![Building a Serverless Web Scraper with a ~little~ lot of help from Amazon Q [Part 1]](https://assets.community.aws/a/2hsVDf8pQVJYkIriTkB09iIyVaW/hero.webp)
Building a Serverless Web Scraper with a ~little~ lot of help from Amazon Q [Part 1]
I moved to Seattle at the end of 2021 on an L1b visa, and need to keep an eye on the priority dates for my Green Card application, so I decided to build an app that will pull the historic data for me and graph it.
Cobus Bernard
Amazon Employee
Published Jul 1, 2024
Update: 2024-06-14: This turned out to be more than I initially though, so will be turned into a series of articles, this being the first. I'll be posting the followups in this series, and link them as a list.
I've been itching to build a little serverless API for a while now, so I decided to use Amazon Q Developer to help decide how to build it. I didn't feel like building yet another Todo or note taking app, so I picked a practical problem I currently have: US Green Card priority dates. Since I moved to the US at the end of 2021, I've been going through the process to apply for a Green Card. An important aspect of this is your "priority date". The process is a convoluted one, the short summary is that each month, they publish the dates per visa category. While the latest date is useful, I wanted to look at the historic data as the bulletins go back to 2002. There are many factors influencing how these dates progress, so I'll be looking at the last 5 years (at least, that's my plan right now).
The idea is to write an API that pulls the values from all the bulletins, and then add it to a database table so I can query it. I'll be using Amazon Q Developer to help me along the way - both for asking general questions, and to help generate my code. So let's get started. I'm going to try my best to follow the instructions as-is, and only make small changes to see how far I can get.
All the code for this app can be found on GitHub, with the code as it was at the end of this article on the article-1 tag.
For each Visa Bulletin, there are 2 tables that I need to keep an eye on, the TL;DR: version is there are 2 important tables under “final action date” and “filing date”, and only 1 of these is applicable at a given time for my category. At the moment, it is the “final action date” one, and the table looks like this for the July 2024 bulletin:
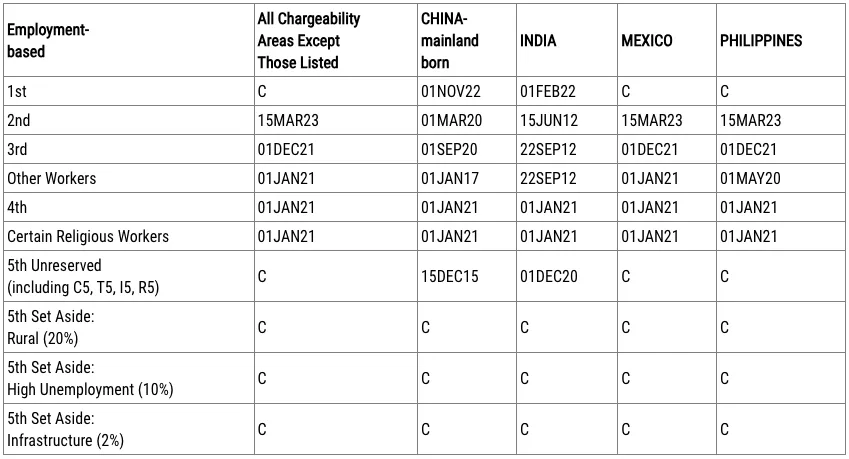
The first step is to decide how I want to build this. I want to start off using AWS Lambda functions, DynamoDB, Amazon S3, and then Terraform to create all the infrastructure, and then deploy my function(s). I’m expecting a lot of back and forth questions with Amazon Q Developer, so I’ll add all the prompts/responses and my comments about them at the bottom of this page. I’m not sure which programming language to use yet, and will see what is suggested. Overall, it seem doable based on the first prompt’s response, so let’s start with the Terraform code.
Setting up Terraform for a new project always has a chicken & egg problem: you need some infrastructure to store you state file, and you need IaC to create your infrastructure. After looking at the answer, I create the following files to deal with it. I started using Terraform in ~2015 from v0.5, so I’m incorporating what I’ve learned using it in production, and will do the same for all the responses from Amazon Q Developer throughout this article.
You may have noticed the lack of any AWS credentials in the code above, and that is intentional. I know I will run into AWS credential issues when I try to run
terraform plan
- I specifically never have the default
profile configured due to the many different accounts I use. I would rather have an error that forces me to think where I want to run something than accidentally deploy / make any API calls to the incorrect account. I have set up a profile for this project called development
, so I export it via the AWS_PROFILE
environment variable by calling:Now I can run
terraform apply
to create those resources for the state file, uncomment the lines in statefile.tf
, and then run terraform init
to store the state file in S3.Important: You cannot use variables in theterraform
block for thebackend
, so make sure to copy the exact names for the resources from_bootstrap.tf
.
Ok, we can now start building the app.
I ask the following to help me get started:
Thanks, can you show me how I would write a webpage scraper with Lambda to scrape all the pages linked from https://travel.state.gov/content/travel/en/legal/visa-law0/visa-bulletin.html with the format https://travel.state.gov/content/travel/en/legal/visa-law0/visa-bulletin/2024/visa-bulletin-for-july-2024.html - they should be linked from the first page listed. Each page has multiple sections and tables, I would like to only store the rows in the tables listed under the heading “A. FINAL ACTION DATES FOR EMPLOYMENT-BASED PREFERENCE CASES” and “B. DATES FOR FILING OF EMPLOYMENT-BASED VISA APPLICATIONS”. Can you show me how to create a Lambda function to do this and store the data in DynamoDB?
The provided code needs a few changes, and I now have this:
I do see that it needs a DynamoDB table, so I added it after asking for the Terraform code - I will add everything for the application to
app.tf
for now, may split it out later, here is the table definition so far (foreshadowing… :) ):Next up, I want to ensure we can deploy the application. There are may different ways to accomplish this, and since I’m already using Terraform for the rest of the infrastructure, I want to use it to zip up the file and deploy it as a Lambda function as well. The first attempt is close, but requires me to manually install the dependencies and zip up the code, so I try again, being more specific. While this attempt looks closer to what I need, I can see it won’t work due to how
requirements.txt
is handled. I find this article and like the approach more as part of the 3rd attempt:- Install the dependencies in
requirements.txt
- Zip these up
- Deploy it as a Lambda layer
This means that my build will only update this layer if my dependencies in
requirements.txt
change. I also move handler.py
into /src
, add src/package
and layer
to my .gitignore
. Now I need to make sure that requirements.txt
have the correct imports - I’ve used python enough to know about venv
to install dependencies, but haven’t had to do it from scratch before. After poking around for a while, learn that the “easiest” way would be look at the import
statements at the top of my code, and then call pip3 install <module>
for each one, currently I have requests
, boto3
, and BeautifulSoup
, and then run pip3 freeze > requirements.txt
. This does highlight a problem in my current approach: I’ve been merrily setting up all the infrastructure with Terraform, but not run my code at all. Once I have the infrastructure creation working, I will then focus on making sure the code works. I end up with the following Terraform in app.tf
:Looking at this code, I do see a problem for later: the
dynamodb:PutItem
Action will only allow my Lambda to write to the table, but not read. I’m going to leave it here for now till we get there. On the first terraform plan
, I ran into the following error, and had to run terraform init -upgrade
to install the null
and archive
providers:I now have my Lambda function and dependencies deployed, but no idea yet if it works, so I look how I can run it locally and create
src/local_test.py
:I can now run this by calling
python3 local_test.py
, and it will be able to access my AWS resources we exported the environment variable AWS_PROFILE
. And it looks like it works since I don’t see any errors:Time to add some outputs to my code so I can see what is happening - I’m not going to try to implement proper logging at this point, will leave that and all the other productionizing for a future date. I add in a
print
line in the scrape_visa_bulletin
function:When I now run
local_text.py
, I can see that it is processing all the bulletins, but there is no data in my DynamoDB table:Looking at the page source for one of the bulletins, I can see that there aren’t any
<div>
fields that match field-item even
for the class
as defined in this line in my app:After trying again, and a few more times, I realise that I’m not able to clearly articulate exactly how I want to extract the data, so instead of continuing, I just take a somewhat brute-force approach and loop over the
tables
, rows
, and cells
to inspect the data. The code afterwards for scrape_visa_bulletin
now looks like this (and it isn’t storing the data in the DB yet):It does however prompt me with some autocomplete suggestions that I use and the modify a bit more - below you can see what it looks like, the grayed out text is the suggestion:
Using the URL format of
visa-bulletin-for-july-2024.html
to determine the date of the bulletin, I extract that, and store it in the bulletin_date
. The debug output from the print
line after I add the data to employment_based_data
looks mostly right to me at this point:Now that I have the raw data, storing it in DynamoDB is the next step. The shape of the data is not what I want to store, currently it is an object per table cell. Ideally I want the data in the format:
The response looks ok, but I am by no means proficient in python so let’s copy & paste and use it, here is what was added:
The last step is to add
transformed_data = transform_data(data)
to the lambda_handler
. All good so far, except when I run the code, it returns None
for transformed_data
. After wrapping the code in try
blocks, I still can’t see the issue, and even with some more help, it stays None
. I even try json-serializing the objects to better inspect them, which results in me learning about custom serialization in Python via:With a bunch of
print
statements and json.dumps
later, I still see the correct data in grouped_data
and transformed_data
(inside the transform_data
method though). Then it hits me: if I’m calling a method and using the result of it, that method should probably have a return
call in it… Right, let’s pretend that didn’t happen and I had return transformed_data
in there the whole time… Ahem!Since we started on this merry adventure, I didn’t really think about the shape of the data and how I want to store it, I just went with the recommendation from Amazon Q Developer. Now that we have the data in the shape we need, let’s see what changes are needed. I need to update the DynamoDB table, and then also add in the logic to store the data, and then add a method to retrieve the specific data set I’m interested in. The table definition now is:
When I try to
terraform apply
this change, it returns the error write capacity must be > 0 when billing mode is PROVISIONED
. After looking at the dynamodb_table
resource definition, it appears you need to specify write_capacity
and read_capacity
for the global_secondary_index
as well. With that fixed, I try to run the “final” version for today’s efforts, but it errors with:Luckily my handing coding assistant is extremely polite, and instead of telling me “didn’t you pay attention to the code I gave you”, it tells me “The issue here is that the
Key
function is part of the boto3.dynamodb.conditions
module, and you need to import it explicitly in your Python script.”. Yes. This is me not paying attention to the output. After adding the import, the error is gone, but I do notice that after I changed the logic in lambda_handler
it errors.Changed from:
To:
There is an error “Unable to group the data, error: list indices must be integers or slices, not str”, and I remember seeing this as part of a previous response:
Note: This code assumes that theemployment_based_data
collection contains unique combinations offiling_type
,country
,category
, andbulletin_date
. If there are duplicate entries, you may need to modify the code to handle them appropriately (e.g., by keeping only the latest entry or aggregating the data in some way).
Which makes sense when I look at how I am grouping the data:
After checking again and again I find the error:
The returned object from
scrape_visa_bulletin
is a list, not a single object, so using .append()
causes the issue as it will add that whole list as a single object in my new list. Instead, I need to use .extend()
. While debugging this and looking at how the data is stored, I also realise that we don’t need the transform_data
at all, if you look at what is being stored via the table.put_item
, the flat list of objects we extract from the web pages would work:So all I would need to store the data is:
Realisation #3: so far, I have only approached this as a single run, if I were to run the code a 2nd time, it would add the full history a 2nd time. So let’s fix this by adding another DynamoDB table called
ProcessedURLs
and updating the code by adding processed_urls_table = dynamodb.Table('ProcessedURLs')
at the start of handler.py
under our existing reference to VisaBulletinData
, and then update lambda_handler
to:Now the only step left is to update the IAM policy to allow read and write access to this new table. Remember earlier in this article where I mentioned we will need to also add read permission to the
VisaBulletinData
table? Let’s combine that into a single request! At first glance, I’m confused why it created 2 statements instead of adding the 2 resources as an array in the Resources:
section. Looking a 2nd time, I spot the difference in the statement Action
sections:When we call the
VisaBulletinData
table to read from it, we are not using a .get()
call, but a .query()
call. I would have missed that 😳 … Nice to see that someone else is at least paying attention.Once all these changes were in place, I ran the app again locally to process all the bulletins for 2022 - 2024. While I was building so far, I had limited it to only the most recent one (July 2024). The first few pages it processed scrolled past very quickly, and the it just hung in my terminal. No error. And I realised what the issue was:
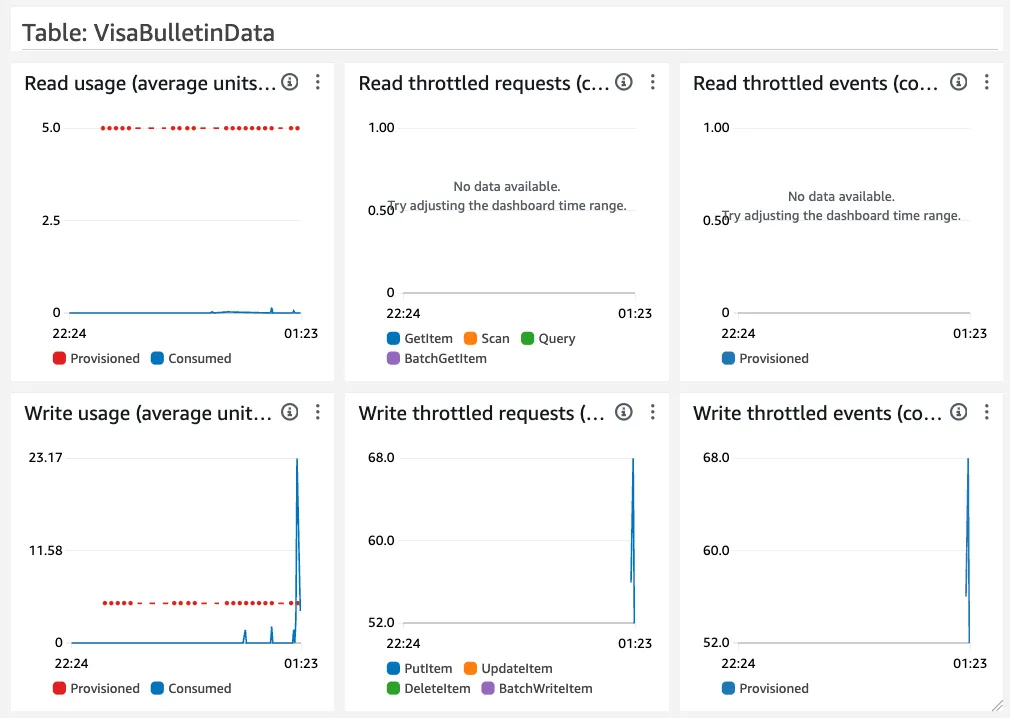
The way I’m processing and storing the data results in more calls than the 5 read / write units I had provisioned for my DynamoDB table. I called it a day, and when I came back this morning, the process had finished without breaking. At least without any exceptions, my table design needs to be completely redone though. From the code further up, this is how I was storing my data, see if you can spot the issue:
I’ll give you a hint: using the above, my primary key (pk) for the data I’m interested in would be
Final Date#3rd
, and it can only store 1 entry per country
with 1 bulletin_date
and date
stored: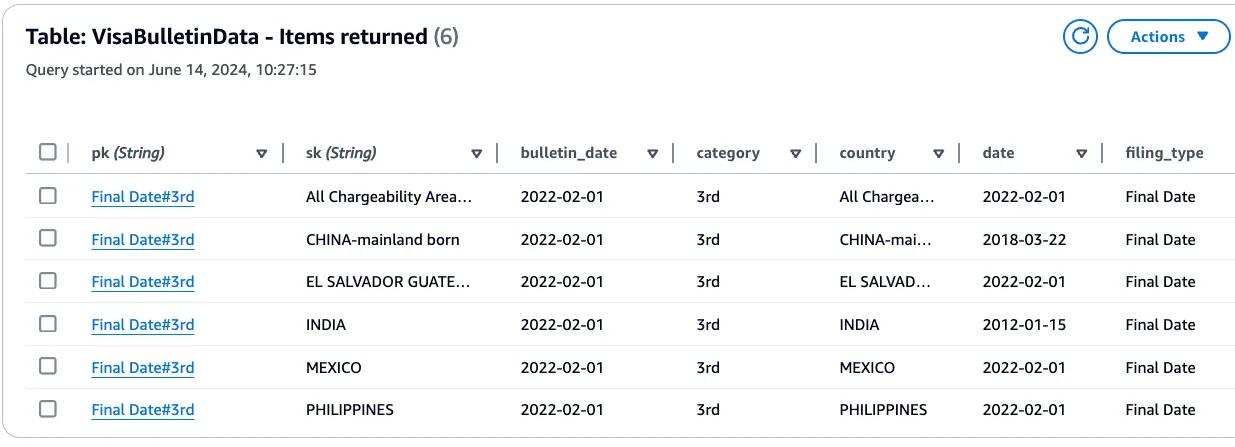
At this point, my todo list has grown to:
- Fix the data structure to store all the data
- Test the Lambda function to see if it works
- Add parameters to the function to be able to only select the specific set of dates you want
- Decide how to expose the Lambda function - either using AWS API Gateway as I have before, or setting up an invocation URL
- Set up a CI/CD pipeline to deploy it
- Add in tests
This would make this article way too long, so my plan now is to update the code to take the data I’ve retrieved, pull the subset I need, and not try to store it in DynamoDB. Then I can tackle that and the other items in follow up pieces.
Changing the code to use the collection of data I already have results in the following:
I’m setting some defaults for now as I’m very keen to see if it works. And it does! Yay!!!! Here is the output from that
print
statement:I’m going to pause at this point, and continue in the next article.
This was actually a lot of fun, I love trying out something I have no idea how to do, making mistakes along the way, and learning. One of my favourite interview questions, after asking what projects a person has worked on, is to ask them “How did it break / go wrong, and how did you go about fixing it”. I’ve found that if a person can’t answer that, they were either not that involved, or didn’t really build it. But that is a post for another time.
From this little adventure, I would summarise what I learnt into the following sections.
I’ve been using Amazon Q Developer for a few months now while building an internal reporting tool in C# (my primary coding language at the moment), and this was definitely a very different experience. I’ve used Python quite a few times over the last 19 years I’ve been building full time, but never from scratch, or with AWS services other than single-purpose, one-off Lambda functions. And never deployed it with Terraform. Getting
requirements.txt
(correctly) populated took me longer than I expected. It was definitely a lot faster and easier with Amazon Q Developer than with my old approach of googling things piecemeal. If I had to summarise it, the conversational aspect along with not needing to sit and think exactly what keywords to add, makes a huge difference. Usually I would open multiple of the search results that are kind-of-but-not-quite what I’m looking for, spend the first 10 seconds to assess the source by scrolling the page, looking at when it was published, and if it feels trust-worthy. Then I would need to string together multiple parts from different sources unless I was really lucky and found exactly what I was looking for.As for not even having to switch to my browser, that is probably the biggest win. I’ve found myself even using it for non-coding questions, e.g. the difference between “learnt” and “learned”. While it makes me much more productive, that doesn’t mean I can copy and paste everything. Large language models (LLMs) are still improving every day, and while I didn’t run into any hallucinations in this exercise, I did have to evaluate each response for accuracy, and if it was the way I should be solving the problem. Just look at the part where I built that whole
transform_data
method only to realise I didn’t need it all. All my experience over the last 32 years (GET OFF MY LAWN YA KIDS, I ENJOYED CHANGING DOS 6.22 MENUS!!!) is still very relevant.One pain point I had was when I accidentally closed my IDE, and with that, lost the conversation thread I had going. I’ll definitely be passing on that feedback to our service teams, and also seeing if it doesn’t keep a local log somewhere. If anyone knows, please drop that in a comment below please.
This was probably the biggest one for me. My background the last 10+ years has been very / mostly DevOps focussed, so I approached this by starting with the infrastructure automation, how I would use a Lambda function to store my data in DynamoDB. Instead, I should have started with just the Python code to retrieve and process the data, then turn that into a Lambda function, deploy it, etc etc.
If I wanted to just get this done, I would have used C#, but I wanted to play around and spend some time learning. Amazon Q Developer suggested using Python, so I went with it, and it definitely stretched my brain to use a dynamic language. Looking back at the first prompt, I thought I would be using Bedrock for this, but I didn’t really think it through, and even though it was included it in the list (and even called it a “core service you’ll use to build your generative AI application”), I didn’t end up using it at all. At least, not yet ;)
The most interesting part for me was the 💡 moment regarding Lambda Layers. While I have known about them for a while, as well as how they worked, it had yet to fully “click” in my brain. Using a layer for all the dependencies that
pip
installs makes complete sense to me, and this is a really good way to split deploying changes between the application and the dependencies. I can see some interesting ways how you could use this test out upgrading the dependency version and then testing them before you roll it out to production…While doing the Terraform code, I quickly looked at the difference between
path.cdw
and path.module
, and I will need to read up more. Most of the time, I’ve seen path.module
used, but it looks like path.cwd
should be used instead.As this is going to turn into a series of articles, I’ve tagged the code at this point in time, you can have a look at the source for what was covered in this article and is “working”, I’ll include instructions in the
README.md
on how to run it locally.To wrap things up, I would say this was a (mostly) successful attempt to solve what I trying to do, with a number of detours. I’m going to start working on the next part later today, I really want to get to a point where I can just call an URL to get the information instead of finding the pinned tab in my browser, and refreshing it daily to see if there are any updates. As the next articles come out, I’ll add links to them below.
And yes, I am 100% aware that I could just have copied the dates into a spreadsheet by hand and slapped on a graph in about 1h, but what fun would that be? I’ll leave you with two of my favourite pieces of advice from xkcd.
I want to build an app using Amazon Bedrock that can pull in data from a public website using Terraform to build the infrastructure, can you start by telling me if this is possible, and which services I will need to use?
Response:
Yes, it is possible to build an application using Amazon Bedrock that can pull data from a public website, and you can use Terraform to provision the necessary infrastructure. Here’s an overview of the services you might need to use:
- Amazon Bedrock: This is the core service you’ll use to build your generative AI application. With Bedrock, you can choose from various foundation models (FMs) like Anthropic’s Claude, AI21 Labs’ Jurassic-2, and Amazon’s Titan models. You can also create Knowledge Bases and Agents to integrate external data sources and execute complex tasks. [1]
- AWS Lambda: You can use AWS Lambda functions to fetch data from public websites and integrate with Amazon Bedrock. Lambda functions can be triggered by various events, such as API Gateway requests or scheduled events.
- Amazon API Gateway: If you want to expose your application as an API, you can use API Gateway to create, publish, and manage APIs that can invoke your Lambda functions.
- Amazon S3: You might need to store the data fetched from public websites in Amazon S3 buckets, which can then be used by your Bedrock Knowledge Bases or Agents.
- AWS IAM: You’ll need to manage permissions and roles using AWS Identity and Access Management (IAM) to allow your Lambda functions and Bedrock resources to access other AWS services securely.
- Terraform: You can use Terraform to define and provision the necessary AWS resources, such as Lambda functions, API Gateway, S3 buckets, IAM roles, and Bedrock resources, in a declarative and reproducible manner.
To get started, you can create a Terraform configuration that provisions the required resources, such as Lambda functions to fetch data from public websites, S3 buckets to store the data, and Bedrock resources like Knowledge Bases and Agents to integrate the data and build your application logic.
You can also explore the AWS Bedrock Studio, a web-based development environment that simplifies the process of building generative AI applications using Bedrock’s capabilities.
Sources:
This is mostly ok, except I prefer not to create the infrastructure to store the statefile by hand, so I usually have_bootstrap.tf
that contains the details of my base infrastructure for Terraform, create the S3 bucket, DynamoDB locking table, and KSM key with that, and then I add in the backend config to use those resources.
Thanks, let’s start by getting the Terraform set up storing the statefile in an S3 bucket
Response:
Sure, let’s start by setting up Terraform to store the state file in an Amazon S3 bucket. This is a recommended practice for managing Terraform state in a team or CI/CD environment, as it provides a centralized and durable location for the state file. [1]
Here are the steps to set up an S3 bucket for storing the Terraform state file: [2]
- Create an S3 bucket: First, you’ll need to create an S3 bucket to store the state file. You can do this using the AWS Management Console, AWS CLI, or Terraform itself. Make sure to choose a unique bucket name and configure the appropriate access controls and versioning settings.
- Configure the Terraform backend: In your Terraform configuration, you’ll need to specify the S3 backend for storing the state file. Create a
backend.tf
file with the following content:
Replace the placeholders with your actual S3 bucket name, the desired path and name for the state file, your AWS region, and an optional DynamoDB table name for state locking (recommended for team environments).
- Initialize the backend: Run
terraform init
to initialize the Terraform working directory and configure the S3 backend. - Configure AWS credentials: Terraform needs access to your AWS credentials to interact with the S3 bucket and other AWS resources. You can configure your AWS credentials using environment variables, shared credentials file, or other methods supported by the AWS provider.
- Apply the configuration: Run
terraform apply
to create the S3 bucket (if it doesn’t exist) and store the initial state file in the bucket.
After completing these steps, Terraform will store the state file in the specified S3 bucket and use it for subsequent operations. This ensures that your Terraform state is centralized, durable, and accessible to your team or CI/CD pipeline.
Remember to follow best practices for securing your S3 bucket and state file, such as enabling versioning, configuring appropriate bucket policies, and using server-side encryption.
Sources:
Thanks, can you show me how I would write a webpage scraper with Lambda to scrape all the pages linked from https://travel.state.gov/content/travel/en/legal/visa-law0/visa-bulletin.html with the format https://travel.state.gov/content/travel/en/legal/visa-law0/visa-bulletin/2024/visa-bulletin-for-july-2024.html - they should be linked from the first page listed. Each page has multiple sections and tables, I would like to only store the rows in the tables listed under the heading “A. FINAL ACTION DATES FOR EMPLOYMENT-BASED PREFERENCE CASES” and “B. DATES FOR FILING OF EMPLOYMENT-BASED VISA APPLICATIONS”. Can you show me how to create a Lambda function to do this and store the data in DynamoDB?
Response:
Sure, I can help you with that. Here’s an example of how you can create an AWS Lambda function in Python to scrape the pages you mentioned and store the relevant data in DynamoDB.
First, let’s install the required Python libraries:
Next, we’ll define a function to scrape the data from a given URL:
This function takes a URL as input, sends a GET request to fetch the page content, and then uses BeautifulSoup to parse the HTML. It finds the relevant tables by looking for
<div>
elements with the class field-item even
. For each table, it checks if the heading matches the desired sections (“FINAL ACTION DATES” or “DATES FOR FILING”). If so, it extracts the data from the table rows, skipping the header row, and appends it to a list.Now, let’s define the Lambda handler function:
In the
lambda_handler
function, we first fetch the base URL (https://travel.state.gov/content/travel/en/legal/visa-law0/visa-bulletin.html
) and find all the links to visa bulletin pages using BeautifulSoup. Then, for each visa bulletin page link, we call the scrape_visa_bulletin
function to extract the relevant data.For each item of data extracted, we construct a table name based on the current date and the table heading, and store the data in DynamoDB using the
put_item
method of the DynamoDB table resource.Finally, the function returns a success message with a status code of 200.
To deploy this Lambda function, you’ll need to create a DynamoDB table named
VisaBulletinData
with a partition key named table_name
(string type). You can do this using the AWS Management Console, AWS CLI, or Terraform.You can then create the Lambda function using Terraform, specifying the Python code as the function source code, and configuring the necessary IAM permissions for the Lambda function to access DynamoDB.
Note: This code assumes that you have configured your AWS credentials correctly, either by setting environment variables or using a shared credentials file. Additionally, make sure to handle any potential errors or exceptions that may occur during the scraping process.
There is a change needed here, it should use the full heading I supplied as there are other sections starting withFINAL ACTION DATES
andDATES FOR FILING
. I do like how Q extracted'/visa-bulletin-for-'
for the individual bulletin links.
Can you please show me the Terraform code to create the dynamodb table with the partition key mentioned above?
Response:
Sure, here’s an example of how you can create the DynamoDB table using Terraform: [1]
In this Terraform code:
resource "aws_dynamodb_table" "visa_bulletin_data" { ... }
defines a new DynamoDB table resource.name = "VisaBulletinData"
sets the name of the DynamoDB table.billing_mode = "PAY_PER_REQUEST"
specifies that the table should use the pay-per-request billing mode, where you pay for the read and write throughput consumed by your application.hash_key = "table_name"
defines the partition key (hash key) for the table astable_name
.attribute { ... }
block defines the attribute for the partition key, specifying its name (table_name
) and type (S
for String).
After defining this resource in your Terraform configuration, you can run
terraform apply
to create the DynamoDB table with the specified settings.Make sure to include the required AWS provider configuration in your Terraform code, specifying the AWS region and your AWS credentials (either through environment variables or a shared credentials file).
Once the DynamoDB table is created, you can use it in your Lambda function to store the scraped data as shown in the previous example.
Note: If you need to make any changes to the DynamoDB table later, such as adding a sort key or configuring additional settings, you can modify the Terraform code and run
terraform apply
again to update the table.Sources:
Since we already defined the AWS provider for Terraform, we can discard that part.
Thanks, can you now show me how I would deploy this Python code to Lambda using Terraform?
Response:
Sure, here’s an example of how you can deploy the Python code to AWS Lambda using Terraform: [1]
Here’s what this Terraform code does:
1. Define the Lambda function: The
aws_lambda_function
resource defines the Lambda function. It specifies the filename of the ZIP archive containing the Python code (lambda_function.zip
), the function name, the IAM role for the function, the handler function (lambda_function.lambda_handler
), and the Python runtime version (python3.9
). The source_code_hash
attribute is used to trigger updates when the source code changes. The environment
block sets the DYNAMODB_TABLE
environment variable with the name of the DynamoDB table.2. Define the IAM role: The
aws_iam_role
resource defines the IAM role that the Lambda function will assume. The assume_role_policy
specifies that the role can be assumed by the Lambda service.3. Attach IAM policies: The
aws_iam_policy_attachment
resources attach the necessary IAM policies to the Lambda role. The AWSLambdaBasicExecutionRole
policy provides basic permissions for Lambda functions, and the dynamodb_access_policy
(defined below) grants access to the DynamoDB table.4. Define the DynamoDB access policy: The
aws_iam_policy
resource defines an IAM policy that grants the PutItem
permission on the DynamoDB table. This policy is attached to the Lambda role in the previous step.Before applying this Terraform configuration, make sure to:
- Create a ZIP archive (
lambda_function.zip
) containing your Python code (lambda_function.py
). - Update the
filename
attribute in theaws_lambda_function
resource with the correct path to the ZIP archive. - Replace the
runtime
attribute with the appropriate Python version if you’re using a different version. - Configure the AWS provider with your AWS credentials and region.
After applying the Terraform configuration, Terraform will create the Lambda function, IAM role, and IAM policies. The Lambda function will have the necessary permissions to write data to the DynamoDB table.
Note: If you need to update the Lambda function code, you’ll need to create a new ZIP archive and update the
source_code_hash
attribute in the Terraform configuration. Then, run terraform apply
again to update the Lambda function.Sources:
This is close, but not quite there, I don’t want to add a manual step to zip up the Python app. I’m also assuming I need to run a command to install the dependencies, or store them somewhere. I also notice that the IAM permission is only for writing to the table, not reading, but that is fine for now, we haven’t gotten to the part where we need to access the data. Let’s follow-up with another question on how to avoid the manual zipping of the file.
Is there a way to handle the zipping of the file and installing any dependencies with Terraform?
Response:
Yes, Terraform provides a way to handle the zipping of the Lambda function code and installing dependencies without requiring manual steps. You can use the
archive_file
data source and the null_resource
resource with local-exec provisioners to achieve this. [1]Here’s an example of how you can modify the Terraform code to handle the zipping and dependencies: [2]
Here’s what the new code does:
- The
data "archive_file" "lambda_zip"
block creates a ZIP archive of the Lambda function code located in thelambda_code/
directory. - The
resource "null_resource" "lambda_package"
block is used to install dependencies and create the final Lambda package. Thetriggers
block ensures that this resource is re-created whenever the source code changes.- The
local-exec
provisioner runs a series of commands:- Create a Python virtual environment (
venv
). - Activate the virtual environment and install the dependencies listed in the
requirements.txt
file into thepackage/python
directory. - Deactivate the virtual environment.
- Change to the
package/python
directory and create a new ZIP archive containing the installed dependencies and the Lambda function code. - Remove the temporary
package
directory.
- The
aws_lambda_function
resource is updated to use thefilename
andsource_code_hash
values from thearchive_file
data source. Thedepends_on
attribute ensures that thenull_resource
is executed before creating or updating the Lambda function.
With this setup, Terraform will automatically handle the zipping of the Lambda function code and the installation of dependencies whenever you run
terraform apply
. The final ZIP archive will be created in the same directory as your Terraform configuration.Make sure to replace
${path.module}/lambda_code/
with the actual path to your Lambda function code directory, and ${path.module}/lambda_code/requirements.txt
with the path to your Python requirements file (if you have one).Note: The
null_resource
with local-exec
provisioners should be used as a last resort solution, as it can introduce potential issues with remote execution environments like Terraform Cloud. If possible, consider using a more robust packaging solution like AWS Lambda Layers or Docker containers for managing dependencies.Sources:
The mention of${path.module}
makes me think this code is from a module, so I will need to update it. Looking at the steps, I don’t think this will work. Theresource "null_resource" "lambda_package"
block uses thedata.archive_file.lambda_zip.output_base64sha256
as a trigger to run the commands inprovisioner "local-exec"
, which makes sense as it is a way to figure out if it should trigger a new build based on if anything has changed. Further down it doesn’t use thezip
created with thislocal-exec
though, just the original with just the source code, so will need to update this.
How would I add the dependencies for my python app to requirements.txt if I only have the .py file with imports at the top?
Response:
If you only have the Python file (e.g.,
lambda_function.py
) with the imports at the top, you can generate the requirements.txt
file automatically using the pipreqs
tool. Here are the steps: [1]- Install
pipreqs
using pip:pip install pipreqs - Navigate to the directory containing your Python file (
lambda_function.py
). - Run the
pipreqs
command with the path to your Python file:pipreqs /path/to/lambda_function.py
This will generate arequirements.txt
file in the same directory, containing the list of dependencies and their versions based on the imports in your Python file. [2]Alternatively, you can use the following command to generate therequirements.txt
file in a specific directory:pipreqs /path/to/lambda_function.py --force --savepath /path/to/requirements/directory
This command will create (or overwrite) therequirements.txt
file in the specified/path/to/requirements/directory
. - Review the generated
requirements.txt
file and make any necessary adjustments or additions. [3] - In your Terraform configuration, update the
local-exec
provisioner in thenull_resource
block to use the correct path to therequirements.txt
file:
- Replace
/path/to/requirements/directory/requirements.txt
with the actual path to yourrequirements.txt
file.
After making these changes, when you run
terraform apply
, Terraform will use the requirements.txt
file to install the necessary dependencies for your Python application.Note that the
pipreqs
tool analyzes the import statements in your Python file to generate the requirements.txt
file. It may not always be 100% accurate, especially for complex projects with nested dependencies or non-standard import patterns. In such cases, you may need to manually review and update the requirements.txt
file.Sources:
How would I call the lambda_handler function in my code while doing local development?
Response:
To call the
lambda_handler
function in your code during local development, you can create a separate Python script that imports and invokes the lambda_handler
function with the appropriate event and context objects. [1]Here’s an example of how you can do this:
- Create a new Python file, e.g.,
local_test.py
, in the same directory as yourlambda_function.py
file. - In
local_test.py
, import thelambda_handler
function from yourlambda_function.py
file:from lambda_function import
lambda_handler - Define a mock event object and a mock context object. The event object should mimic the structure of the event that your Lambda function expects to receive when invoked. The context object can be a simple dictionary or a custom class that implements the required methods and attributes.Here’s an example of a mock event object:
And here’s an example of a mock context object:class MockContext:
4. In
print(result)
5. Run the
local_test.py
, call the lambda_handler
function with the mock event and context objects:result =
lambda_handler(mock_event, mock_context)print(result)
5. Run the
local_test.py
script:python local_test.pyThis will execute the
lambda_handler
function with the mock event and context objects, and print the result returned by the function.You can modify the mock event and context objects to match the expected input for your Lambda function, and add any additional logic or assertions in
local_test.py
to verify the behavior of your lambda_handler
function.By separating the local testing code from the actual Lambda function code, you can easily run and debug your Lambda function locally without the need for deploying it to AWS or invoking it through AWS Lambda. [2]
Note: When running locally, you may need to mock or simulate any external dependencies or services that your Lambda function interacts with, such as DynamoDB, S3, or other AWS services. You can use libraries like
moto
or placebo
to mock AWS services during local development and testing.Sources:
For this prompt, I had unfortunately closed my VSCode instance, and had to restart the conversation.
For the html in src/sample.html, can you show me how I would extract the table values using Python, BeautifulSoup, and store each cell in DynamoDB? For the DynamoDB table, please show me the terraform code to create it. The html in src/sample.html is a local copy of a file, please assume that the URL will be passed as a parameter to the function that extracts the data.
Response:
To extract the table values from the HTML file using Python and BeautifulSoup, and store each cell in DynamoDB, you can follow these steps:
- Parse the HTML file using BeautifulSoup
2. Store the data in DynamoDB using Boto3 [1]
This code assumes that you have the necessary AWS credentials configured in your environment. It creates a DynamoDB table with a composite primary key consisting of
row_index
(hash key) and col_index
(range key). The extract_table_data
function retrieves the HTML content from the provided URL, parses the table, and returns a list of lists containing the table data. The store_data_in_dynamodb
function iterates over the table data and stores each cell value in DynamoDB using the put_item
method. [2]- Terraform code to create the DynamoDB table [3]
This Terraform code creates a DynamoDB table named
table_data
with a composite primary key consisting of row_index
(hash key) and col_index
(range key). The billing_mode
is set to PROVISIONED
, and the read_capacity
and write_capacity
are both set to 5. You can adjust these values based on your requirements.Note: Make sure to replace
os.environ.get('URL')
with the actual URL or a method to obtain the URL dynamically. Additionally, ensure that you have the necessary AWS credentials configured in your environment or use an AWS credentials provider for Boto3.Sources:
Given that the URLs I’m processing are all in the following format: https://travel.state.gov/content/travel/en/legal/visa-law0/visa-bulletin/2024/visa-bulletin-for-july-2024.html How can I extract the date for which it is based on the last part of the URL “-july-2024.html” and add it to the employment_based_data data I’m storing as a field called “Date” which should be a date-only object as the first day of that month?
Response:
To extract the date from the URL and add it as a field called “Date” in the
employment_based_data
list, you can modify the scrape_visa_bulletin
function as follows:Here’s what has been added or modified:
- Imported the
re
module for regular expression operations and thedatetime
module from the Python standard library. - Added a regular expression pattern
date_pattern
to match the date portion of the URL (e.g., “july-2024” in “visa-bulletin-for-july-2024.html”). - Used
re.search
to find the match in the URL, and extracted the month name and year from the match groups. - Created a
datetime
objectdate_obj
using the extracted month name and year, setting the day to the first of the month. - Added the
date_obj
to the dictionary in theemployment_based_data
list as the value for the “Date” key.
With these changes, the
employment_based_data
list will contain dictionaries with the following keys: “group_name”, “country”, “type”, “Value”, and “Date”. The “Date” value will be a datetime
object representing the first day of the month extracted from the URL.Note that this assumes the URL follows the format “visa-bulletin-for-{month}-{year}.html”, where {month} is a three-letter abbreviation of the month name (e.g., “jan”, “feb”, “mar”, etc.), and {year} is a four-digit year. If the URL format is different, you may need to modify the regular expression pattern accordingly.
The code blocks are added here only for readability, the prompt contained it as pasted in-line code. Given that I have the following collection built up using:
Can you show me how I would transform this collection into a data shape grouped in the following structure?
Response:
Sure, I can help you transform the collection into the desired data structure. Here’s an example of how you can achieve this in Python:
Here’s how the code works:
- We start by importing the
defaultdict
from thecollections
module, which will help us group the data efficiently. [1] - We create an empty list
transformed_data
to store the transformed data. - We use a nested
defaultdict
to group the data byfiling_type
andcategory
. The outerdefaultdict
groups byfiling_type
, and the innerdefaultdict
groups bycategory
. For each item inemployment_based_data
, we append a dictionary containing thecountry
,bulletin_date
, anddate
to the appropriate group. - Next, we iterate over the grouped data (
grouped_data
). For eachfiling_type
andcategory
combination, we create anotherdefaultdict
to group the data bycountry
. - For each
country
group, we create a list of dictionaries containing thebulletin_date
anddate
for that country. - Finally, we append a dictionary to
transformed_data
with thefiling_type
,category
, and a list of dictionaries containing thecountry
and itshistory
(the list ofbulletin_date
anddate
dictionaries).
After running this code, the
transformed_data
list will contain the data in the desired structure.Note: This code assumes that the
employment_based_data
collection contains unique combinations of filing_type
, country
, category
, and bulletin_date
. If there are duplicate entries, you may need to modify the code to handle them appropriately (e.g., by keeping only the latest entry or aggregating the data in some way).Sources:
Thanks, when I use this code, I can see that “grouped_data” has data in it, but the “transformed_data” object is returned as a “None”, can you help me troubleshoot this to fix it?
Response:
Sure, I can help you troubleshoot this issue. The problem might be that the
print(transformed_data)
statement is not being executed because of an error or exception occurring earlier in the code.To troubleshoot this, we can add some print statements and exception handling to the code to see where the issue is occurring. Here’s the modified code with some debugging statements:
In this modified code, we’ve added try-except blocks to catch any exceptions that might occur during the grouping and transformation steps. We’ve also added print statements to display the grouped data and the transformed data.
When you run this code, it will print the grouped data first. If there are no issues with the grouping step, it will then attempt to transform the data. If an exception occurs during the transformation step, it will print the error message and continue executing the code.
Finally, it will print the transformed data. If the
transformed_data
list is empty or None
, it will print an empty list or None
.By running this modified code, you should be able to identify where the issue is occurring and potentially fix it based on the error message or the printed data.
If you still can’t identify the issue, please provide the output of running this modified code, along with a sample of the
employment_based_data
collection, so that I can further assist you in troubleshooting the problem.Again, codeblock is just for readability. Thanks, that helped me find the issue. I now want to relook how the data will be stored in DynamoDB. I currently have the table defined using Terraform as:
Can you show me how I should change this so I can do a look up of the data using "filing_type", "category", and by a specific "country" in "countries"? Please only show the change the the DynamoDB table's Terraform code, the snippet for storing the whole data set, and the snippet for retrieving a subset of the data based on the 3 lookups I defined.
Response:
Sure, I can help you with that. To enable lookups based on
filing_type
, category
, and country
, we need to modify the DynamoDB table schema and use a composite primary key. Here's how you can change the Terraform code for the DynamoDB table:In this updated Terraform code:
- The
hash_key
is set to"pk"
(partition key), and therange_key
is set to"sk"
(sort key). Both keys are of type"S"
(String). - A
global_secondary_index
named"CountryIndex"
is defined, which has the samehash_key
andrange_key
as the table's primary key. Theprojection_type
is set to"ALL"
, which means that all attributes will be projected into the index. [1]
With this schema, you can store the data in DynamoDB using a composite primary key that combines the
filing_type
, category
, and country
values. Here's an example of how you can store the data:In this code snippet, we construct the
pk
(partition key) by combining the filing_type
and category
with a delimiter (#
). The sk
(sort key) is constructed by combining the country
and bulletin_date
(converted to an ISO-formatted string) with a delimiter.To retrieve a subset of the data based on
filing_type
, category
, and country
, you can use the following code:In this code snippet:
- We construct the
pk
(partition key) by combining thefiling_type
andcategory
with a delimiter (#
). - We construct the prefix for the
sk
(sort key) by combining thecountry
with a delimiter (#
). - We use the
query
method of the DynamoDB table to retrieve items where thepk
matches the constructed value, and thesk
begins with the constructed prefix. - The retrieved items are stored in the
items
variable.
This approach allows you to efficiently retrieve data based on the
filing_type
, category
, and country
values. You can modify the query conditions as needed to retrieve different subsets of the data.Note: Make sure to replace the
read_capacity
and write_capacity
values in the Terraform code with appropriate values based on your application's read and write requirements. Additionally, consider enabling auto-scaling for the DynamoDB table to automatically adjust the provisioned capacity based on your application's traffic.Sources:
The code below is causing an error, I see that "Key" is highlighted in my IDE with the warning: "Key is not defined" Here is the code causing the issue: response = table.query( KeyConditionExpression=Key('pk').eq(pk) & Key('sk').begins_with(sk_prefix) ) And the error when I run it: NameError: name 'Key' is not defined
Response:
The issue here is that the
Key
function is part of the boto3.dynamodb.conditions
module, and you need to import it explicitly in your Python script.To fix this error, add the following import statement at the top of your Python file:
from boto3.dynamodb.conditions import Key
After adding this import statement, the
Key
function will be available, and you can use it in your code without encountering the NameError
.With this change, your code should run without the
NameError: name 'Key' is not defined
error.The
Key
function is part of the boto3.dynamodb.conditions
module, which provides a way to construct condition expressions for DynamoDB queries and scans. It allows you to create conditions based on the primary key attributes (partition key and sort key) and other attributes of the DynamoDB table.By importing the
Key
function from the boto3.dynamodb.conditions
module, you can use it to construct the KeyConditionExpression
for your DynamoDB query, specifying conditions on the pk
(partition key) and sk
(sort key) attributes.Can you help me update the following code to handle multiple items with the same "country" field? The "bulletin_date" should be unique per "country". Here is the code causing the issue:
Response:
To handle multiple items with the same "country" field and ensure that the "bulletin_date" is unique per "country", we need to modify the grouping logic slightly. Here's the updated code:
Here's what has changed:
- We're using a nested
defaultdict
with three levels:filing_type
,category
, andcountry
. This allows us to group the data by all three keys. - In the grouping step, we're appending the
bulletin_date
anddate
dictionaries to the innermostdefaultdict
for each unique combination offiling_type
,category
, andcountry
. - In the transformation step, we iterate over the
countries
dictionary for eachfiling_type
andcategory
combination. For eachcountry
, we create a dictionary with thecountry
key and thehistory
data (the list ofbulletin_date
anddate
dictionaries). - We append these dictionaries to a
countries_list
, which is then added to thetransformed_data
list.
With this updated code, the "bulletin_date" will be unique per "country" because we're grouping the data by all three keys (
filing_type
, category
, and country
). The history
list for each country
will contain only unique bulletin_date
entries.Note: This code assumes that the
employment_based_data
collection does not contain duplicate entries for the same combination of filing_type
, country
, category
, and bulletin_date
. If there are duplicate entries, you may need to modify the code to handle them appropriately (e.g., by keeping only the latest entry or aggregating the data in some way).I am still getting the same error, can you show me the code to add to detect and print duplicates that would cause this issue?
Response:
Sure, I can help you with that. Here's the updated code that includes a check for duplicate entries based on the combination of
filing_type
, country
, category
, and bulletin_date
:Here's what has been added or modified:
- A
duplicates_found
flag is initialized toFalse
. - Before appending the
bulletin_date
anddate
dictionaries to the innermostdefaultdict
, we create a tuplekey
with the combination offiling_type
,country
,category
, andbulletin_date
. - We check if this
key
already exists in the innermostdefaultdict
for the correspondingfiling_type
,category
, andcountry
. If it does, it means we have a duplicate entry, and we print a message indicating the duplicate entry. - If the
key
doesn't exist, we append thebulletin_date
anddate
dictionaries to the innermostdefaultdict
, and we also append thekey
tuple to the same list. This will help us identify and remove thekey
tuple later. - After the grouping step, we check the
duplicates_found
flag. If it'sTrue
, we print a message indicating that duplicates were found, and the user should review the data. - If no duplicates were found, we proceed with the transformation step. However, before appending the
history
data to thecountries_list
, we remove thekey
tuple from thehistory_data
list using thepop()
method.
With these changes, the code will now detect and print any duplicate entries based on the combination of
filing_type
, country
, category
, and bulletin_date
. If duplicates are found, it will print a message and skip the transformation step. If no duplicates are found, it will proceed with the transformation and remove the key
tuples from the history
data before appending it to the transformed_data
list.Note: This code assumes that you want to keep the first occurrence of a unique entry and discard any subsequent duplicates. If you have a different requirement for handling duplicates (e.g., keeping the latest entry or aggregating the data), you'll need to modify the code accordingly.
I have a list called "visa_bulletin_links" which contains a list of strings. I then loop over the list to process the URLs in that list, can you show me how I would store the URL in DynamoDB after I process it successfully so that on the next run, I skip that URL? If another DynamoDB table is needed, please provide the Terraform code to create it.
Response:
Sure, I can help you with that. To store the processed URLs in DynamoDB and skip them on subsequent runs, you can create a separate DynamoDB table to keep track of the processed URLs. Here's how you can do it:
- Create a new DynamoDB table using Terraform: [1]Create a new file called
processed_urls_table.tf
and add the following Terraform code to create a DynamoDB table for storing the processed URLs:
- This Terraform code creates a DynamoDB table named
ProcessedURLs
with a partition key (hash key) namedurl
of type string (S
). You can adjust theread_capacity
andwrite_capacity
values based on your application's requirements. - Check if the URL has been processed before:Before processing a URL, you can check if it has already been processed by querying the
ProcessedURLs
table. Here's an example Python code snippet:
- sdf
In this code:- We create a DynamoDB resource and get a reference to the
ProcessedURLs
table. - For each URL in the
visa_bulletin_links
list, we use theget_item
method to check if the URL already exists in theProcessedURLs
table. - If the URL exists (i.e.,
'Item'
is in the response), we print a message indicating that the URL has already been processed and skip to the next URL usingcontinue
. - If the URL hasn't been processed, we print a message indicating that we're processing the URL, and then execute your code to process the URL.
- After successfully processing the URL, we store the URL in the
ProcessedURLs
table using theput_item
method.
By following this approach, you can keep track of the processed URLs in a separate DynamoDB table and skip them on subsequent runs of your application.
Note: Make sure to run
terraform apply
after creating the processed_urls_table.tf
file to create the ProcessedURLs
table in your AWS account. Also, remember to adjust the read_capacity
and write_capacity
values based on your application's requirements and enable auto-scaling if necessary.Sources:
Can you show me how to update the aws_iam_policy in the terraform code to allow access to this new DynamoDB table, and also include the required permissions to read from it and the VisaBulletinData table?
Response:
Sure, I can help you with that. Here's how you can update the AWS IAM policy in Terraform to allow access to the new
ProcessedURLs
DynamoDB table and the existing VisaBulletinData
table:Assuming you have an existing IAM policy resource in your Terraform code, you can add the following statements to the policy document:
resource "aws_iam_policy" "your_policy_name" {
name = "YourPolicyName"
description = "Policy for your application"
policy = <<EOF
{
"Version": "2012-10-17",
"Statement": [
# Existing statements...
name = "YourPolicyName"
description = "Policy for your application"
policy = <<EOF
{
"Version": "2012-10-17",
"Statement": [
# Existing statements...
{
"Effect": "Allow",
"Action": [
"dynamodb:GetItem",
"dynamodb:PutItem"
],
"Resource": "${aws_dynamodb_table.processed_urls.arn}"
},
{
"Effect": "Allow",
"Action": [
"dynamodb:GetItem",
"dynamodb:Query"
],
"Resource": "${aws_dynamodb_table.visa_bulletin_data.arn}"
}
]
}
EOF
}
"Effect": "Allow",
"Action": [
"dynamodb:GetItem",
"dynamodb:PutItem"
],
"Resource": "${aws_dynamodb_table.processed_urls.arn}"
},
{
"Effect": "Allow",
"Action": [
"dynamodb:GetItem",
"dynamodb:Query"
],
"Resource": "${aws_dynamodb_table.visa_bulletin_data.arn}"
}
]
}
EOF
}
In this updated policy:
- The first new statement allows the
GetItem
andPutItem
actions on theProcessedURLs
DynamoDB table. This will allow your application to check if a URL has been processed and store processed URLs in the table. - The second new statement allows the
GetItem
andQuery
actions on theVisaBulletinData
DynamoDB table. This will allow your application to retrieve data from theVisaBulletinData
table.
Note that you'll need to replace
your_policy_name
with the actual name of your IAM policy resource, and visa_bulletin_data
with the actual resource name of your VisaBulletinData
DynamoDB table.If you don't have an existing IAM policy resource in your Terraform code, you can create a new one with the following code:
resource "aws_iam_policy" "your_policy_name" {
name = "YourPolicyName"
description = "Policy for your application"
policy = <<EOF
{
"Version": "2012-10-17",
"Statement": [
{
"Effect": "Allow",
"Action": [
"dynamodb:GetItem",
"dynamodb:PutItem"
],
"Resource": "${aws_dynamodb_table.processed_urls.arn}"
},
{
"Effect": "Allow",
"Action": [
"dynamodb:GetItem",
"dynamodb:Query"
],
"Resource": "${aws_dynamodb_table.visa_bulletin_data.arn}"
}
]
}
EOF
}
name = "YourPolicyName"
description = "Policy for your application"
policy = <<EOF
{
"Version": "2012-10-17",
"Statement": [
{
"Effect": "Allow",
"Action": [
"dynamodb:GetItem",
"dynamodb:PutItem"
],
"Resource": "${aws_dynamodb_table.processed_urls.arn}"
},
{
"Effect": "Allow",
"Action": [
"dynamodb:GetItem",
"dynamodb:Query"
],
"Resource": "${aws_dynamodb_table.visa_bulletin_data.arn}"
}
]
}
EOF
}
After adding or updating the IAM policy resource in your Terraform code, you'll need to run
terraform apply
to apply the changes and update the IAM policy in your AWS account.Remember to attach this IAM policy to the IAM role or user that your application is using to access the DynamoDB tables.
Given that I have the following code that populates my collection:
employment_based_data.append({
})
'filing_type'
: filing_type, 'country': countries[cell_id - 1
], 'category'
: category_value, 'bulletin_date': bulletin_date.strftime("%Y-%m-%d"
), 'date': cell_date.strftime("%Y-%m-%d"
)})
How would I extract all the entries where I provide the filling_type, category, and country, and then sort and print the matching results in descending order by bulletin_date?
Response:
To extract all the entries where you provide the
filing_type
, category
, and country
, and then sort and print the matching results in descending order by bulletin_date
, you can use the following Python code:from operator import
itemgetter# Assuming employment_based_data is your initial collection
filing_type = 'Final Action Date'
category = 'EB-3'
country = 'All Chargeability Areas Except Those Listed'
# Filter the data based on filing_type, category, and country
filtered_data = [entry for entry in
employment_based_data if entry['filing_type'
] == filing_type and entry['category'
] == category and entry['country'
] == country]# Sort the filtered data in descending order by bulletin_date
sorted_data = sorted(filtered_data, key=itemgetter('bulletin_date'), reverse=True
)# Print the sorted data
for entry in
sorted_data: print(f"Bulletin Date: {entry['bulletin_date']}, Date: {entry['date']}"
)Here's how the code works:
- We define the
filing_type
,category
, andcountry
values for which we want to extract the data. - We use a list comprehension to filter the
employment_based_data
collection based on thefiling_type
,category
, andcountry
values. The resultingfiltered_data
list contains only the entries that match the specified criteria. - We use the
sorted
function to sort thefiltered_data
list in descending order based on thebulletin_date
value. Thekey
parameter of thesorted
function is set toitemgetter('bulletin_date')
, which tells thesorted
function to use thebulletin_date
value as the sorting key. Thereverse=True
argument ensures that the sorting is done in descending order. - The sorted data is stored in the
sorted_data
list. - Finally, we iterate over the
sorted_data
list and print each entry'sbulletin_date
anddate
values.
After running this code, you should see the matching entries printed in descending order by
bulletin_date
.Note: This code assumes that the
bulletin_date
and date
values in the employment_based_data
collection are strings in the format "YYYY-MM-DD"
. If they are in a different format or data type, you may need to modify the code accordingly.Can you explain the difference between "learnt" and "learned" please?
Response:
"Learnt" and "learned" are both past participle forms of the verb "to learn," but they are used differently in different varieties of English.
In American English, "learned" is the preferred past participle form, while in British English, "learnt" is more commonly used.
Here are some examples:
American English:
- I have learned a lot from this experience.
- She had learned to play the piano when she was a child.
- The students learned their lesson after failing the test.
British English:
- I have learnt a lot from this experience.
- She had learnt to play the piano when she was a child.
- The students learnt their lesson after failing the test.
In American English, "learnt" is generally considered incorrect or non-standard, although it may be used in some regional dialects or informal speech. In British English, both "learned" and "learnt" are considered correct, but "learnt" is more common in everyday speech and writing.
It's worth noting that in both varieties of English, the present participle form is "learning," as in "I am learning to code" or "She is learning French."
In summary, while "learnt" and "learned" mean the same thing, "learned" is the preferred form in American English, and "learnt" is more commonly used in British English. [1]
Sources:
Any opinions in this post are those of the individual author and may not reflect the opinions of AWS.