How to use Retrieval Augmented Generation (RAG) for Go applications
Implement RAG (using LangChain and PostgreSQL) to improve the accuracy and relevance of LLM outputs
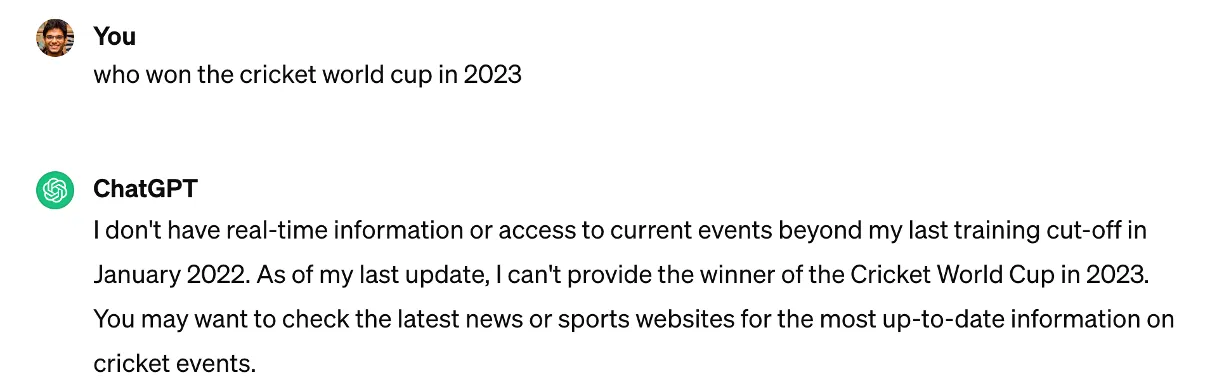
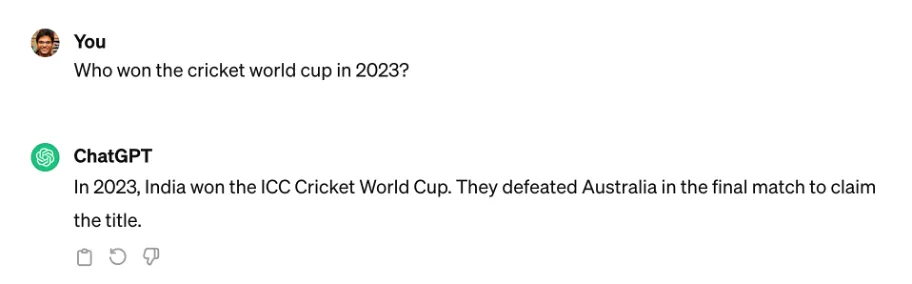
- Task-Specific tuning: Fine-tuning large language models on specific tasks or datasets to improve their performance on those domains.
- Prompt Engineering: Carefully designing input prompts to guide language models towards desired outputs, without requiring significant architectural changes.
- Few-Shot and Zero-Shot Learning: Techniques that enable language models to adapt to new tasks with limited or no additional training data.
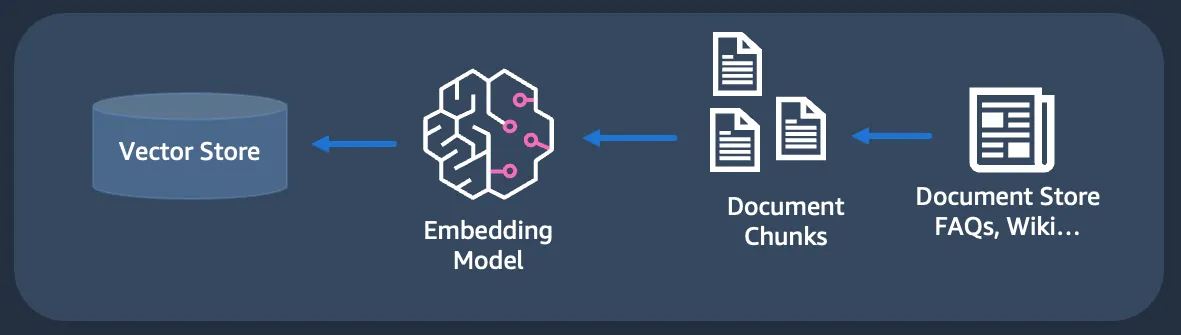
Thanks to generative AI technologies, there has also been an explosion in Vector Databases. These include established SQL and NoSQL databases that you may already be using in other parts of your architecture - such as PostgreSQL, Redis, MongoDB and OpenSearch. But there also database that are custom-built for vector storage. Some of these include Pinecone, Milvus, Weaviate,, etc.
- Retrieving data from a variety of external sources like documents, images, web URLs, databases, proprietary data sources, etc. This consists of sub-steps such as chunking which involves splitting up large datasets (e.g. a 100 MB PDF file) into smaller parts (for indexing).
- Create embeddings - This involves using an embedding model to convert data into their numerical representations.
- Store/Index embeddings in a vector store
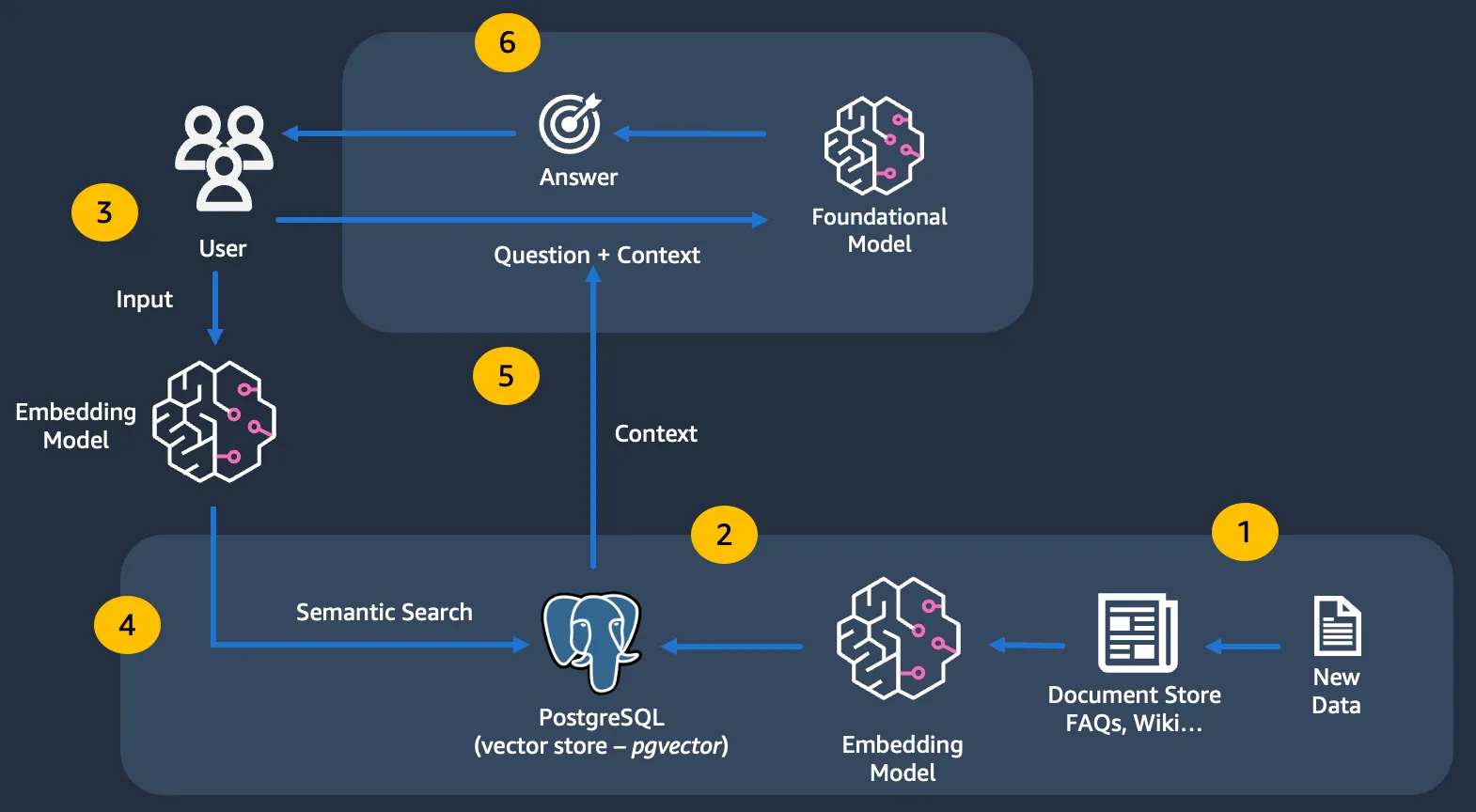
Ultimately, this is integration as part of a larger application where the contextual data (semantic search result) is provided to LLMs (along with the prompts).
- PostgreSQL - It will be uses as a Vector Database, thanks to the pgvector extension. To keep things simple, we will run it in Docker.
- langchaingo - It is a Go port of the langchain framework. It provides plugins for various components, including vector store. We will use it for loading data from web URL and index it in PostgreSQL.
- Text and embedding models - We will use Amazon Bedrock Claude and Titan models (for text and embedding respectively) with
langchaingo
. - Retrieval and app integration -
langchaingo
vector store (for semantic search) and chain (for RAG).
- Amazon Bedrock access configured from your local machine - Refer to this blog post for details.
1
docker run --name pgvector --rm -it -p 5432:5432 -e POSTGRES_USER=postgres -e POSTGRES_PASSWORD=postgres ankane/pgvector
pgvector
extension by logging into PostgreSQL (using psql
) from a different terminal:1
2
3
4
# enter postgres when prompted for password
psql -h localhost -U postgres -W
CREATE EXTENSION IF NOT EXISTS vector;
1
2
git clone https://github.com/build-on-aws/rag-golang-postgresql-langchain
cd rag-golang-postgresql-langchain
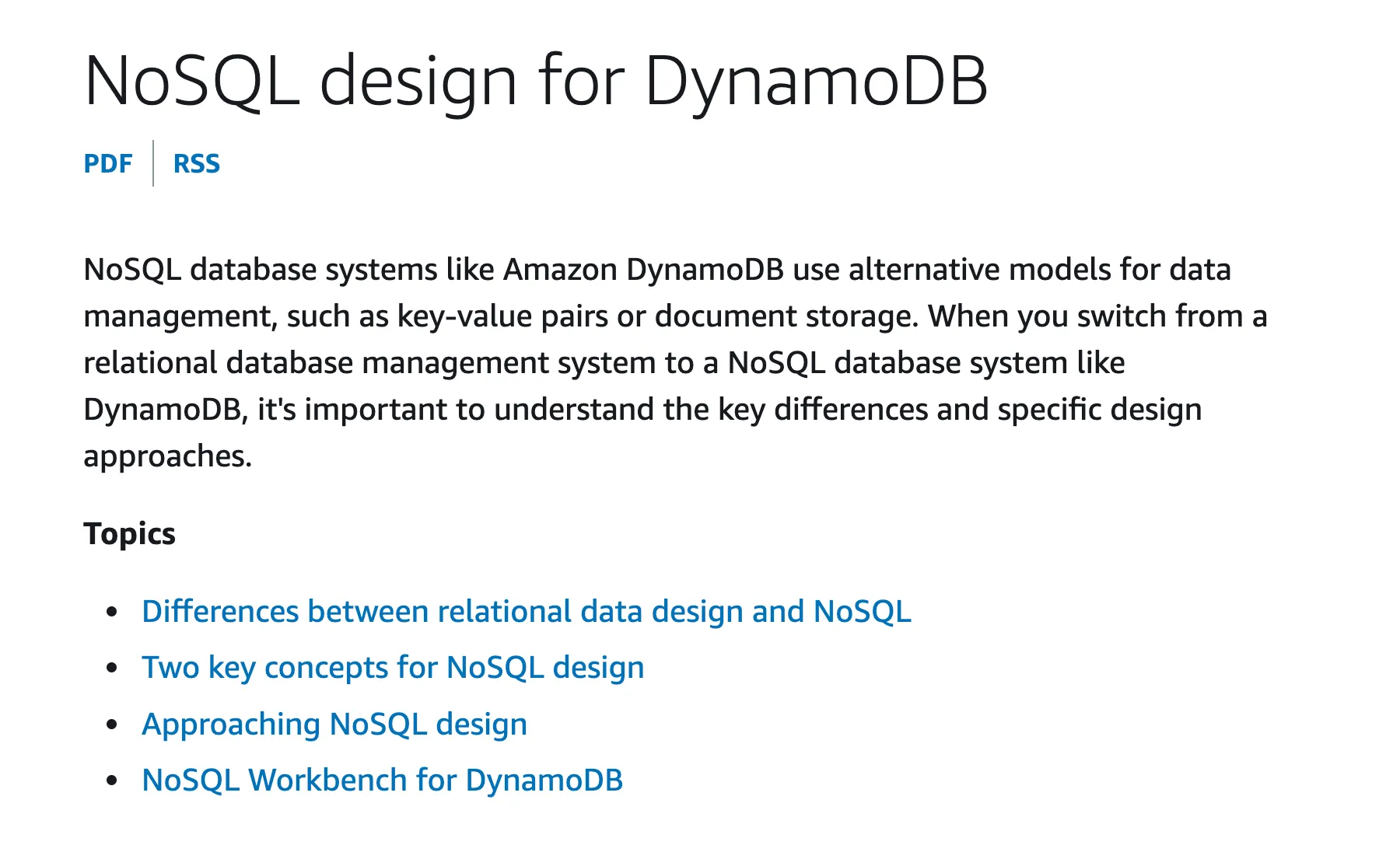
1
2
3
4
5
6
export PG_HOST=localhost
export PG_USER=postgres
export PG_PASSWORD=postgres
export PG_DB=postgres
go run *.go -action=load -source=https://docs.aws.amazon.com/amazondynamodb/latest/developerguide/bp-general-nosql-design.html
1
2
3
loading data from https://docs.aws.amazon.com/amazondynamodb/latest/developerguide/bp-general-nosql-design.html
vector store ready - postgres://postgres:postgres@localhost:5432/postgres?sslmode=disable
no. of documents to be loaded 23
1
data successfully loaded into vector store
psql
terminal and check the tables:1
\d
langchain_pg_collection
and langchain_pg_embedding
. These are created by langchaingo
since we did not specify them explicitly (that's ok, it's convenient for getting started!). langchain_pg_collection
contains the collection name while langchain_pg_embedding
stores the actual embeddings.1
2
3
4
| Schema | Name | Type | Owner |
|--------|-------------------------|-------|----------|
| public | langchain_pg_collection | table | postgres |
| public | langchain_pg_embedding | table | postgres |
1
2
3
4
select * from langchain_pg_collection;
select count(*) from langchain_pg_embedding;
select collection_id, document, uuid from langchain_pg_embedding LIMIT 1;
langchain_pg_embedding
table, since that was the number of langchain
documents that our web page source was split into (refer to the application logs above when you loaded the data)1
2
3
4
5
6
7
8
9
brc := bedrockruntime.NewFromConfig(cfg)
embeddingModel, err := bedrock.NewBedrock(bedrock.WithClient(brc), bedrock.WithModel(bedrock.ModelTitanEmbedG1))
//...
store, err = pgvector.New(
context.Background(),
pgvector.WithConnectionURL(pgConnURL),
pgvector.WithEmbedder(embeddingModel),
)
pgvector.WithConnectionURL
is where the connection information for PostgreSQL instance is providedpgvector.WithEmbedder
is the interesting part, since this is where we can plug in the embedding model of our choice.langchaingo
supports Amazon Bedrock embeddings. In this case I have used Amazon Bedrock Titan embedding model.
schema.Document
(getDocs
function) using the langchaingo
in-built HTML loader for this.1
docs, err := documentloaders.NewHTML(resp.Body).LoadAndSplit(context.Background(), textsplitter.NewRecursiveCharacter())
langchaingo
vector store abstraction and use the high level function AddDocuments
:1
_, err = store.AddDocuments(context.Background(), docs)
1
2
3
4
5
6
export PG_HOST=localhost
export PG_USER=postgres
export PG_PASSWORD=postgres
export PG_DB=postgres
go run *.go -action=semantic_search -query="what tools can I use to design dynamodb data models?" -maxResults=3
1
2
3
4
5
6
7
8
9
10
11
12
13
14
15
16
17
18
19
20
21
22
23
vector store ready
============== similarity search results ==============
similarity search info - can build new data models from, or design models based on, existing data models that satisfy
your application's data access patterns. You can also import and export the designed data
model at the end of the process. For more information, see Building data models with NoSQL Workbench
similarity search score - 0.3141409
============================
similarity search info - NoSQL Workbench for DynamoDB is a cross-platform, client-side GUI
application that you can use for modern database development and operations. It's available
for Windows, macOS, and Linux. NoSQL Workbench is a visual development tool that provides
data modeling, data visualization, sample data generation, and query development features to
help you design, create, query, and manage DynamoDB tables. With NoSQL Workbench for DynamoDB, you
similarity search score - 0.3186116
============================
similarity search info - key-value pairs or document storage. When you switch from a relational database management
system to a NoSQL database system like DynamoDB, it's important to understand the key differences
and specific design approaches.TopicsDifferences between relational data
design and NoSQLTwo key concepts for NoSQL designApproaching NoSQL designNoSQL Workbench for DynamoDB
Differences between relational data
design and NoSQL
similarity search score - 0.3275382
============================
-maxResults=3
).langchaingo
, we were able to easily ingest our source data into PostgreSQL and use the SimilaritySearch
function to get the top N results corresponding to our query (see semanticSearch
function in query.go):1
searchResults, err := store.SimilaritySearch(context.Background(), searchQuery, maxResults)
- Loaded vector data
- Executed semantic search
action
(rag_search
):1
2
3
4
5
6
export PG_HOST=localhost
export PG_USER=postgres
export PG_PASSWORD=postgres
export PG_DB=postgres
go run *.go -action=rag_search -query="what tools can I use to design dynamodb data models?" -maxResults=3
1
2
3
4
5
6
7
8
9
Based on the context provided, the NoSQL Workbench for DynamoDB is a tool that can be used to design DynamoDB data models. Some key points about NoSQL Workbench for DynamoDB:
- It is a cross-platform GUI application available for Windows, macOS, and Linux.
- It provides data modeling capabilities to help design and create DynamoDB tables.
- It allows you to build new data models or design models based on existing data models.
- It provides features like data visualization, sample data generation, and query development to manage DynamoDB tables.
- It helps in understanding the key differences and design approaches when moving from a relational database to a NoSQL database like DynamoDB.
So in summary, NoSQL Workbench for DynamoDB seems to be a useful tool specifically designed for modeling and working with DynamoDB data models.
langchaingo
vector store implementation. For this, we use a langchaingo
chain which takes care of the following:- Invokes semantic search
- Combines the semantic search along with a prompt
- Sends it to a Large Language Model (LLM), which in this case happens to be Claude on Amazon Bedrock.
ragSearch
in query.go):1
2
3
4
5
6
7
8
9
result, err := chains.Run(
context.Background(),
chains.NewRetrievalQAFromLLM(
llm,
vectorstores.ToRetriever(store, numOfResults),
),
question,
chains.WithMaxTokens(2048),
)
maxResults
to 10
, which means that the top 10
results from the vector database will be used to formulate the answer.1
go run *.go -action=rag_search -query="how is NoSQL different from SQL?" -maxResults=10
1
2
3
4
5
6
7
8
9
10
11
12
13
14
15
16
17
18
19
Based on the provided context, there are a few key differences between NoSQL databases like DynamoDB and relational database management systems (RDBMS):
1. Data Modeling:
- In RDBMS, data modeling is focused on flexibility and normalization without worrying much about performance implications. Query optimization doesn't significantly affect schema design.
- In NoSQL, data modeling is driven by the specific queries and access patterns required by the application. The data schema is designed to optimize the most common and important queries for speed and scalability.
2. Data Organization:
- RDBMS organizes data into tables with rows and columns, allowing flexible querying.
- NoSQL databases like DynamoDB use key-value pairs or document storage, where data is organized in a way that matches the queried data shape, improving query performance.
3. Query Patterns:
- In RDBMS, data can be queried flexibly, but queries can be relatively expensive and don't scale well for high-traffic situations.
- In NoSQL, data can be queried efficiently in a limited number of ways defined by the data model, while other queries may be expensive and slow.
4. Data Distribution:
- NoSQL databases like DynamoDB distribute data across partitions to scale horizontally, and the data keys are designed to evenly distribute the traffic across partitions, avoiding hot spots.
- The concept of "locality of reference," keeping related data together, is crucial for improving performance and reducing costs in NoSQL databases.
In summary, NoSQL databases prioritize specific query patterns and scalability over flexible querying, and the data modeling is tailored to these requirements, in contrast with RDBMS where data modeling focuses on normalization and flexibility.
langchaingo
has support for lots of different model, including ones in Amazon Bedrock (e.g. Meta LLama 2, Cohere, etc.) - try tweaking the model and see if it makes a difference? Is the output better?- What about the Vector Database? I demonstrated PostgreSQL, but
langchaingo
supports others as well (including OpenSearch, Chroma, etc.) - Try swapping out the Vector store and see how/if the search results differ? - You probably get the gist, but you can also try out different embedding models. We used Amazon Titan, but
langchaingo
also supports many others, including Cohere embed models in Amazon Bedrock.
langchaingo
as the framework. but this doesn't always mean you have to use one. You could also remove the abstractions and call the LLM platforms APIs directly if you need granular control in your applications or the framework does not meet your requirements. Like most of generative AI, this area is rapidly evolving, and I am optimistic about having Go developers having more options build generative AI solutions.Any opinions in this post are those of the individual author and may not reflect the opinions of AWS.