about this other integrations.
For more information on this topic check the next resources:
Building with Amazon Bedrock:
Any opinions in this post are those of the individual author and may not reflect the opinions of AWS.