
Easy Serverless RAG with Knowledge Base for Amazon Bedrock
Discover enhancing FMs with contextual data for Retrieval Augmented Generation (RAG) to provide more relevant, accurate, and customized responses.
Published Feb 7, 2024
Last Modified Feb 12, 2024
In this article, we will learn and experiment with Amazon Bedrock Knowledge Base and AWS Generative AI CDK Constructs to build fully managed serverless RAG solutions to securely connect foundation models (FMs) in Amazon Bedrock to our custom data for Retrieval Augmented Generation (RAG).
We will build a simple Resume AI that can respond with more relevant, context-specific, and accurate responses according to the resume data we provided.
We will use several AWS Services like Amazon Bedrock, AWS Lambda, and AWS CDK.
- Knowledge Bases for Amazon Bedrock provides a fully managed RAG experience and the easiest way to get started with RAG in Amazon Bedrock.
- AWS CDK to define our cloud infrastructure in AWS.
- AWS Lambda is our serverless function to generate responses from Amazon Bedrock to the user.
Retrieval-augmented generation (RAG) is a technique in artificial intelligence that combines information retrieval with text generation. It's used to improve the quality of large language models (LLMs) by allowing them to access and process information from external knowledge bases. This can make LLMs more accurate, up-to-date, and relevant to specific domains or tasks.
By combining information retrieval with text generation, RAG can:
- Improve the accuracy and factual consistency of LLM responses. LLMs are trained on massive amounts of text data, but this data may not always be accurate or up-to-date. By accessing external knowledge bases, RAG can ensure that LLMs are using the most current and reliable information to generate their responses.
- Make LLMs more relevant to specific domains or tasks. By focusing on retrieving information from specific knowledge bases, RAG can tailor LLMs to specific domains or tasks. This can be useful for applications such as question answering, summarization, and translation.
- Provide transparency and explainability. RAG can provide users with the sources of the information that was used to generate a response. This can help users to understand how the LLM arrived at its answer and to assess its credibility.
Pre-processing data
To enable effective retrieval from private data, a common practice is to first split the documents into manageable chunks for efficient retrieval. The chunks are then converted to embeddings and written to a vector index, while maintaining a mapping to the original document. These embeddings are used to determine semantic similarity between queries and text from the data sources. The following image illustrates pre-processing of data for the vector database.
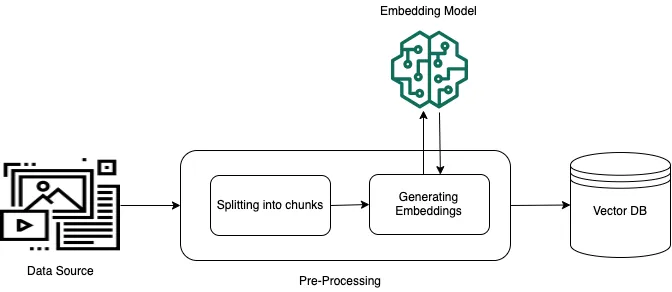
At runtime, an embedding model is used to convert the user's query to a vector. The vector index is then queried to find chunks that are semantically similar to the user's query by comparing document vectors to the user query vector. In the final step, the user prompt is augmented with the additional context from the chunks that are retrieved from the vector index. The prompt alongside the additional context is then sent to the model to generate a response for the user. The following image illustrates how RAG operates at runtime to augment responses to user queries.
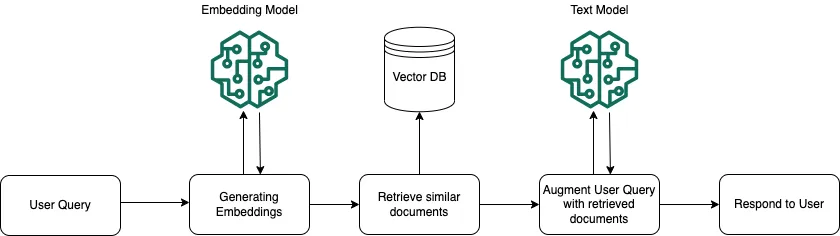
Amazon Bedrock is a fully managed service that offers a choice of high-performing foundation models (FMs) from leading AI companies like AI21 Labs, Anthropic, Cohere, Meta, Stability AI, and Amazon via a single API, along with a broad set of capabilities you need to build generative AI applications with security, privacy, and responsible AI.
Since Amazon Bedrock is serverless, you don't have to manage any infrastructure, and you can securely integrate and deploy generative AI capabilities into our applications using the AWS services you are already familiar with.
With a knowledge base, you can securely connect foundation models (FMs) in Amazon Bedrock to your company data for Retrieval Augmented Generation (RAG). Access to additional data helps the model generate more relevant, context-specific, and accurate responses without continuously retraining the FM.
Knowledge Bases gives you a fully managed RAG experience and the easiest way to get started with RAG in Amazon Bedrock.
Knowledge Bases for Amazon Bedrock manages the end-to-end RAG workflow for you. You specify the location of your data, select an embedding model to convert the data into vector embeddings, and have Amazon Bedrock create a vector store in your account to store the vector data. When you select this option (available only in the console), Amazon Bedrock creates a vector index in Amazon OpenSearch Serverless in your account, removing the need to manage anything yourself.
AWS Cloud Development Kit (CDK) is an open-source software development framework to define cloud infrastructure in code and provision it through AWS CloudFormation. The AWS CDK allows developers to model infrastructure using familiar programming languages, such as TypeScript, Python, Java, C#, and others, rather than using traditional YAML or JSON templates.
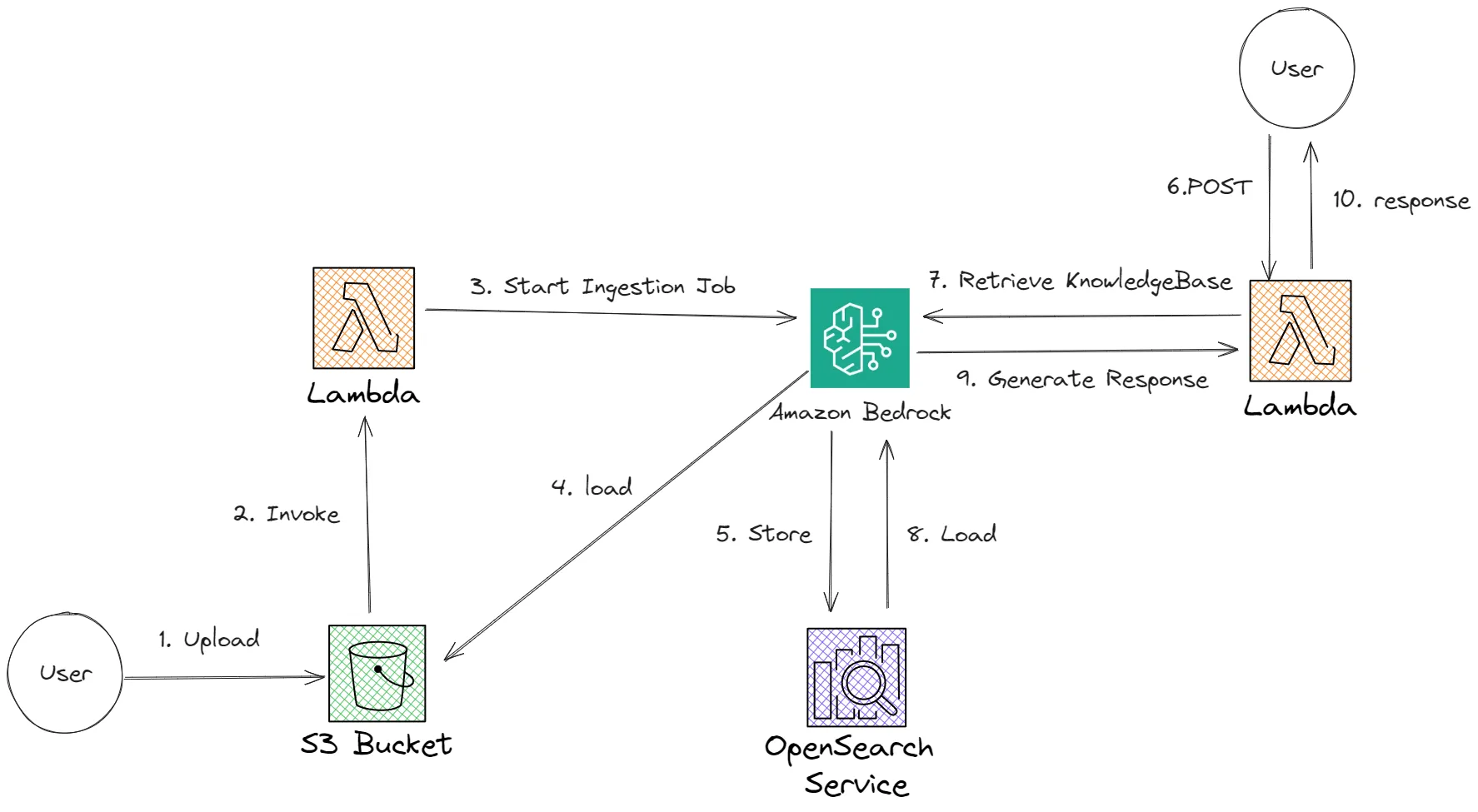
- We will start creating our project folder by running following command in our terminal.
- Then initialize our CDK project.
- First, prepare our resume data in a text or PDF file and save it as
data/resume.txt
with example content like this.
- We will create a couple of lambda functions in
src
folder.
- Create our first lambda function in
src/queryKnowledgeBase
- This function will handle user request queries and return the RAG response from Bedrock model.
- Type the following function code inside
src/queryKnowledgeBase/index.js
- Then create our second lambda function in
src/IngestJob
- This function will run Ingest Job in Bedrock Knowledge Base to do pre-processing and will get triggered when we upload our data to S3 Bucket.
- Type the following function code inside
src/IngestJob/index.js
.
- Then to start building our service with CDK, go to
lib/resume-ai-stack.js
file and type the following code.
- Then we can start deploying our services with CDK.
- After deployment is complete, CDK will return several output values including
KnowledgeBaseId
,QueryFunctionUrl
andResumeBucketName
.
- Based on the output above, we will sync all files in our
data
folder to S3 bucket nameknowledgebasecdkstack-resumebucket4dbbd0e5-qcstjyegctqe
.
- To start sending and receiving responses we can build our front end by following this script.
Our front end will look like this.
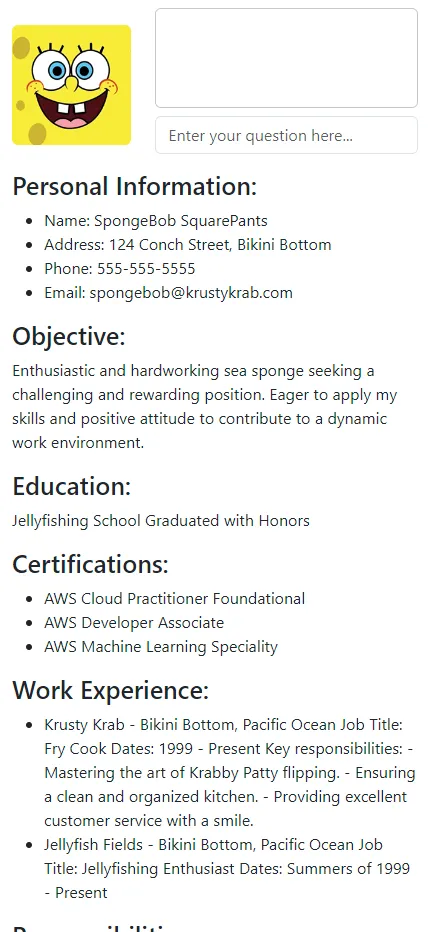
- Then we can start testing our RAG response for example by asking questions like
Tell me about yourself
,What certifications do you own?
or etc in the chat box. - It will return a response like this.
Finally, if we are done experimenting we can delete all our services by typing this command.
In this article, we learn how we can easily build our RAG solutions on AWS with Amazon Bedrock Knowledge Base and we also look into how we can utilize experimental AWS Generative AI CDK Constructs to build our generative AI services.
One thing to take note of is this CDK construct currently only supports Amazon OpenSearch Serverless. By default, this resource will create an OpenSearch Serverless vector collection and index for each Knowledge Base you create, with a minimum of 4 OCUs. But you can provide an existing collection and/or index to have more control.
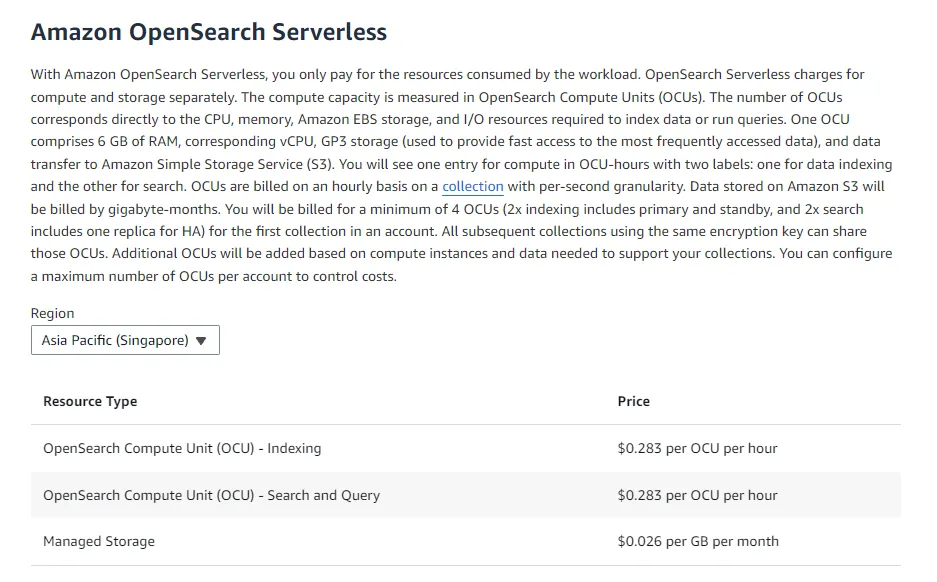