Sustainability at the core of data strategy
A 3-part series taking a deeper look into building a sustainable data management practice. This post talks about designing a data strategy that implements technology and deploys workloads with sustainability as a core principle.
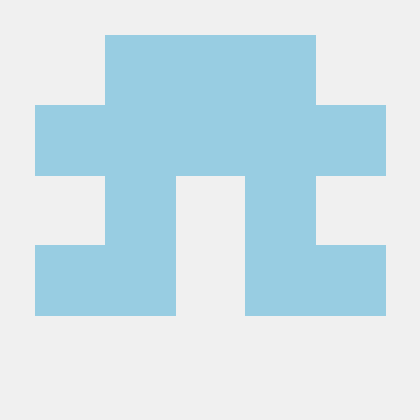
This is part 2 of a 3-part series:
Data management practices have evolved significantly with technological advancements. However, with the current emphasis on environmental consciousness and energy efficiency, it is essential to design data strategy through a sustainability lens. Organizations create data strategies considering the three pillars of people, process, and technology – sustainability touches all three pillars.
In this post, we will focus on the technology pillar with an aim to guide you on how to weave sustainability into your data management strategies, ensuring efficient resource utilization and reduced wastage while staying agile and scalable.
To unlock the value of data at scale, you will need more than singular data solutions. Given the diverse use cases, tools, and evolving needs, an end-to-end data strategy is essential for adopting sustainable data management practices. Refer to the AWS modern data architecture page to find out how you can build such a strategy on AWS. By embedding sustainability into the core of your data strategy, you ensure that every data-related decision, from collection, storage, processing, consumption, and governance, aligns with sustainability principles. The data analytics lens of the Well-architected framework will help you embed sustainability principles for designing new or optimizing existing data management processes. The diagram below illustrates the five key principles for building sustainable practices for data management. It is important to apply these strategies on end-to-end data lifecycle from data ingestion to data consumption.

As I mentioned previously, in traditional data management practices, businesses usually set up large servers, provisioned for peak traffic. But outside of those peaks, those servers are underutilized, consuming electricity. It is like running a large furnace for a small pot of soup. Cloud services provide a better solution with on-demand capacity provisioning through auto- scaling – automatically scaling up compute during peaks and scaling down as demand subsides. Not only do you maximize utilization, but with a pay-as-you-go model, you just pay for what you use.
Consider the following best practices in your architecture decisions for maximizing utilization and reducing waste:
Use managed services where suitable: Managed services perform infrastructure provisioning and on-demand scaling without you having to manage them. This removes overhead on your part and since AWS takes that responsibility - with dedicated teams experienced in managing infrastructure, it is done efficiently.
Use the correct type of compute: When using provisioned compute qualify the right kind of compute for the workload. Using the right type of compute suitable for the workload would improve efficiency. For non-critical jobs use spare EC2 capacity in the data center through Amazon EC2 Spot instances, it also reduces cost of your data processing jobs by 90%. Learn from recommendations in AWS Compute Optimizer for optimal resource configurations on EC2 instances, EBS volumes, ECS configurations, etc.
Reduce storage footprint: Design processes with the principle of reducing the storage footprint. Create ingestion processes to filter out unnecessary data, compress data objects before you store them so they consume less space, and use the right format to store objects so they can be consumed efficiently by downstream processes. You can also implement processes to automate general clean-up mechanisms to remove duplicate or unused data.
As a data team you need to combine tools, resources, and processes for ingesting, storing, querying data, building machine learning models, and ultimately helping end users develop data-driven insights. Your architecture strategy should include processes that qualify the right tool for the job. When you use the right tool for the right purpose, you gain greater flexibility in optimizing resource usage. For example, an object storage is specifically designed for storing unstructured files like images and videos. For transactional data, a database optimized for fast operations would provide optimal performance efficiency. Using the right storage type for the data means the services operate in their natural state and are more efficient. Would you ever use a sledgehammer to hang a picture?
Consider negotiating Service Level Agreements with data consumers where occasional, scheduled downtime are acceptable during off-peak hours. For disaster recovery, agree on a Recovery Time Objective (RTO) and Recovery Point Objective (RPO) that is optimal an works for the business. Adopt colder recovery strategies where possible to reduce parallel running of redundant resources on the secondary domain. It will cut down costs, allow you to be more energy-efficient, and still meet your business needs. For data dissemination, optimize processes to meet SLA, not over-achieve them.
With customers, suppliers and partners across the globe, data transfer can be a significant load, especially if you are sending extensive datasets for minor updates. By sending only the changes or updates across the network, you would conserve bandwidth, reduce costs, and minimize the energy footprint of data transfers. Explore techniques where access to data is federated, and data is not moved over the network. For example, if you are using S3 cross-region replication to move data stored in S3 between two AWS regions, check whether sharing the data using AWS Lake Formation managed catalog could replace the replication process. AWS services like Amazon Athena and Amazon Redshift also provide federated query connectors to many external data sources for reading data without importing them locally.
Data governance involves standardizing data formats, ensuring data quality, managing security policies, and maintaining metadata, all of which streamline data integration, transformation, and processing. By implementing standardized procedures, you can reduce the computational overhead often linked to handling disparate data formats, leading to energy savings and improved efficiency. With proper data lineage and metadata management, troubleshooting and data auditing become more efficient, minimizing resource-intensive operations. Regular housekeeping as a part of governance ensures that storage is optimized and that data processing pipelines are not working with irrelevant or redundant data. A strong data governance framework reduces the strain on infrastructure, promotes efficient use of computational resources, and aligns data management with sustainability objectives.
Embedding sustainability into data management is more than just an eco-friendly initiative; it is a strategic approach that can offer cost savings, improve efficiency, and nurture responsible growth. By focusing on resource optimization, using purpose-built tools, negotiating eco- friendly SLAs, minimizing data transfers over the network, and establishing robust governance, organizations can ensure they are on the right path to sustainable data practices. Adopting such measures not only reduces our carbon footprint but also sets a precedent for the future of data management.
In the next section we will learn how you can adopt sustainable data management practices in your day-to-day operations.
Any opinions in this post are those of the individual author and may not reflect the opinions of AWS.