#TGIFun🎈 Building GenAI apps with managed AI services
Some random thoughts on managed AI services and their place in the GenAI stack... with examples.
💡 Did you know? You can try some of these services for *free* on the AWS AI Services Demo website!
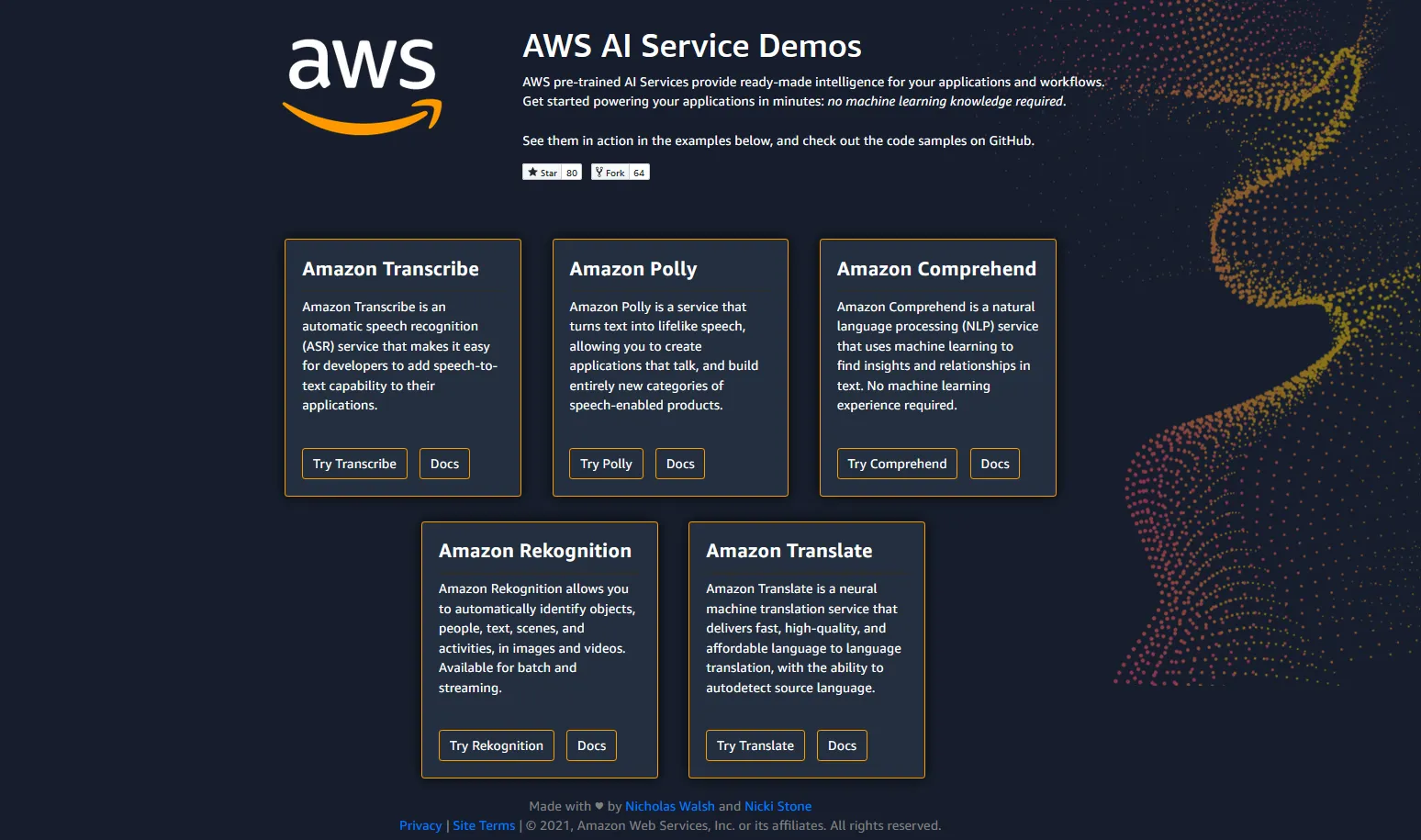
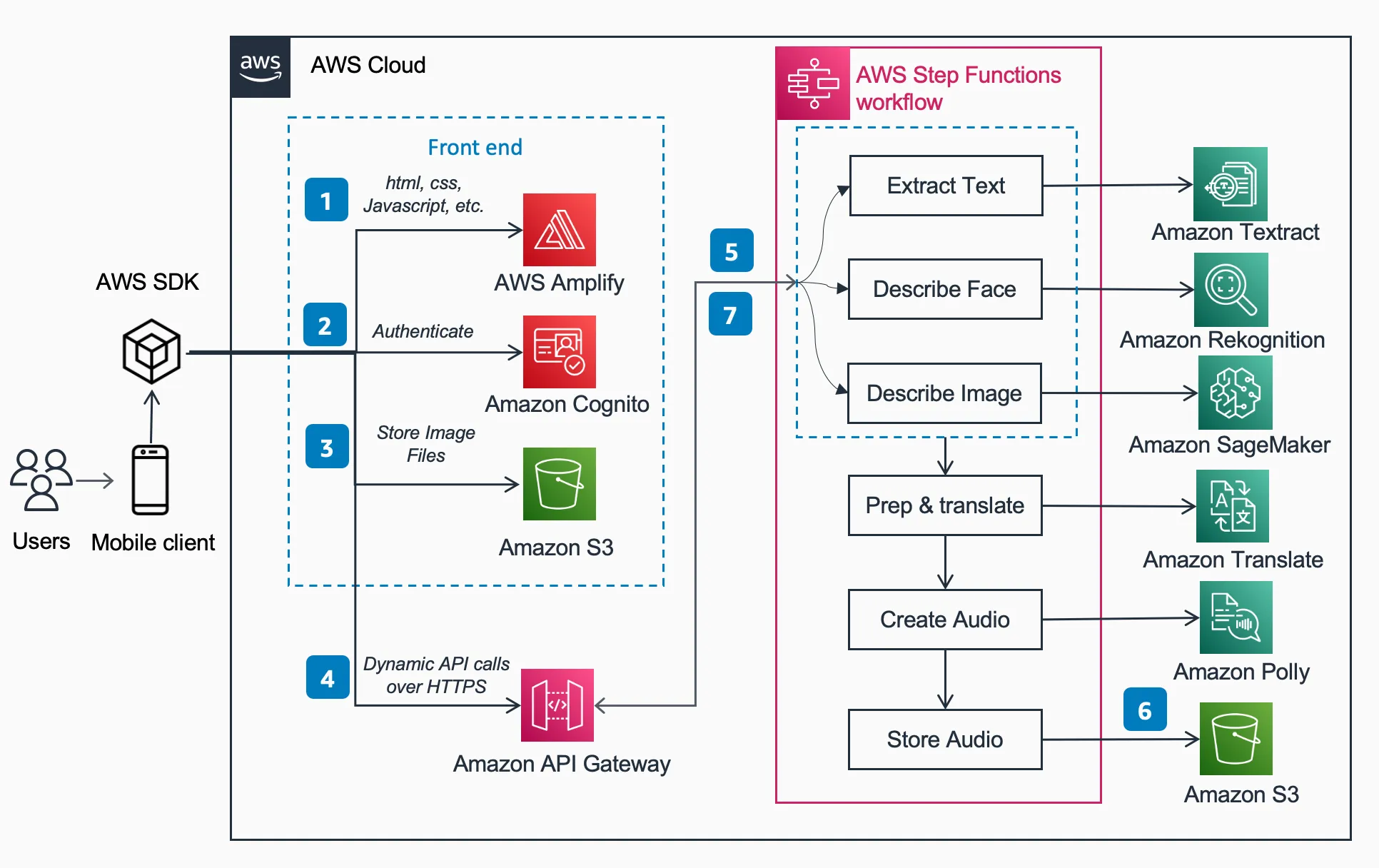
1
2
3
4
5
6
7
8
9
10
11
12
13
14
15
16
17
18
19
20
21
22
23
24
25
26
27
28
29
30
31
32
33
34
35
36
37
38
39
40
41
42
43
44
45
46
47
48
49
50
51
52
53
54
55
56
57
58
59
60
61
62
63
64
65
66
67
68
69
70
71
72
73
74
75
76
77
78
79
80
81
82
83
84
85
86
87
88
89
90
91
92
93
94
95
96
97
98
99
"""
Describe for Me (Bedrock edition ⛰️)
Image ==> Bedrock -> Translate -> Polly ==> Speech
"""
import argparse
import base64
import json
import boto3
# Parse arguments
parser = argparse.ArgumentParser(
prog='Describe for Me (Bedrock Edition) ⛰️',
description="""
Describe for Me is an 'image-to-speech' app
built on top of AI services that was created
to help the visually impaired understand images
through lively audio captions.
""",
)
parser.add_argument('image')
parser.add_argument('-m', '--model', default="anthropic.claude-3-sonnet-20240229-v1:0")
parser.add_argument('-t', '--translate')
parser.add_argument('-v', '--voice')
args = parser.parse_args()
# Initialize clients
bedrock = boto3.client("bedrock-runtime")
translate = boto3.client("translate")
polly = boto3.client("polly")
# Process image
with open(args.image, "rb") as image_file:
image = base64.b64encode(image_file.read()).decode("utf8")
# Generate a description of the image
response = bedrock.invoke_model(
modelId=args.model,
body=json.dumps({
"anthropic_version": "bedrock-2023-05-31",
"max_tokens": 1024,
"messages": [{
"role": "user",
"content": [{
"type": "text",
"text": "Describe the image for me.",
},
{
"type": "image",
"source": {
"type": "base64",
"media_type": f"image/{args.image.split('.')[-1]}",
"data": image,
},
}],
}],
}),
)
result = json.loads(response.get("body").read())
description = " ".join([output["text"] for output in result.get("content", [])])
print(f"##### Original #####\n\n{description}\n")
# Translate the description to a target language
if args.translate:
description = translate.translate_text(
Text=description,
SourceLanguageCode='en',
TargetLanguageCode=args.translate
)['TranslatedText']
print(f"##### Translation #####\n\n{description}\n")
# Read the description back to the user
if args.voice:
# Translate -> Polly language map
lang_map = {
'en': 'en-US',
'pt': 'pt-BR'
}
lang_code = lang_map.get(args.translate, args.translate)
# Synthesize audio description
print(f"\nSynthesizing audio description\n> Language: {lang_code}\n> Voice: {args.voice}\n")
audio_data = polly.synthesize_speech(
Engine="neural", # hardcoded, because we want natural-sounding voices only
LanguageCode=lang_code,
OutputFormat="mp3",
Text=description,
TextType='text',
VoiceId=args.voice,
)
# Save audio description
with open(f"{''.join(args.image.split('.')[:-1])}.mp3", 'wb') as audio_file:
audio_file.write(audio_data['AudioStream'].read())
pt-PT
) spoken by Polly's one and only Inês of Seurat's A Sunday Afternoon on the Island of La Grande Jatte:1
python describeforme.py --translate pt-PT --voice Ines seurat.jpeg
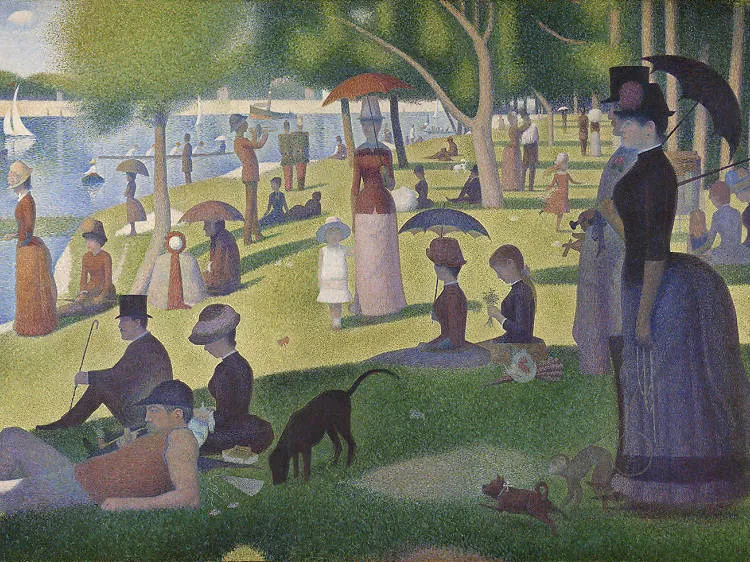
1
2
3
4
5
6
7
8
9
10
11
12
13
14
15
16
17
18
19
20
21
22
23
24
25
26
27
28
29
30
31
32
33
34
35
36
37
##### Original #####
The image depicts a park scene from the late 19th or early 20th century.
It's a lush green landscape with trees and people gathered enjoying the outdoors.
Many are dressed in fashions typical of that era, with men wearing top hats
and women in long dresses with parasols to shade themselves from the sun.
Some people are seated on the grass, while others are strolling along the paths.
There's a body of water in the background, likely a lake or river, with boats
and people gathered near the shoreline.
The style of painting is distinctive, with areas of flat color and simplified forms,
characteristic of the Post-Impressionist or early modern art movements.
The scene captures a leisurely day in an urban park setting, conveying a sense
of relaxation and appreciation for nature amidst the city environment.
##### Translation #####
A imagem retrata uma cena de parque do final do século XIX ou início do século XX.
É uma paisagem verdejante com árvores e pessoas reunidas a desfrutar do ar livre.
Muitos estão vestidos com modas típicas daquela época, com homens a usar chapéus
e mulheres em vestidos longos com guarda-sóis para se proteger do sol.
Algumas pessoas estão sentadas na relva, enquanto outras estão a passear pelos caminhos.
Há um corpo de água ao fundo, provavelmente um lago ou rio, com barcos
e pessoas reunidas perto da costa.
O estilo de pintura é distinto, com áreas de cor plana e formas simplificadas,
características dos movimentos de arte pós-impressionista ou do início da arte moderna.
A cena captura um dia de lazer num cenário de parque urbano, transmitindo uma sensação
de relaxamento e apreciação pela natureza em meio ao ambiente da cidade.
Synthesizing audio description
> Language: pt-PT
> Voice: Ines
🎯 Try it out for yourself and share the best descriptions in the comments section below 👇
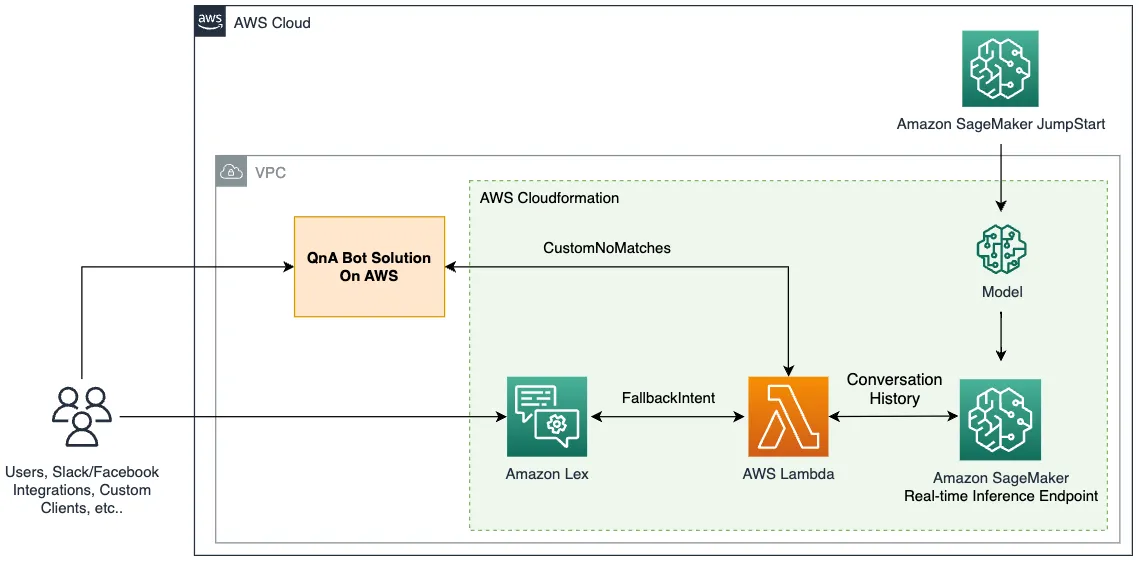
💡 If you want to know more about how Amazon Lex integrates with Lambda functions, read the section on Enabling custom logic with AWS Lambda functions in the Amazon Lex V2 Developer Guide.
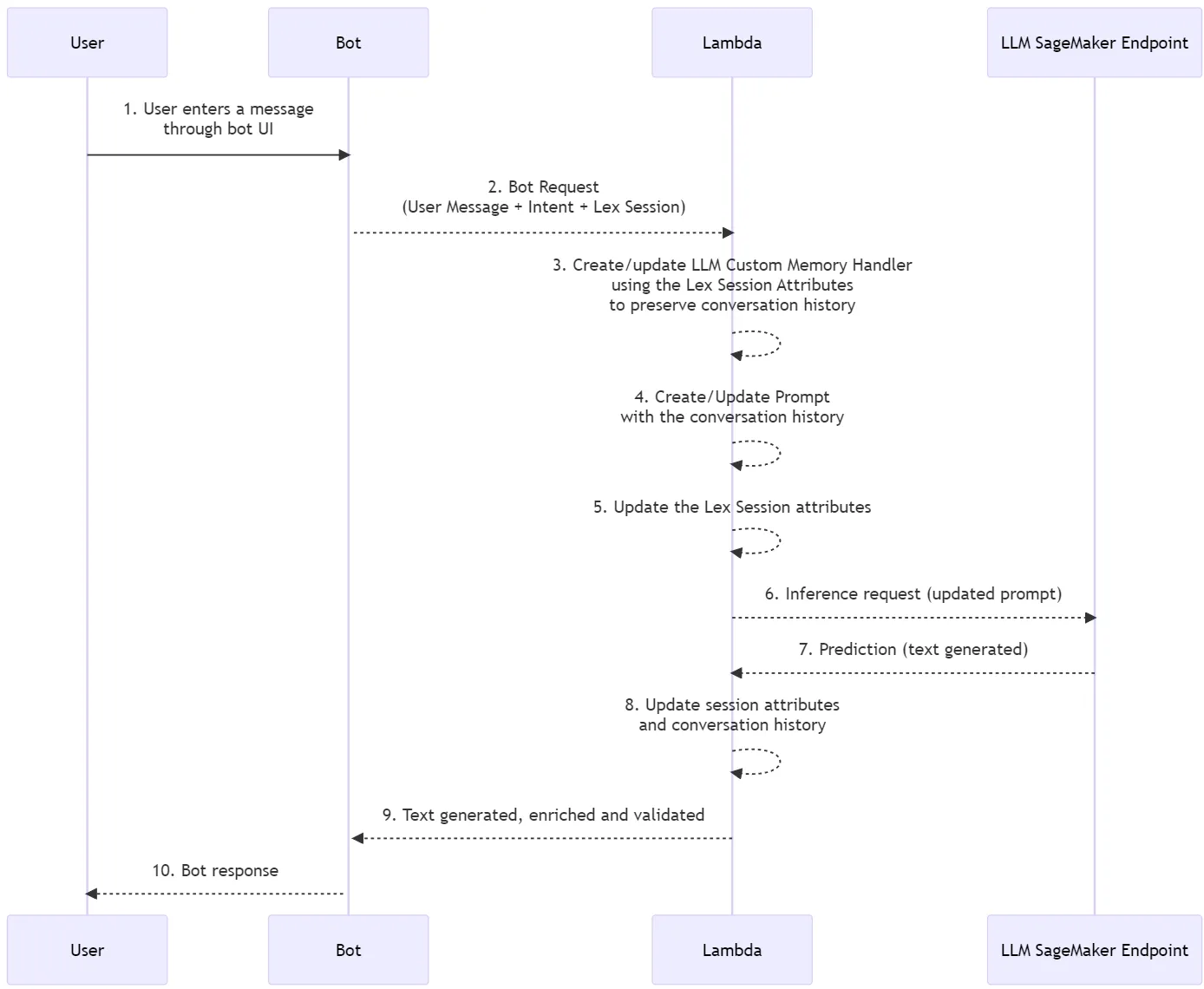
Fun fact: An earlier version was known as YEAH! (Your Excellent Artificial Helper) but it wasn't so well received during private showings.
- Takes in a user prompt
- Translates it to a target language using Amazon Translate
- Sends it to Anthropic's Claude on Amazon Bedrock
- Translates the response back to the source language
- Turns the response into speech via Amazon Polly
1
2
3
4
5
6
7
8
9
10
11
12
13
14
15
16
17
18
19
%% Use the mermaid-cli
%% > mmdc -i meh.mmd -o meh.png -t forest -b transparent
%% or the online version
%% https://mermaid.live
%% to create the diagram
sequenceDiagram
You 😒 ->> +MEH! 🐑 : Prompt ✍️
MEH! 🐑 ->> +Amazon Translate : translate_text 🌐
Amazon Translate ->> MEH! 🐑 :
MEH! 🐑 ->> You 😒 : Translated Prompt
MEH! 🐑 ->> Amazon Bedrock: invoke_model 🧠
Amazon Bedrock ->> MEH! 🐑 :
MEH! 🐑 ->> You 😒 : Response
MEH! 🐑 ->> +Amazon Translate : translate_text 🌐
Amazon Translate ->> MEH! 🐑 :
MEH! 🐑 ->> You 😒 : Translated Response
MEH! 🐑 ->> Amazon Polly : synthesize_speech 🦜
Amazon Polly ->> MEH! 🐑 :
MEH! 🐑 ->> You 😒 : Play 🔊
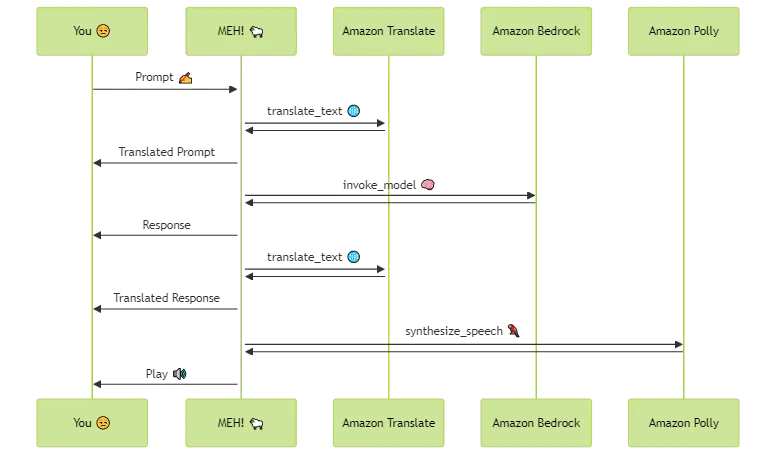
💡 This app uses Boto3, the AWS SDK for Python, to call AWS services. You must configure both AWS credentials and an AWS Region in order to make requests. For information on how to do this, see AWS Boto3 documentation (Developer Guide > Credentials).
1
streamlit run meh.py
1
docker build --rm -t meh .
1
docker run --rm --device /dev/snd -p 8501:8501 meh
1
wsl docker run --rm -e PULSE_SERVER=/mnt/wslg/PulseServer -v /mnt/wslg/:/mnt/wslg/ -p 8501:8501 meh
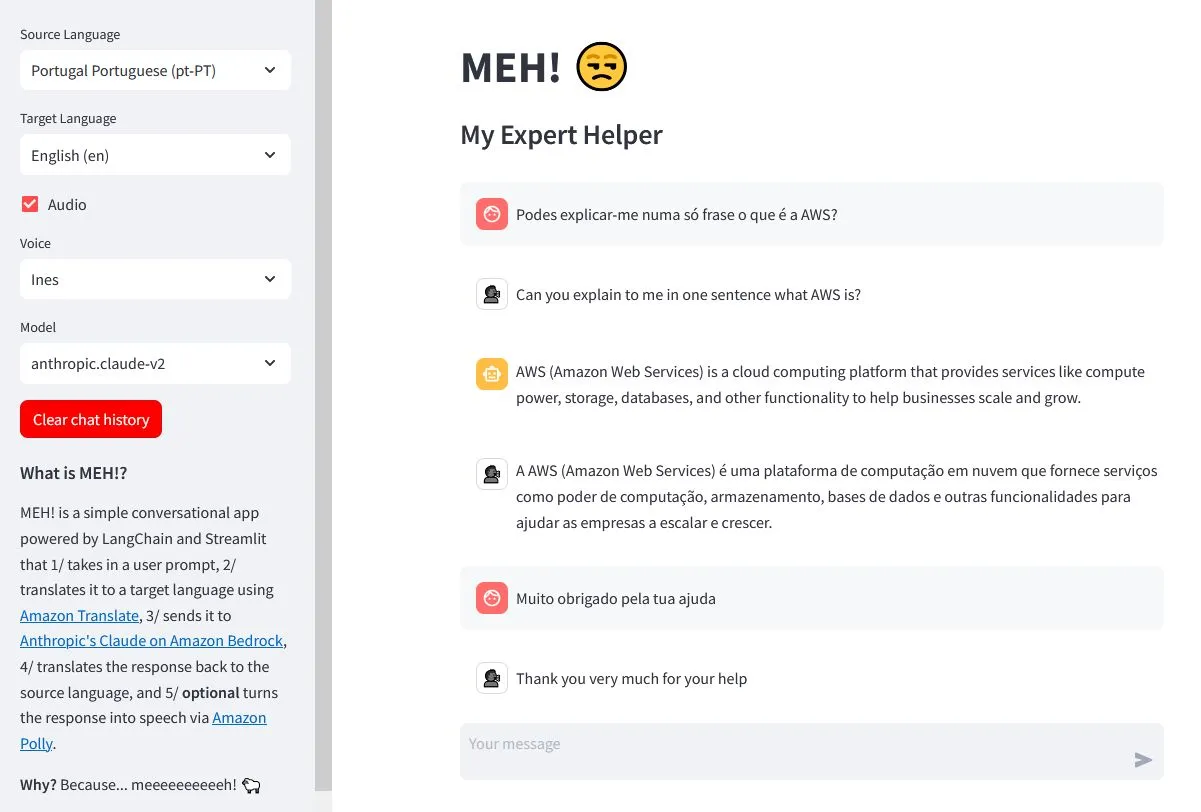
This is the second article in the #TGIFun🎈 series, a personal space where I'll be sharing some small, hobby-oriented projects with a wide variety of applications. As the name suggests, new articles come out on Friday. // PS: If you like this format, don't forget to give it a thumbs up 👍 As always: work hard, have fun, make history!
- JGalego/MEH: A simple conversational app powered by LangChain and Streamlit, feat. Amazon Translate, Amazon Bedrock and Amazon Polly
- aws-samples/conversational-ai-llms-with-amazon-lex-and-sagemaker: Getting Started on Generative AI with Conversational AI and Large Language Models
Any opinions in this post are those of the individual author and may not reflect the opinions of AWS.