How to use Retrieval Augmented Generation (RAG) for Go applications
Implement RAG (using LangChain and PostgreSQL) to improve the accuracy and relevance of LLM outputs
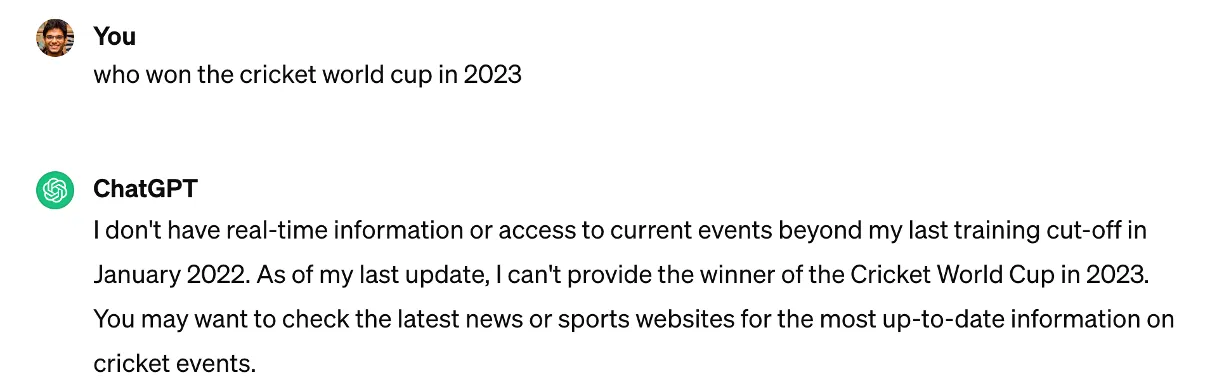
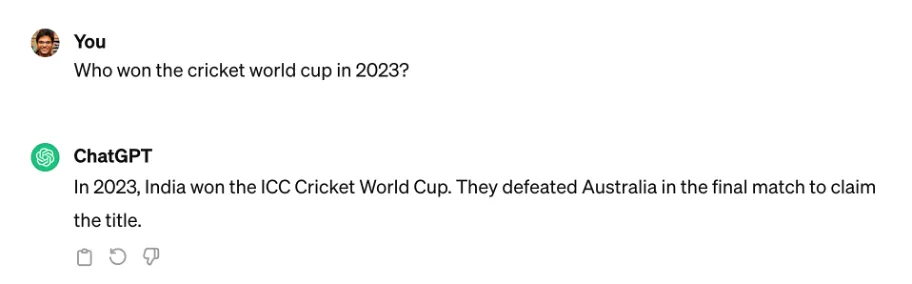
- Task-Specific tuning: Fine-tuning large language models on specific tasks or datasets to improve their performance on those domains.
- Prompt Engineering: Carefully designing input prompts to guide language models towards desired outputs, without requiring significant architectural changes.
- Few-Shot and Zero-Shot Learning: Techniques that enable language models to adapt to new tasks with limited or no additional training data.
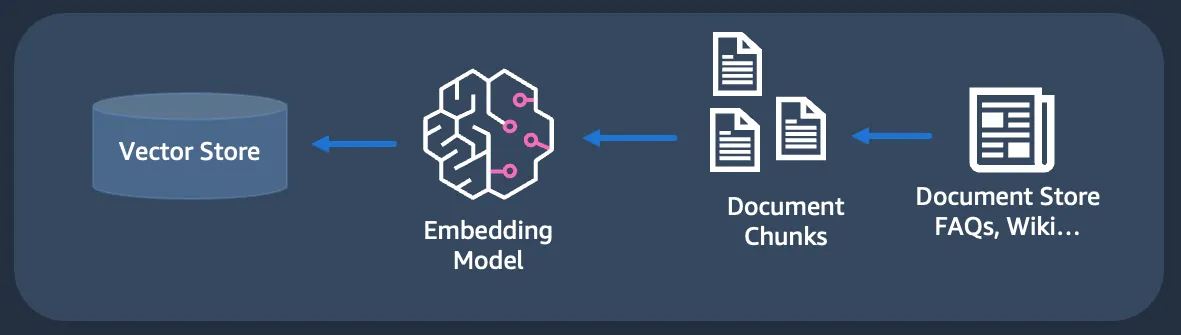
Thanks to generative AI technologies, there has also been an explosion in Vector Databases. These include established SQL and NoSQL databases that you may already be using in other parts of your architecture - such as PostgreSQL, Redis, MongoDB and OpenSearch. But there also database that are custom-built for vector storage. Some of these include Pinecone, Milvus, Weaviate,, etc.
- Retrieving data from a variety of external sources like documents, images, web URLs, databases, proprietary data sources, etc. This consists of sub-steps such as chunking which involves splitting up large datasets (e.g. a 100 MB PDF file) into smaller parts (for indexing).
- Create embeddings - This involves using an embedding model to convert data into their numerical representations.
- Store/Index embeddings in a vector store
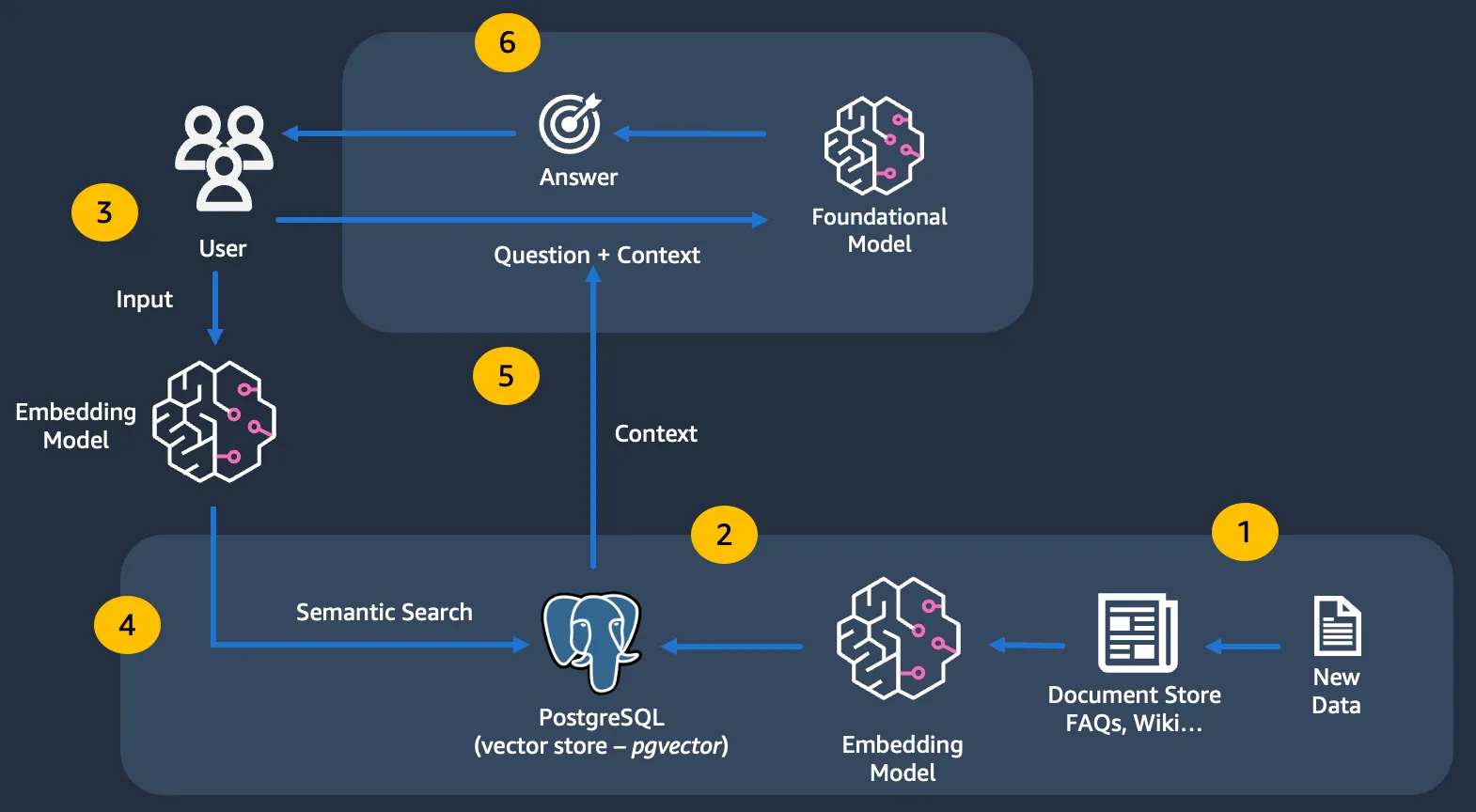
Ultimately, this is integration as part of a larger application where the contextual data (semantic search result) is provided to LLMs (along with the prompts).
- PostgreSQL - It will be uses as a Vector Database, thanks to the pgvector extension. To keep things simple, we will run it in Docker.
- langchaingo - It is a Go port of the langchain framework. It provides plugins for various components, including vector store. We will use it for loading data from web URL and index it in PostgreSQL.
- Text and embedding models - We will use Amazon Bedrock Claude and Titan models (for text and embedding respectively) with
langchaingo
. - Retrieval and app integration -
langchaingo
vector store (for semantic search) and chain (for RAG).
- Amazon Bedrock access configured from your local machine - Refer to this blog post for details.
pgvector
extension by logging into PostgreSQL (using psql
) from a different terminal: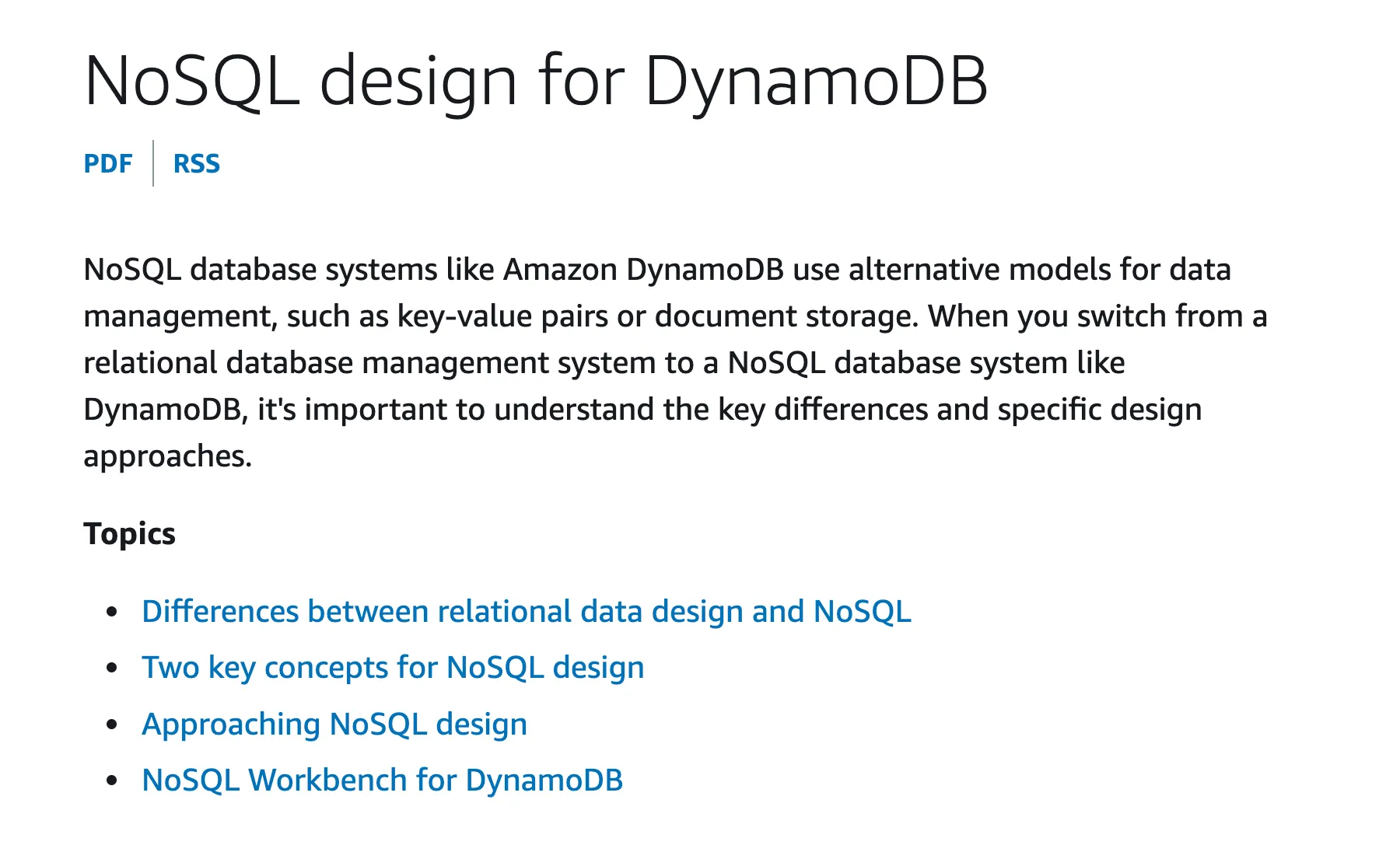
psql
terminal and check the tables:langchain_pg_collection
and langchain_pg_embedding
. These are created by langchaingo
since we did not specify them explicitly (that's ok, it's convenient for getting started!). langchain_pg_collection
contains the collection name while langchain_pg_embedding
stores the actual embeddings.langchain_pg_embedding
table, since that was the number of langchain
documents that our web page source was split into (refer to the application logs above when you loaded the data)pgvector.WithConnectionURL
is where the connection information for PostgreSQL instance is providedpgvector.WithEmbedder
is the interesting part, since this is where we can plug in the embedding model of our choice.langchaingo
supports Amazon Bedrock embeddings. In this case I have used Amazon Bedrock Titan embedding model.
schema.Document
(getDocs
function) using the langchaingo
in-built HTML loader for this.langchaingo
vector store abstraction and use the high level function AddDocuments
:-maxResults=3
).langchaingo
, we were able to easily ingest our source data into PostgreSQL and use the SimilaritySearch
function to get the top N results corresponding to our query (see semanticSearch
function in query.go):- Loaded vector data
- Executed semantic search
action
(rag_search
):langchaingo
vector store implementation. For this, we use a langchaingo
chain which takes care of the following:- Invokes semantic search
- Combines the semantic search along with a prompt
- Sends it to a Large Language Model (LLM), which in this case happens to be Claude on Amazon Bedrock.
ragSearch
in query.go):maxResults
to 10
, which means that the top 10
results from the vector database will be used to formulate the answer.langchaingo
has support for lots of different model, including ones in Amazon Bedrock (e.g. Meta LLama 2, Cohere, etc.) - try tweaking the model and see if it makes a difference? Is the output better?- What about the Vector Database? I demonstrated PostgreSQL, but
langchaingo
supports others as well (including OpenSearch, Chroma, etc.) - Try swapping out the Vector store and see how/if the search results differ? - You probably get the gist, but you can also try out different embedding models. We used Amazon Titan, but
langchaingo
also supports many others, including Cohere embed models in Amazon Bedrock.
langchaingo
as the framework. but this doesn't always mean you have to use one. You could also remove the abstractions and call the LLM platforms APIs directly if you need granular control in your applications or the framework does not meet your requirements. Like most of generative AI, this area is rapidly evolving, and I am optimistic about having Go developers having more options build generative AI solutions.Any opinions in this post are those of the individual author and may not reflect the opinions of AWS.