Automate your Apache Airflow Environments
In this tutorial you will learn how to scale the deployment of your workflows into your Apache Airflow environments.
Automating Your Managed Workflow for Apache Airflow Environments (MWAA)
Recap of Automating Your Managed Workflow for MWAA and next steps
Automating Connections and Variables
Integrating AWS Secret Manager
Recap of Automating Connections and Variables and next steps
Building a Workflow Deployment Pipeline
Implementing intermediary Step
Recap of Building a Workflow Deployment Pipeline and next steps
- What are the common challenges when scaling Apache Airflow, and how can you address those problems
- How to automate the provisioning of the Apache Airflow infrastructure using AWS CDK
- How to automate the deployment of your workflows and supporting resources
About | |
---|---|
โ AWS Level | 200 - Intermediate |
โฑ Time to complete | 90 minutes |
๐ฐ Cost to complete | Approx $25 |
๐งฉ Prerequisites | - This tutorial assumes you have a working knowledge of Apache Airflow - AWS Account - You will need to make sure you have enough capacity to deploy a new VPC - by default, you can deploy 5 VPCs in a region. If you are already at your limit, you will need to increase that limit or clean up one of your existing VPCs - AWS CDK installed and configured (I was using 2.60.0 build 2d40d77) - Access to an AWS region where Managed Workflows for Apache Airflow is supported - git and jq installed - The code has been developed on a Linux machine, and tested/working on a Mac. It should work on a Windows machine with the Windows Subsystem for Linux (WSL) installed although I have not tested this. If you do encounter issues, I recommend that you spin up an Amazon Cloud9 IDE environment and run through the code there. |
๐ป Code Sample | Code sample used in tutorial on GitHub |
๐ข Feedback | Help us improve how we support Open Source at AWS |
โฐ Last Updated | 2023-04-14 |
- Apache Airflow is a complex technology to manage, with lots of moving parts. Do you have the skills or the desire to want to manage this?
- There is constant innovation within the Apache Airflow community, and your data engineers will want to quickly take advantage of the latest updates. How quickly are you able to release updates and changes to support their need?
- How do you ensure that you can provide the best developer experience and to help minimise the issues with deploying workflows to production?
- Ensuring that you bake security from the beginning, how can you separate concerns and make sure that you minimise the number of secrets that developers need access to?
- Deploying workflows to production can break Apache Airflow, so how do you minimise this?
- New Python libraries are released on a frequent basis, new data tools are also constantly changing. How do you enable these for use within your Apache Airflow environments?
- Whether you need the increase level of access, a greater level of control of the configuration of Apache Airflow
- Whether have the need to have the very latest versions or features of Apache Airflow
- Whether you have the need to run workflows that use more resources that managed services provide (for example, need significant compute)
Total Cost Ownership One thing to consider when assessing managed vs self-managed is the cost of the managed service against the total costs of you having to do the same thing. It is important to assess a true like for like, and we often see just the actual compute and storage resources being compared without all the additional things that you need to make this available.

- create a VPC into which MWAA resources will be deployed (See the architecture diagram above)
- ensure we have a unique S3 bucket that we can define for our Airflow DAGs folder
- determine whether we want to integrate Airflow Connections and Variables with AWS Secrets Manager
- create our MWAA environment
The code is available in the supporting repository.
dagss3location
- this is the Amazon S3 bucket that MWAA will use for the Airflow DAGs. You will need to ensure that you use something unique or the stack will failmwaa_env
- the name of the MWAA environment (that will appear in the AWS console and all cli interactions)
mwaa_secrets_var
- this is the prefix you will use to integrate with AWS Secrets Manager for Airflow Variablesmwaa_secrets_conn
- this is the prefix, as the previous, but for Airflow Connections.
MwaaCdkStackVPC
is used to create our VPC resources where we deploy MWAA. MwaaCdkStackDevEnv
is used to create our MWAA environment. MwaaCdkStackDevEnv
has a dependency on the VPC resources, so we will deploy this stack first. Let us explore the code:MwaaCdkStackDevEnv
stack that creates our MWAA environment into the VPC that we just created. The code is documented to help you understand how it works and help you customise it to your own needs. You will notice that we bring in parameters we defined in the app.py
using f"{mwaa_props['dagss3location']
, so you can adjust and tailor this code to your own needs if you wanted to add additional configuration parameters.Note: This code creates an S3 Bucket with the name of the configuration parameter and then appends-dev
, so using our example code the S3 Bucket that would get created ismwaa-094459-devops-demo-dev
.
Y
to start the deployment

Read more Check out this detailed post to dive even deeper into this topic.
app.py
to allow us to easily set what we want the prefix to be. We do not want to hard code the prefix for Connections and Variables, so we define some additional configuration parameters in our app.py
file that will use airflow/variables
and airflow/connections
as the integration points within MWAA:How does this work? To define variables or connections that MWAA can use, you create these in AWS Secrets Manager using the prefix you defined. In the above example, we have set these toairflow/variables
andairflow/connections
. If I create a new secret calledairflow/variable/foo
then from within my Airflow workflows, I can reference the variable asfoo
usingVariable.get
within our Airflow code.
Dive Deeper Read the blog post from John Jackson that looks at this feature in more detail -> Move your Apache Airflow connections and variables to AWS Secrets Manager
Tip! If you wanted to provide a set of standard Variables or Connections when deploying your MWAA environments, you could add these by updating the CDK app and using the AWS Secrets constructs. HOWEVER make sure you understand that if you do this, those values will be visible, so do not share "secrets" that you care about. It is better to deploy and configure these outside of the provisioning of the environment so that these are not stored in plan view.
from airflow.models import Variable
) and then we just create a new variable within our workflow that grabs the variable we defined in AWS Secrets Manager (/airflow/variables/buildon
), but we just refer to it as buildon
. We also add a default value in case that fails, which can be helpful when troubleshooting issues with this.
redshift_default
as a connection within Apache Airflow, it will use these values. Some Connections require addition information in the Extras field, so how do you add these? Lets say the Connection needed some Extra data, we would add this by appending the extra info with ?{parameter}={value}&{parameter}={value}
. Applying this to the above we would create our secret like:- configure whether you want to use both Variables and Connections, or just one of them
- allow you to specify regular expressions to combine both native Airflow Variables and Connections (that will be stored in the Airflow metastore), and AWS Secrets Manager
aws-*
, so for example aws-redshift
, or aws-athena
.requirements.txt
file which we upload to an S3 bucket. Finally, if you want to deploy your own custom Airflow plugins, then these also need to be deployed to an S3 bucket and then the MWAA configuration updated.- need to have a source code repository where our developers will commit their final workflow code
- once we have detected new code in our repository, we want to run some kind of tests
- if our workflow code passes all test, we might want to get a final review/approval before it is pushed to our MWAA environment
- the final step is for the pipeline to deliver the workflow into our MWAA DAGs Folder
MWAAPipeline
). If we look at app.py
:code_repo_name
andbranch_name
which will create an AWS CodeCommit repository,dags_s3_bucket_name
which is the name of our DAGs Folder for our MWAA environment
MWAAPipeline
) is where we create the CodeCommit repository, and configure our CodePipeline and the CodeBuild steps. If we look at this code we can see we start by creating our code repository for our DAGs.$BUCKET_NAME
) so that we can re-use this pipeline.y
after reviewing the security information that pops up.
- running tests - you might want to ensure that before deploying the files to the S3 DAGs folder, that you run some basic tests to make sure they are valid and will reduce the likelihood of errors when deployed
- approvals - perhaps you want to implement an additional approval process before deploying to your production environments
test
, but you would add all the commands you would typically use and define them in this step. You could also include additional resources within the git repository and uses those (for example, unit tests or configuration files for your testing tools).cdk deploy mwaa-pipeline
, you will receive an email to confirm that you are happy to receive notifications from the approval process we have just setup (otherwise you will receive no notifications!).
So far we have just scratched the surface of how you can apply DevOps principles to your data pipelines. If you want to dive deeper, there are some additional topics that you can explore to further automate and scale your Apache Airflow workflows.Creating re-usable workflows will help scale how your data pipelines are used. A common technique is to create generic workflows that are driven by parameters, driving up re-use of those workflows. There are many approaches to help you increase the reuse of your workflows, and you can read more about this by checking out this post, Working with parameters and variables in Amazon Managed Workflows for Apache Airflow.When building your workflows, you will use Python libraries to help you achieve your tasks. For many organisations, using public libraries is a concern and they look to control where those libraries are loaded from. In addition, Development teams are also creating in-house libraries that need to be stored somewhere. Builders often use private repositories to help them solve this. In the post, Amazon MWAA with AWS CodeArtifact for Python dependencies, shows you how you how to integrate Amazon MWAA with AWS CodeArtifact for Python dependencies.Read the post, Automating Amazon CloudWatch dashboards and alarms for Amazon Managed Workflows for Apache Airflow which provides a solution that automatically detects any deployed Airflow environments associated with the AWS account and then builds a CloudWatch dashboard and some useful alarms for each.In the post, Introducing container, database, and queue utilization metrics for the Amazon MWAA environment, dives deeper into metrics you can better understand the performance of your Amazon MWAA environment, troubleshoot issues related to capacity, delays, and get insights on right-sizing your Amazon MWAA environment.
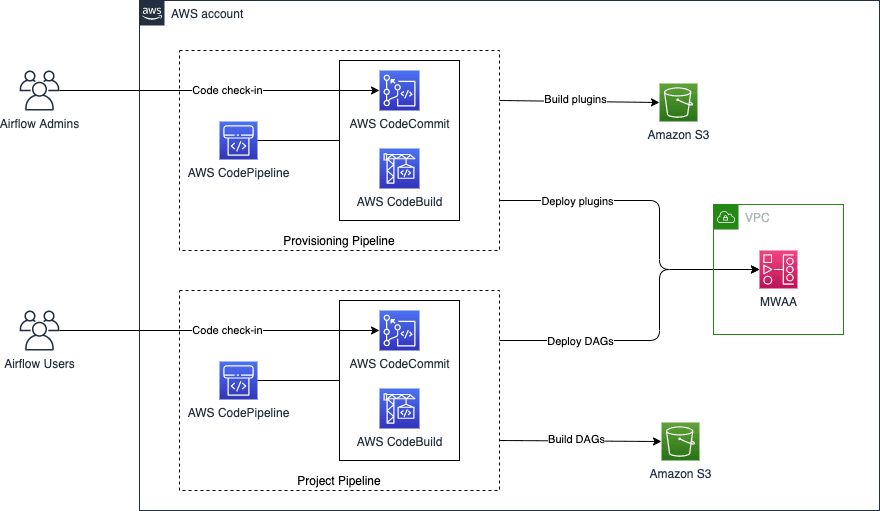
setup.py
is used to initialise Python, and makes sure that all the dependencies for this stack are available. In our instance, we need the following:app.py
, where we define our AWS Account and Region information. We then have a directory called mwaairflow
which contains a number of key directories:assets
- this folder contains resources that you want to deploy to your MWAA environment, specifically a requirements.txt file that allows you to amend which Python libraries you want installed and available, and then packages up and deploys a plugin.zip which contains some sample code for custom Airflow operators you might want to use. In this particular example you can see we have custom Salesforce operatornested_stacks
- this folder contains the CDK code that provisions the VPC infrastructure, then deploys the MWAA environment, and then finally deploys the Pipelineproject
- this folder contains the Airflow workflows that you want to deploy in the DAGs folder. This example provides some additional code around Python linting and testing which you can amend to run before you deploy your workflows
Makefile in our previous pipeline we defined the mechanism to deploy our workflows via the AWS CodeBuildBuildspec
file. This time we have created aMakefile
, and within it created a number of different tasks (test, validated, deploy, etc). To deploy our DAGs this time, all we need to do is run amake deploy $bucket_name=
specifying the target S3 bucket we want to use.
-- context
when performing the cdk deploy command, to pass in configuration values in a key/value.vpcId
- If you have an existing VPC that meets the MWAA requirements (perhaps you want to deploy multiple MWAA environments in the same VPC for example) you can pass in theVPCId
you want to deploy into. For example, you would use--context vpcId=vpc-095deff9b68f4e65f
.cidr
- If you want to create a new VPC, you can define your preferred CIDR block using this parameter (otherwise a default value of172.31.0.0/16
will be used). For example, you would use--context cidr=10.192.0.0/16
.subnetIds
- Is a comma separated list of subnets IDs where the cluster will be deployed. If you do not provide one, it will look for private subnets in the same AZ.envName
- a string that represents the name of your MWAA environment, defaulting toMwaaEnvironment
if you do not set this. For example,--context envName=MyAirflowEnv
.envTags
- allows you to set Tags for the MWAA resources, providing a json expression. For example, you would use--context envTags='{"Environment":"MyEnv","Application":"MyApp","Reason":"Airflow"}'
.environmentClass
- allows you to configure the MWAA Workers size (eithermw1.small
,mw1.medium
,mw1.large
, defaulting tomw1.small
). For example,--context environmentClass=mw1.medium
.maxWorkers
- change the number of MWAA Max Workers, defaulting to 1. For example,--context maxWorkers=2
.webserverAccessMode
- define whether you want a public or private endpoint for your MWAA Environment (usingPUBLIC_ONLY
orPRIVATE_ONLY
). For example, you would use--context webserverAccessMode=PUBLIC_ONLY
mode (private/public).secretsBackend
- configure whether you want to integrate with AWS Secrets Manager, using values Airflow or SecretsManager. For example, you would use--context secretsBackend=SecretsManager
.
mwaairflow_stack
file, which our app.py
file calls.
mwaa-provisioning
and mwaaproject
.mwaairflow_stack
file), and then push the change back to the git repository. This will kick off the AWS CodePipeline and trigger the reconfiguration.requirements.txt
file to update the Python libraries. We are going to update our MWAA environment to use a later version of the Amazon Provider package. We need to check out the repo, make the change and then commit it back.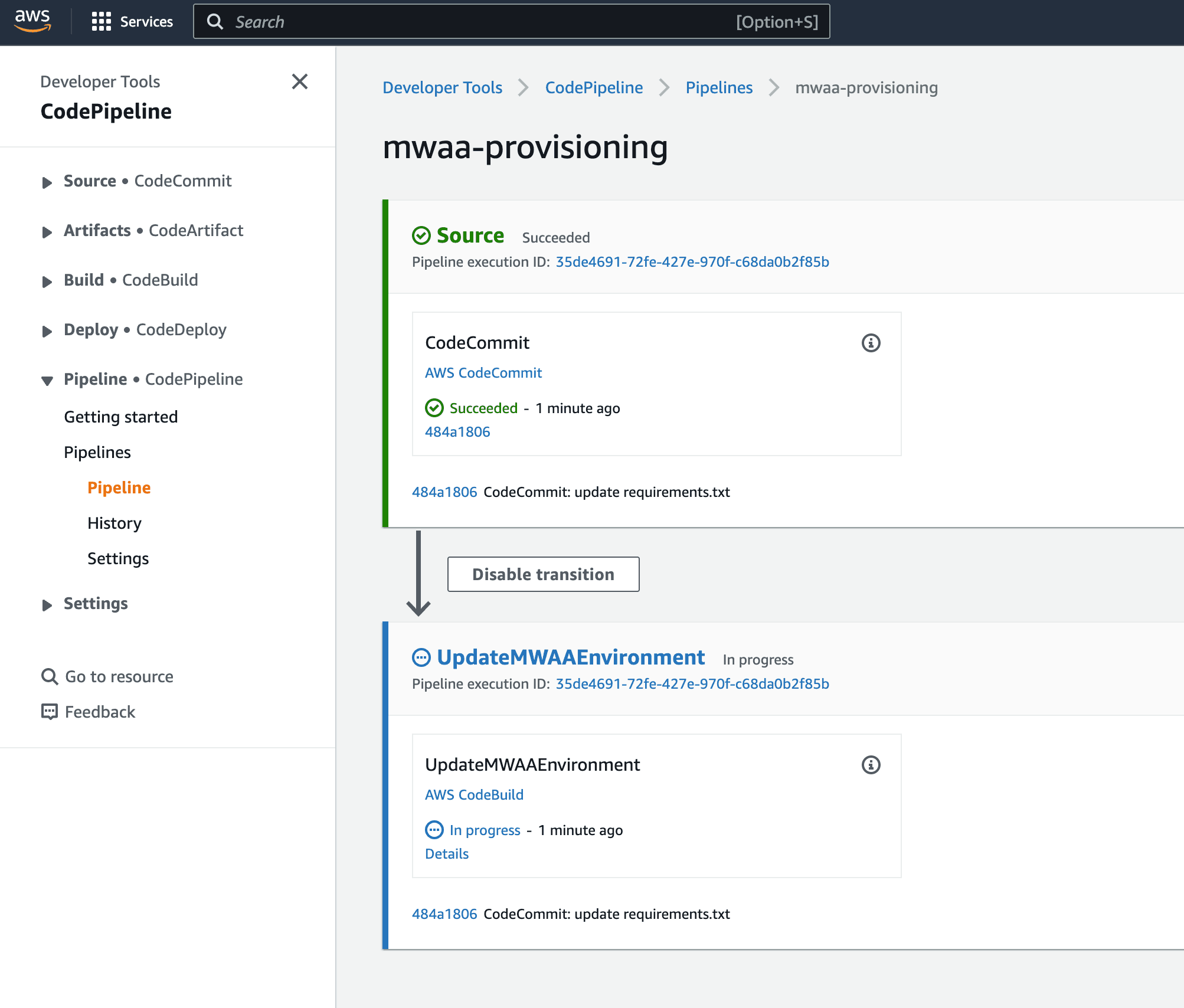

requirements.txt
has not been set by the MWAA environment. The reason for this is that this is going to trigger an environment restart, and so this is likely something you want to think about before doing. You could automate this, and we would add the following to the deploy part of the CodeBuild deployment stage:Tip! If you wanted to run this separately, just set thebucket_name
andmwaa_env
variables to suit your environment.
Makefile
deploy
task, copying the DAGs folder to our MWAA environment. You can use and adjust this workflow to do more complex workflows, for example, developing support Python resources that you might use within your workflows.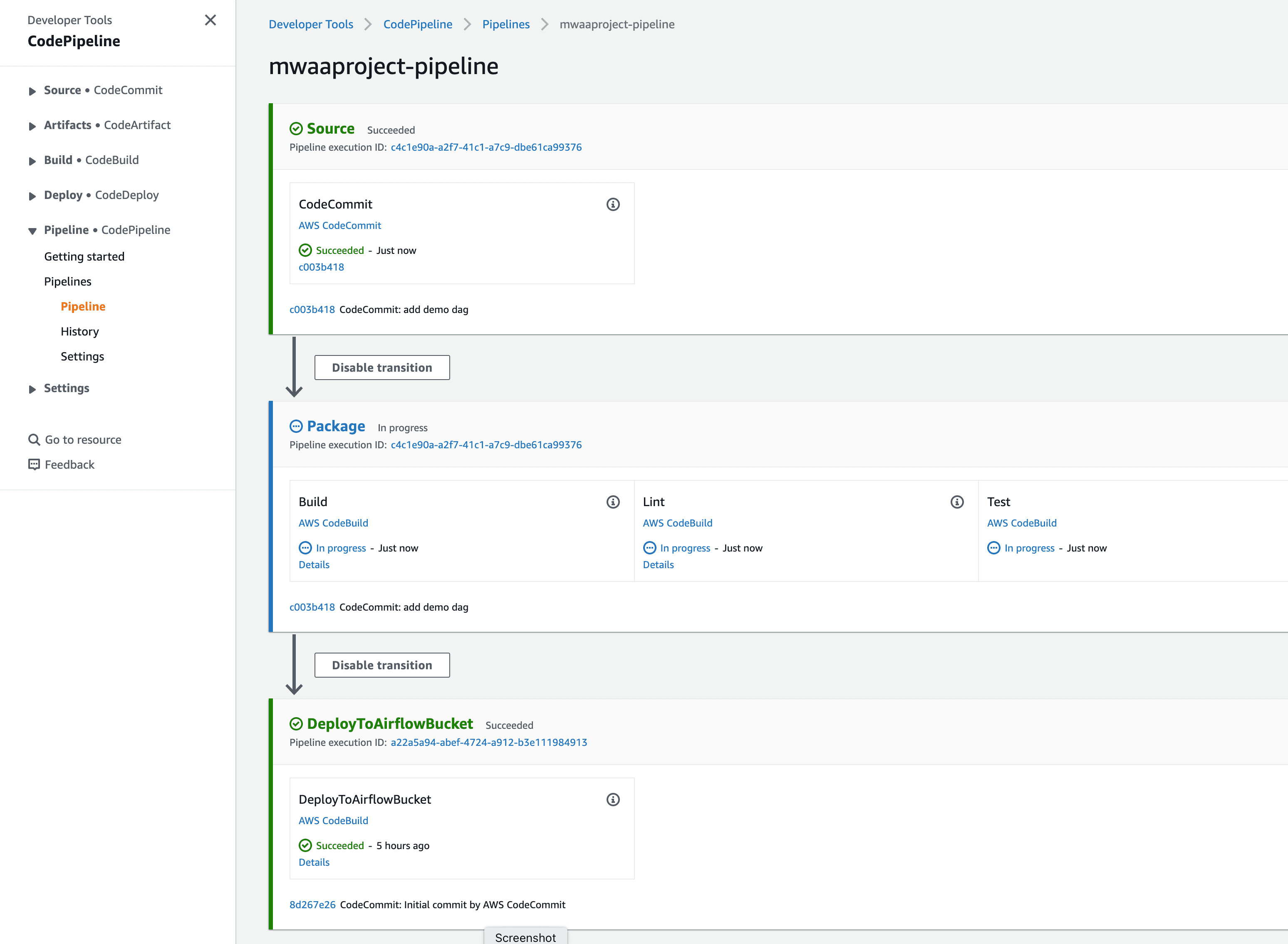

Check the CodeBuild logs If you want more details as to what happened during both the environment and workflow pipelines, you can view the logs from the CodeBuild runners.
Note: The delete process will fail at some point due to not being able to delete the S3 buckets. You should delete these buckets via the AWS Console (using Empty and then Delete), and then manually delete the stacks via the CloudFormation console.
Any opinions in this post are those of the individual author and may not reflect the opinions of AWS.