Simplify RAG applications with MongoDB Atlas and Amazon Bedrock
Integrate MongoDB Atlas as the vector store and setup the entire workflow for your RAG application
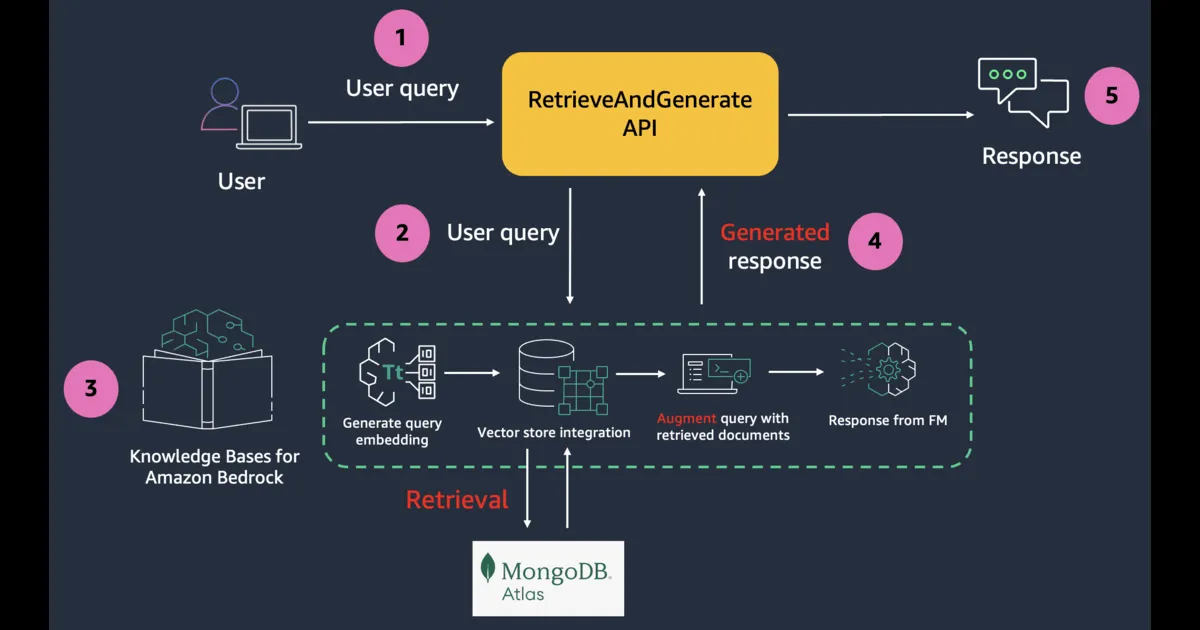
- This integration requires an Atlas cluster tier of at least M10. During cluster creation, choose an M10 dedicated cluster tier.
- Create a database and collection.
- For authentication, create a database user. Select Password as the Authentication Method. Grant the Read and write to any database role to the user.
- Modify the IP Access List – add IP address
0.0.0.0/0
to allow access from anywhere. For production deployments, AWS PrivateLink is the recommended way to have Amazon Bedrock establish a secure connection to your MongoDB Atlas cluster.
AMAZON_BEDROCK_TEXT_CHUNK
– Contains the raw text for each data chunk. We are usingcosine
similarity and embeddings of size1536
(we will choose the embedding model accordingly - in the the upcoming steps).AMAZON_BEDROCK_CHUNK_VECTOR
– Contains the vector embedding for the data chunk.AMAZON_BEDROCK_METADATA
– Contains additional data for source attribution and rich query capabilities.
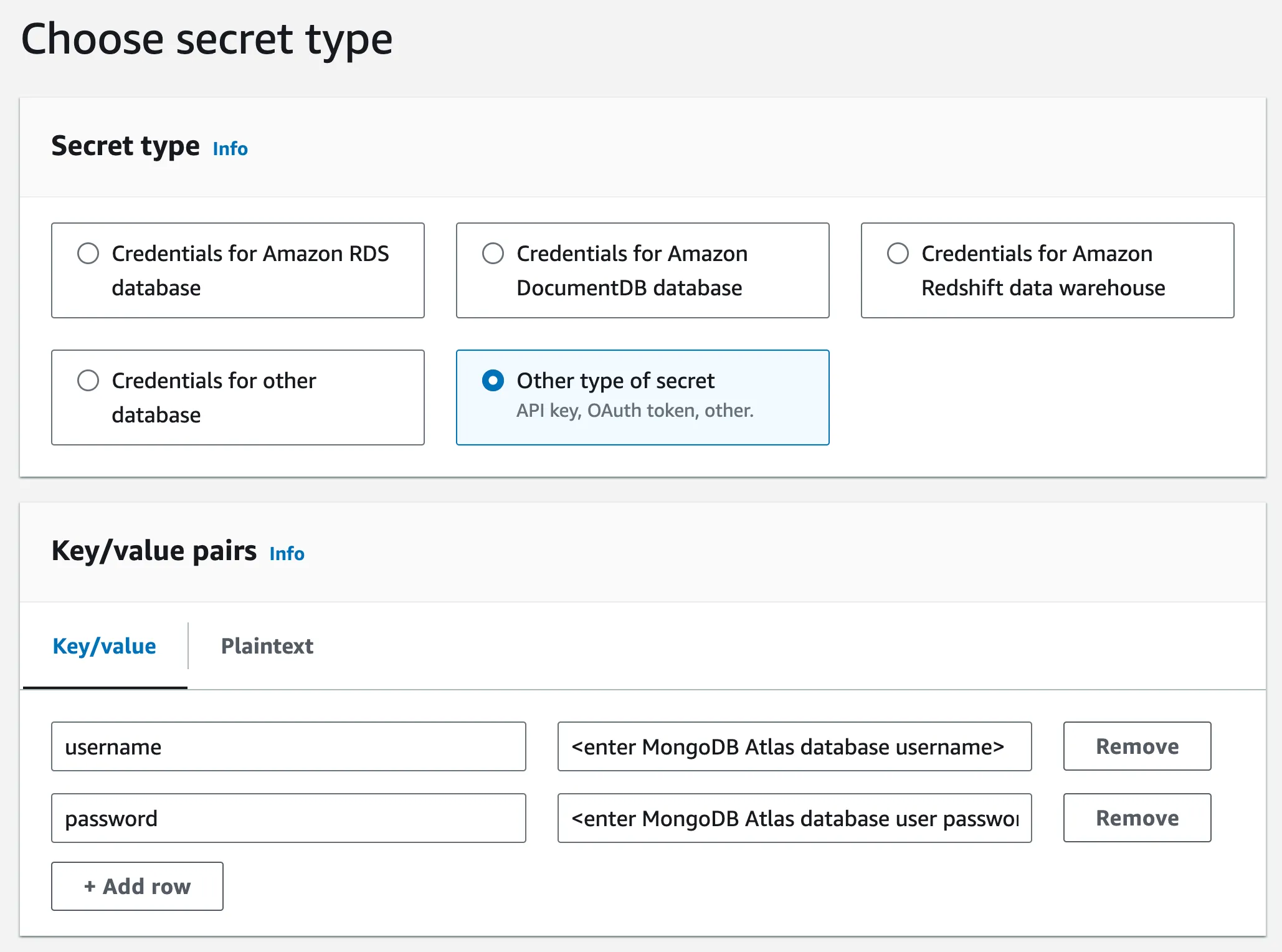
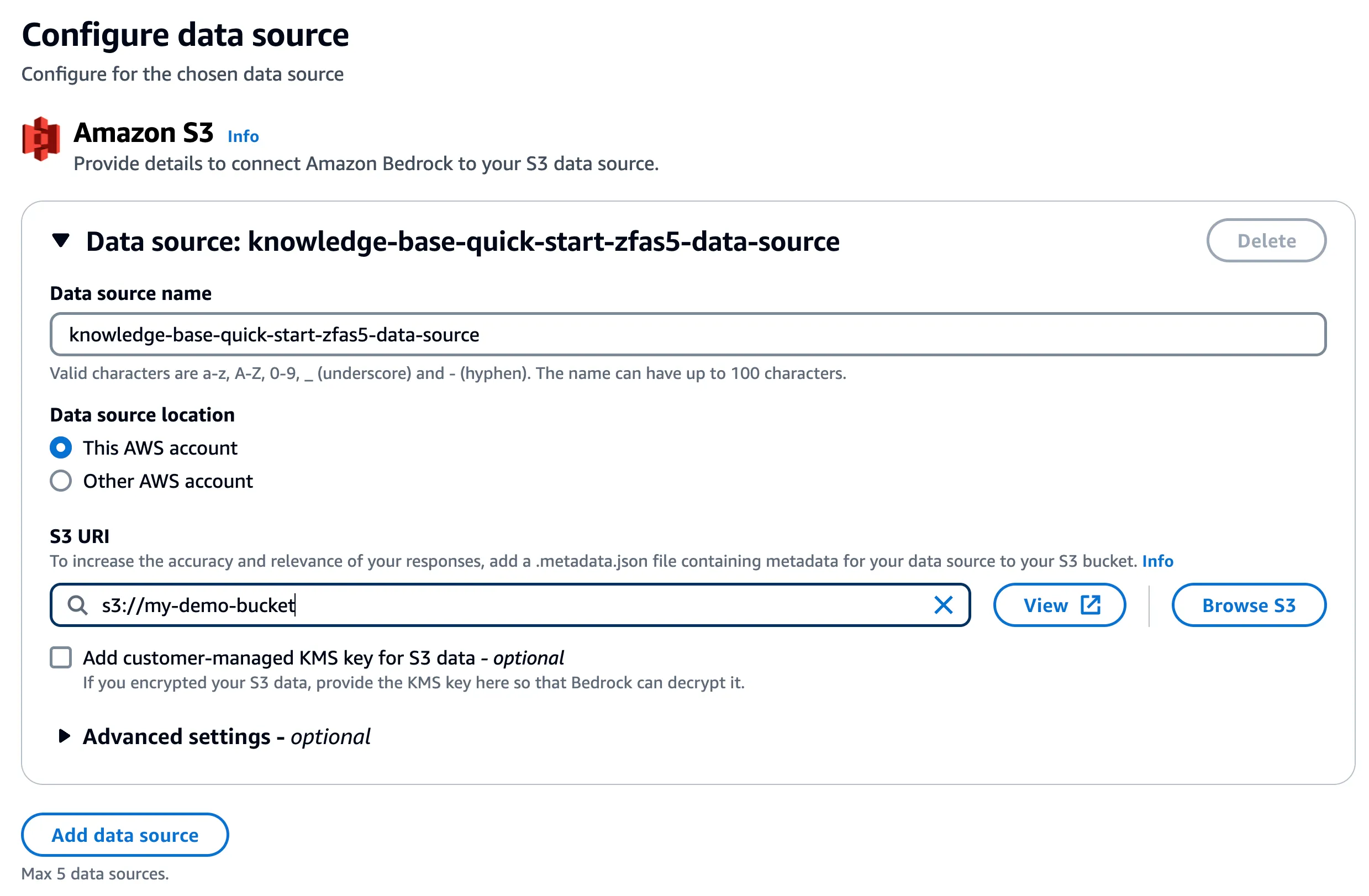
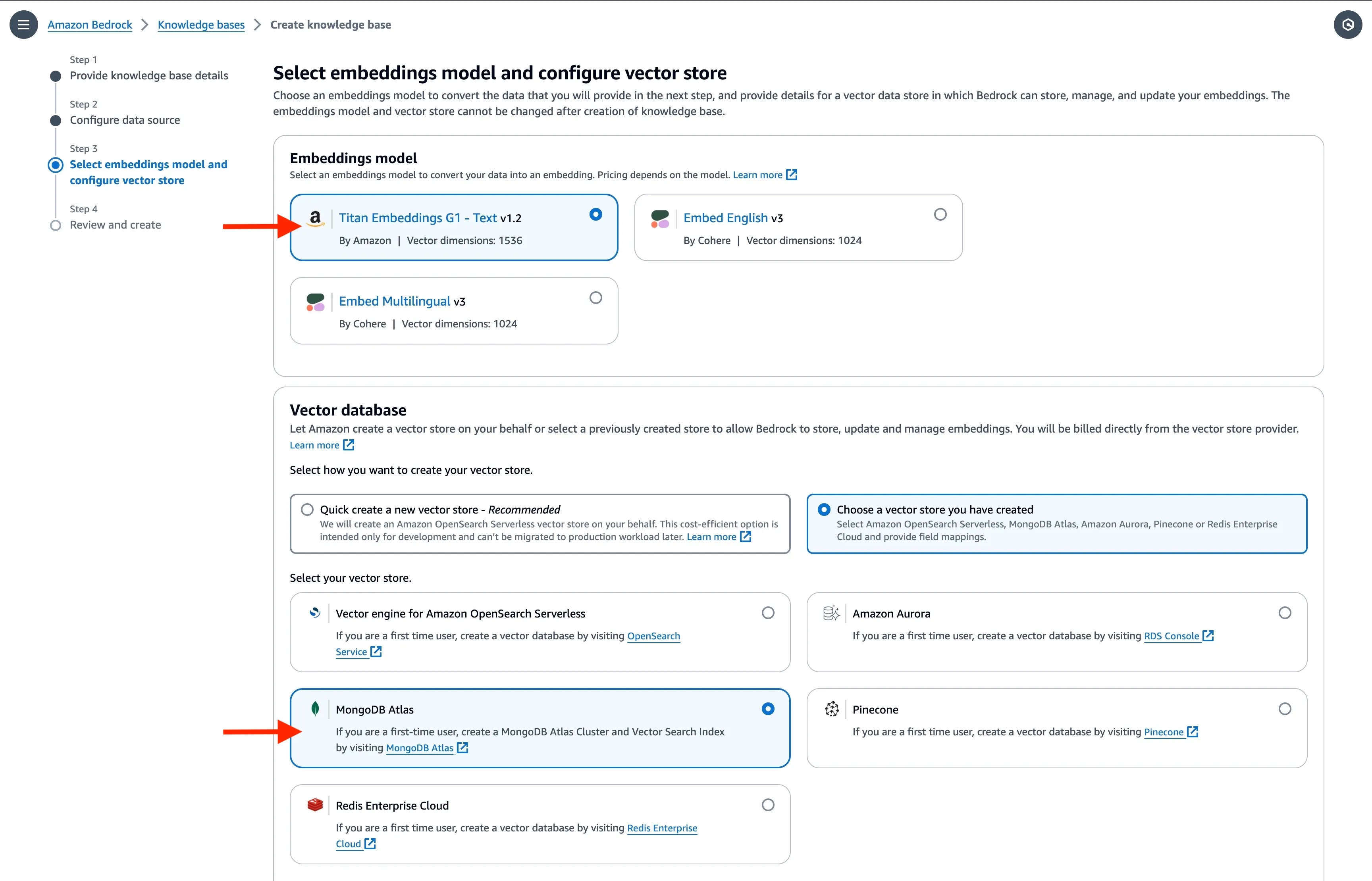
ARN
of the AWS Secrets Manager secret you had created earlier. In the Metadata field mapping attributes, enter the vector store specific details. They should match the vector search index definition you used earlier.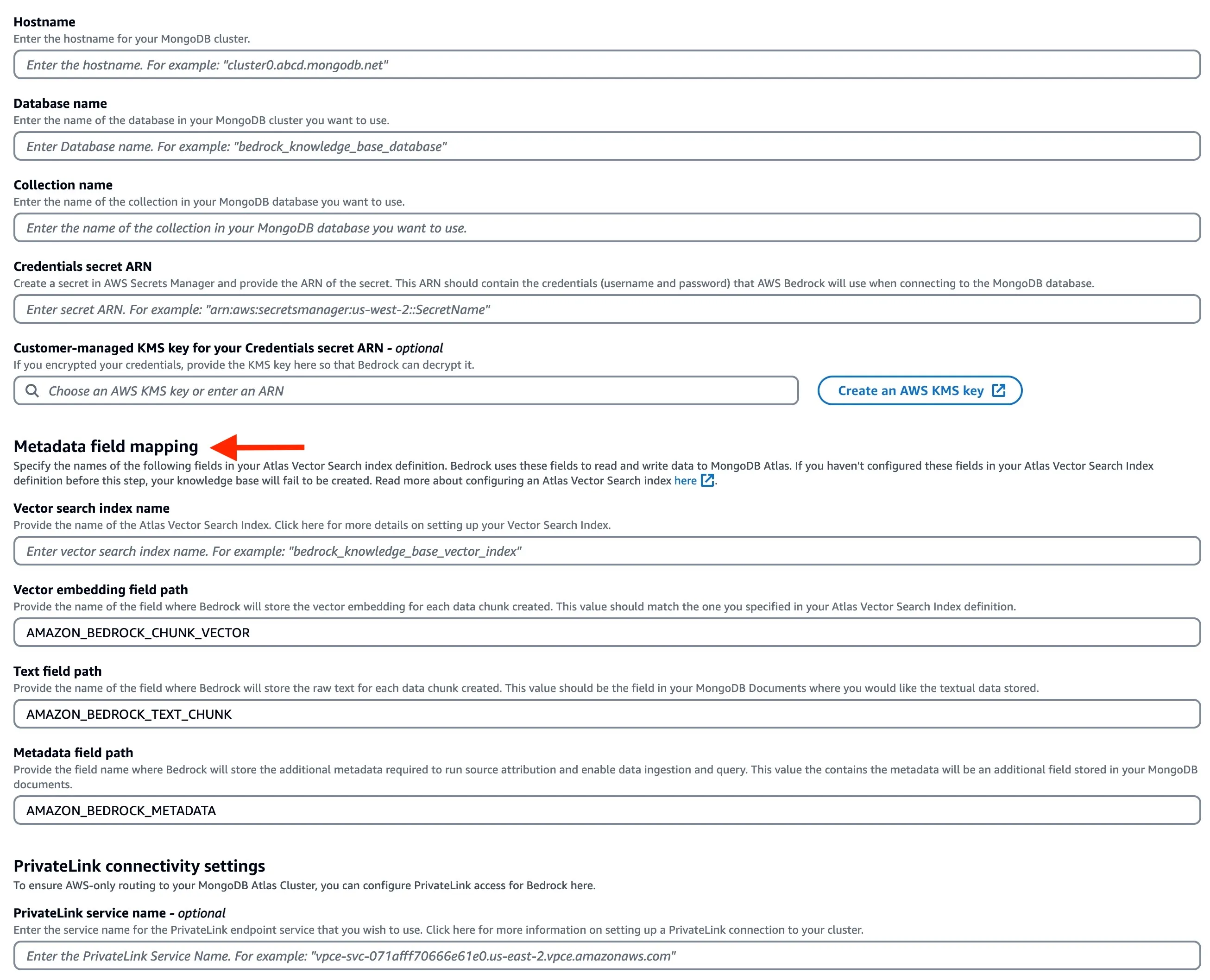

AMAZON_BEDROCK_CHUNK_VECTOR
along with the text chunk and metadata in AMAZON_BEDROCK_TEXT_CHUNK
and AMAZON_BEDROCK_METADATA
, respectively.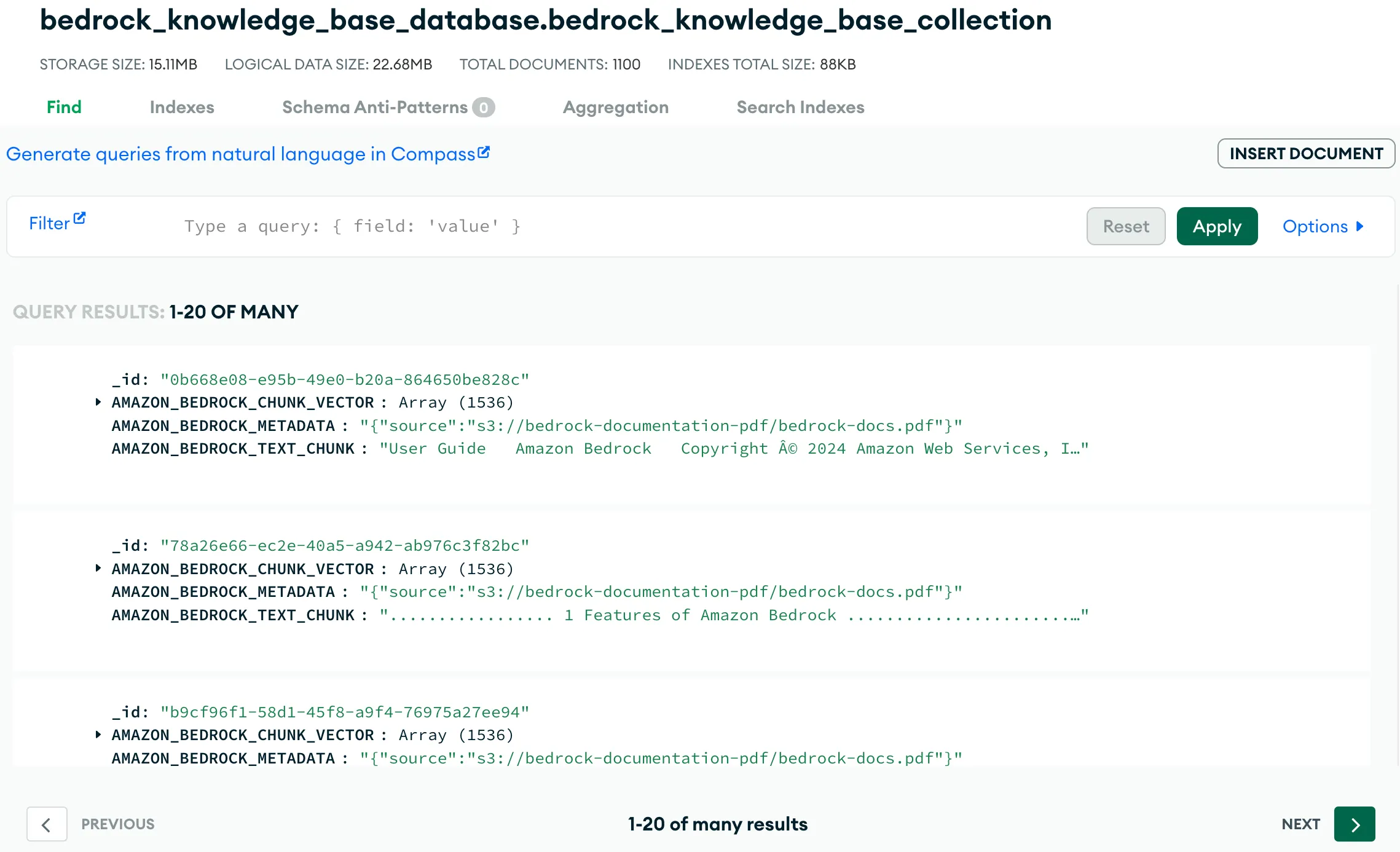
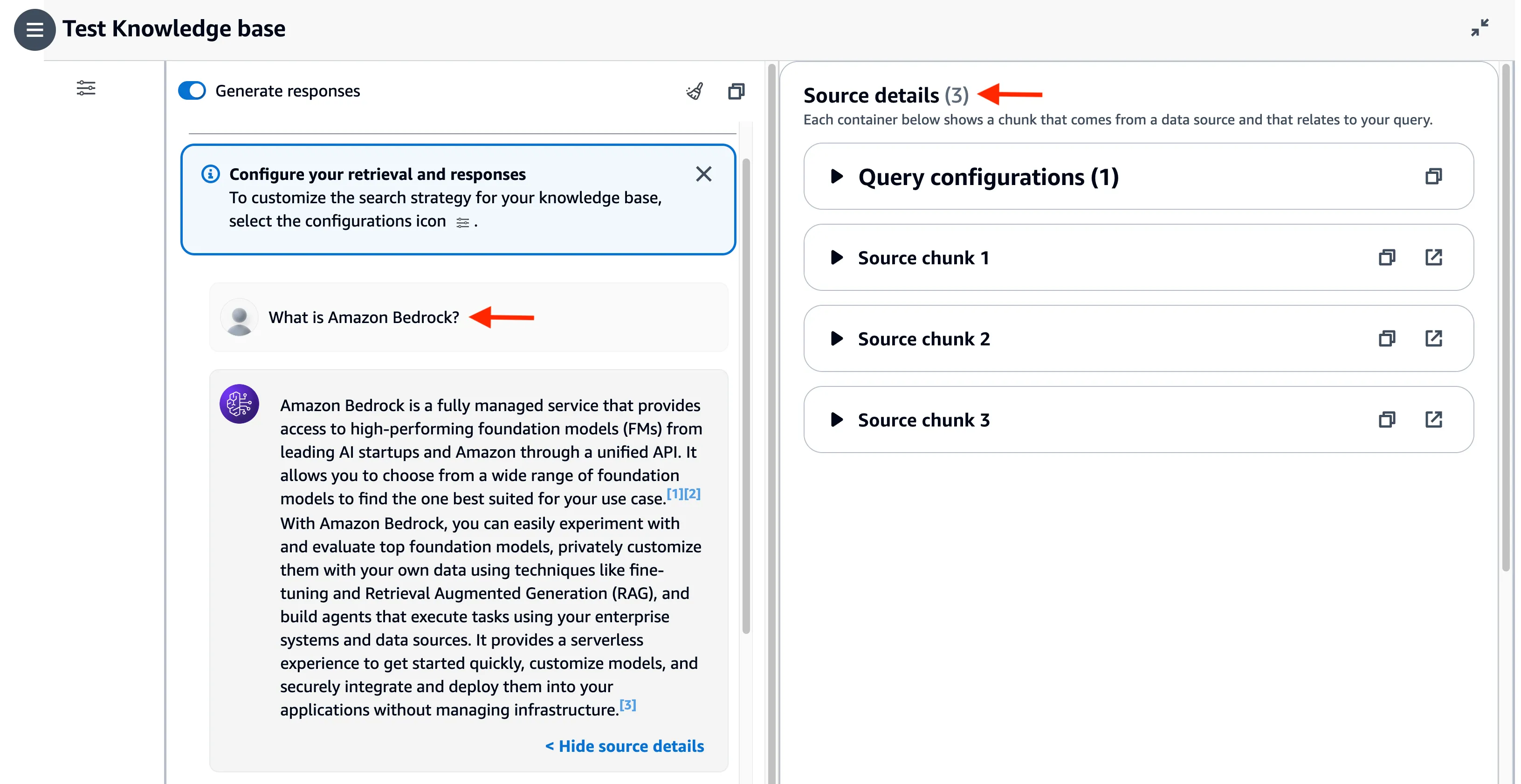
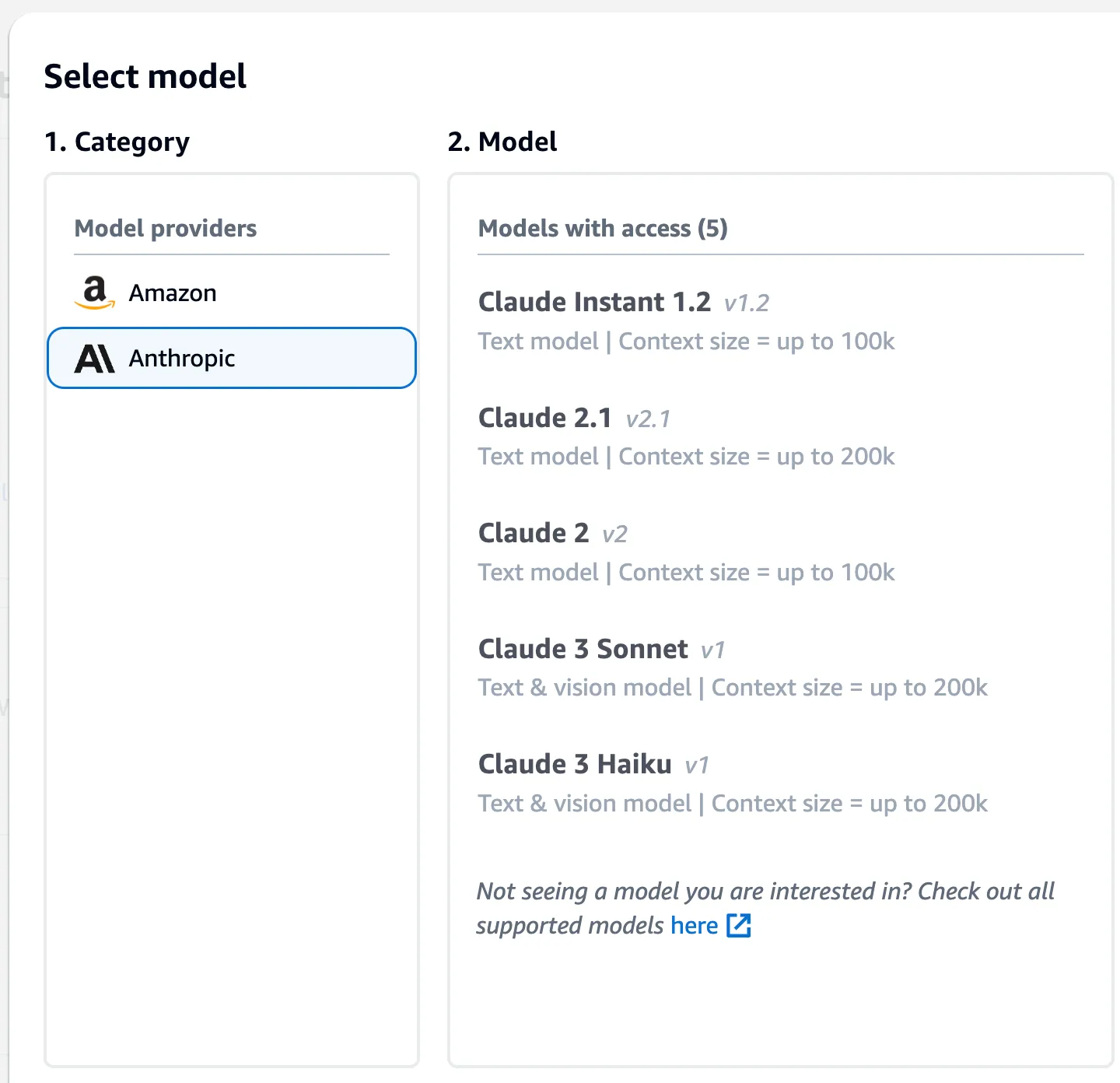
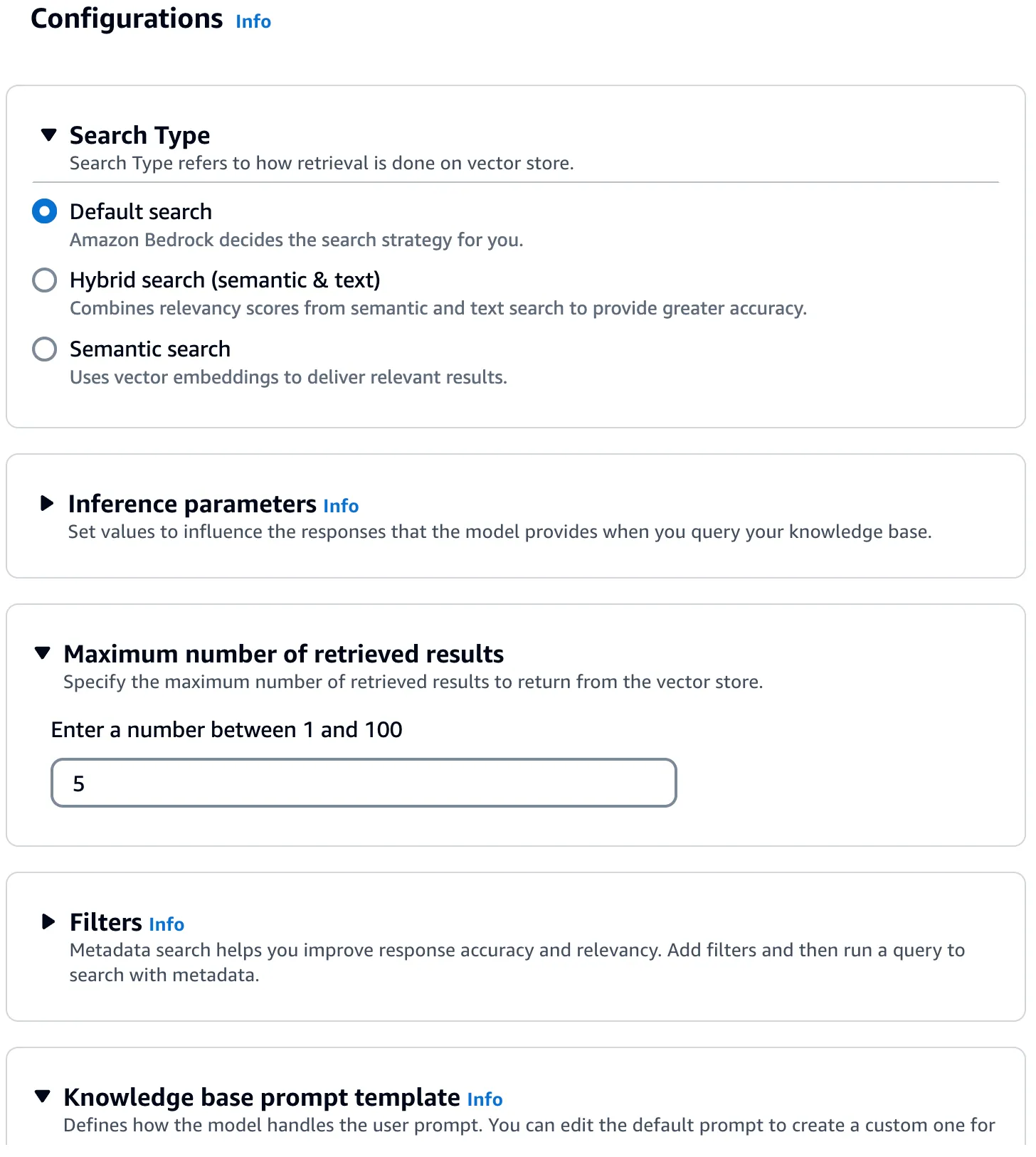
Any opinions in this post are those of the individual author and may not reflect the opinions of AWS.